In today’s technological era, Namboodiri Arun Mullamangalath Kesavan, an innovator in network architecture, has developed groundbreaking insights into the integration of Artificial Intelligence (AI) within Secure Access Service Edge (SASE) frameworks. This synthesis heralds a transformative era in network security, delivering unprecedented capabilities in threat detection, compliance, and performance optimization.
Real-Time Threat Detection: A Game-Changer
Integrating AI into SASE frameworks transforms threat detection and response by leveraging machine learning to analyze network telemetry in real time, establish behavioral baselines, and detect anomalies. This enables proactive identification of zero-day vulnerabilities and emerging threats. Predictive analytics reduces mean time to detect threats by 90%, enhancing response times, mitigating risks, and addressing evolving cybersecurity challenges effectively.
Dynamic Network Optimization: Intelligent Resource Management
AI-enhanced SASE frameworks optimize network performance dynamically by monitoring metrics like latency, jitter, and throughput. Using reinforcement learning, they intelligently reroute traffic based on real-time conditions, improving performance for diverse applications. This results in a 45% reduction in latency and a 60% increase in throughput compared to traditional networks. Additionally, AI predicts user movement and preconfigures resources for seamless connectivity across devices and locations, offering adaptability crucial for cloud-native environments with dynamic workloads and demanding network management needs.
Automation in Compliance Management
Compliance management poses challenges in navigating complex regulations. AI-powered SASE frameworks simplify this by automating adherence to standards like GDPR, HIPAA, and PCI-DSS. Machine learning maps security controls to compliance frameworks, ensuring continuous alignment. Natural language processing (NLP) interprets regulations to implement policies effectively. Automated auditing systems monitor compliance in real time, flag violations, and generate detailed reports. These advancements have reduced violations by 80% and cut manual auditing by 65%, enabling organizations to focus on strategic priorities.
Privacy-Preserving Security: Encrypted Traffic Analysis
The increasing use of encrypted traffic poses a dual challenge for organizations: maintaining robust security while preserving data privacy. AI-enhanced SASE frameworks address this challenge through encrypted traffic analysis, which balances privacy and security. Using metadata analysis and differential privacy techniques, these systems detect threats without accessing the actual payloads of encrypted data. This approach ensures compliance with privacy regulations while maintaining high detection accuracy. For instance, AI systems can analyze packet timing, size, and flow characteristics to identify malicious activities within encrypted traffic, achieving a detection rate exceeding 95% for known attack patterns. This capability is particularly valuable in industries such as healthcare and finance, where sensitive data handling is critical.
Operational Efficiency through AI-Driven Management
AI’s impact on operational efficiency within SASE frameworks is transformative. Infrastructure as Code (IaC) principles enable automated deployment, while machine learning algorithms analyze network requirements to generate optimal configurations. These systems incorporate rollback capabilities and validation checks, ensuring smooth implementation with minimal disruption. Real-time monitoring further enhances efficiency by providing comprehensive visibility into network operations. Predictive analytics identify potential issues before they affect service quality, enabling proactive resolution. Organizations adopting AI-driven SASE have reported a 70% reduction in incident resolution time and a 40% decrease in operational expenses. The automation of manual tasks, such as configuration management, has freed up IT resources to focus on strategic improvements.
Future-Proofing Security Architectures
As enterprises continue to adopt cloud-native applications and remote work models, the demands on network security architectures grow exponentially. AI-enhanced SASE frameworks offer a scalable, future-proof solution to these challenges. By combining real-time adaptability with automated precision, these frameworks set a new benchmark for enterprise security. The convergence of AI and SASE not only addresses current challenges but also prepares organizations for the future. With capabilities such as predictive threat detection, dynamic optimization, and privacy-preserving analysis, AI-driven SASE frameworks are well-positioned to tackle emerging security threats and regulatory complexities.
In conclusion, the integration of AI with SASE marks a pivotal advancement in network security, driven by Namboodiri Arun Mullamangalath Kesavan’s innovative contributions. AI empowers enterprises with enhanced threat detection, network optimization, and compliance management, ensuring robust and adaptive security solutions. This transformative approach improves operational efficiency and privacy compliance, enabling organizations to navigate digital transformation confidently. As AI-powered SASE frameworks become integral to modern enterprises, this vision drives ongoing innovation, shaping a secure and scalable future for network security.
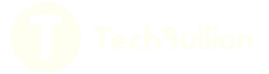