Artificial Intelligence (AI) continues to drive technological evolution, with recent advancements in deep learning and related fields opening up new possibilities for industries worldwide. In this article, we explore breakthroughs in reinforcement learning, generative models, and cybersecurity, providing a glimpse into how these innovations are reshaping the landscape. Chirag Gajiwala, an AI expert, sheds light on these groundbreaking technologies, guiding us through their potential applications and future directions.
Reinforcement Learning: A Step Towards Smarter Systems
Reinforcement Learning (RL) allows AI systems to learn by interacting with their environment and adjusting based on feedback. The integration of deep learning has led to Deep Reinforcement Learning (DRL), enabling AI to handle complex tasks like strategic gaming and navigating unpredictable situations. A notable success was AlphaGo’s victory over a Go world champion, showcasing DRL’s ability to master intricate, long-term decision-making. Beyond gaming, DRL is now applied in robotics and autonomous systems, where it improves machine learning from real-world environments, enhancing efficiency and adaptability in various industries.
Transfer Learning: Bridging the Gap Between Tasks
Transfer learning is a key advancement in reinforcement learning, enabling AI systems to transfer knowledge from one task to another related task, reducing training time and enhancing efficiency. This makes AI more adaptable in real-world applications like robotics and industrial automation. For example, an AI trained to handle one object can quickly adapt to manipulating different objects. Transfer learning has broad applications, from autonomous driving to healthcare, allowing AI to recognize patterns or anomalies across various contexts with minimal retraining.
Generative Models: Unleashing AI’s Creative Potential
While much of AI research has concentrated on analytical and decision-making tasks, generative models have expanded possibilities in creative applications. These models generate new data samples based on learned patterns, revolutionizing content creation across various industries. Two key types are Generative Adversarial Networks (GANs) and Variational Autoencoders (VAEs).
GANs excel in generating hyper-realistic images and videos, widely used in entertainment, fashion, and scientific research. They also create synthetic datasets for machine learning training, addressing data scarcity in many fields.
VAEs, in contrast, focus on learning efficient data representations for tasks such as dimensionality reduction and anomaly detection. Their applications are especially valuable in drug discovery, where they generate new molecular structures, significantly accelerating the development of innovative treatments.
Cybersecurity: A Growing Need for AI Integration
As cyber threats grow more complex, AI is becoming crucial in enhancing cybersecurity. Deep learning algorithms are now used to detect network anomalies, identify vulnerabilities in real-time, and prevent threats before they escalate. Graph Neural Networks (GNNs) are a promising development in this field, capable of analyzing graph-structured data, such as social networks, to detect hidden patterns indicating security breaches. Additionally, AI automates vulnerability assessments and risk prioritization, making it indispensable in today’s digital world. As attackers also use AI, the cybersecurity “arms race” will continue driving innovation in AI-powered defenses.
The Road Ahead: AI’s Evolving Capabilities
The future of AI is filled with exciting possibilities as emerging technologies continue to push its boundaries. One of the most promising developments is Graph Neural Networks (GNNs), which excel at processing complex, graph-structured data. This technology has far-reaching applications, from advancing drug discovery to improving recommendation systems by analyzing intricate data relationships.
Generative AI is another rapidly growing field, highlighting the creative potential of machines. As AI becomes more adept at generating high-quality content—across text, images, and video—industries like entertainment, media, and design are set for major transformations. AI-driven content creation will unlock new possibilities for innovation and efficiency in these fields.
Additionally, the rise of multimodal AI, which integrates diverse data types like text, image, audio, and video, enables more comprehensive and versatile applications. Coupled with edge computing, these advancements will enhance real-time decision-making in devices like autonomous vehicles and IoT systems, making AI more impactful across various sectors.
In conclusion, AI advancements in reinforcement learning, generative models, and cybersecurity are transforming industries, presenting both opportunities and challenges. As these technologies evolve, their applications will grow, from automating tasks to generating new digital content. Chirag Gajiwala highlights AI’s innovative progress, emphasizing that the future of AI is not only about smarter machines but also about reshaping creativity, security, and problem-solving.
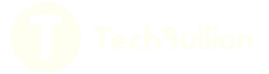