Introduction to GPT and Language Capabilities
Fundamentals of Generative Pre-training Transformer
The Generative Pre-training Transformer (GPT), a product ofOpenAI, is a large language model that leverages deep learning for text generation. It’s a pre-trained model that can be adjusted for many different natural language processing (NLP) tasks such as translation, classification, and generation of text.
The model, based on a transformer architecture with self-attention mechanisms, is proficient at handling sequential inputs. This feature equips GPT with a high capacity to interpret context and meaning in longer text sequences.
Unsupervised learning approaches are employed during model training, which relies on large volumes of text data. The models learn based on input text patterns and structures without the need for labelled data. The latest iteration, GPT-3, was trained on a colossal 45-terabyte text dataset, making it one of the most extensive language models available.
Multilingual Functionality in GPT
GPT models can process text in a variety of languages, making them particularly useful for applications requiring multilingual functionality. GPT-3, for instance, supports more than 40 languages, including popular ones like English, Spanish, French, German, Chinese, and Japanese.
Multilingual functionality in GPT is realized through a technique known as zero-shot learning. With this, the model can generate text in languages it hasn’t been explicitly trained on by using a language-agnostic representation of language.
Specific languages or language pairs can be used to fine-tune GPT models, which is particularly beneficial for machine translation tasks. For example, you could fine-tune GPT on an English-French translation dataset to create a model capable of accurate translation between those languages.
Overall, the transformer architecture and multilingual functionality make GPT a potent asset for an array of natural language processing applications.
Investigating the Linguistic Diversity of OpenAI’s Free Chat GPT
OpenAI’s Free Chat Gpt in Spanish is an AI chatbot that has seen a surge in popularity since its introduction. It can generate human-like responses to text inputs in a range of languages, which is one of its most notable features.
Comparative Study of GPT in Varied Languages
Free Chat GPT has been trained on massive quantities of text data in various languages, allowing it to generate responses in those languages. However, the tool’s performance does fluctuate between languages. A comparative study shows that the tool performs better for some languages than others.
The tool excels in English response generation, courtesy of the large volume of English text data available. In contrast, for low-resource languages such as Swahili and Hausa, the performance of Free Chat GPT is subpar due to the scarcity of text data in these languages.
Obstacles and Solutions in Supporting Linguistic Diversity
Supporting a variety of languages in Free Chat GPT comes with its unique set of difficulties. The availability of text data in various languages is one significant challenge. Low-resource languages like Swahili and Hausa have limited text data, which poses a challenge when training Free Chat GPT models in these languages.
The structural and grammatical differences between languages present another challenge in developing a universal model that can generate responses in all languages. To overcome this, researchers have created language-specific models trained on text data in individual languages.
In conclusion, Free ChatGpt in Japanese is a powerful tool for communication across different languages. However, the support for a variety of languages comes with its own challenges, which need creative solutions. Continuous efforts are being made by researchers to enhance the performance of Free Chat GPT in various languages and to tackle the issues associated with linguistic diversity.
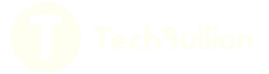