Technology is evolving at an unprecedented pace, but with this rapid expansion comes the growing concern of digital waste. Addressing this challenge is Durga Rao Manchikanti, whose research delves into mitigating digital waste in artificial intelligence (AI) and cloud computing. His work offers innovative solutions to balance technological progress with environmental responsibility.
Redefining Digital Waste in the Modern Era
Digital waste encompasses inefficient data storage, redundant computations, and underutilized network resources. As AI and cloud computing grow, so does their environmental footprint. The challenge lies in managing this waste and preventing it through smarter design and operational practices. Effective digital waste reduction can significantly impact global energy consumption and carbon emissions.
The Carbon Footprint of AI Training
Training AI models, especially deep learning algorithms, requires extensive computational power, often leading to excessive energy use. Many models are overprovisioned, consuming more resources than necessary. Innovative solutions, such as optimizing model architectures and implementing memory-efficient training techniques, can drastically reduce AI’s environmental impact.
Smart Data Management for Sustainable Computing
One of the primary contributors to digital waste is redundant data storage. Organizations often maintain multiple copies of datasets, consuming unnecessary storage and energy. By employing deduplication techniques and automated data classification, storage waste can be minimized. These strategies not only enhance efficiency but also reduce infrastructure demands.
Edge Computing: A Game Changer for Efficiency
Edge computing is emerging as a revolutionary approach to reducing reliance on centralized data centers. By processing data closer to the source, edge computing significantly reduces data transfer volumes, minimizes energy consumption, and improves real-time processing. This shift reduces network congestion and alleviates the environmental burden of large-scale cloud operations.
Optimizing AI Model Deployment
Beyond training, AI model deployment also contributes to digital waste. Inefficient inference processes and resource-heavy model serving infrastructures lead to unnecessary computational overhead. Implementing dynamic resource provisioning and interference-aware scheduling can enhance efficiency. These strategies ensure that AI applications operate with minimal waste while maintaining optimal performance.
Reducing Cloud Computing Inefficiencies
Data centers, which power cloud computing, consume vast amounts of electricity. Power Usage Effectiveness (PUE) has become a critical metric for assessing data center efficiency. Strategies such as dynamic workload allocation, intelligent resource monitoring, and virtualization have shown promise in optimizing cloud infrastructure. Despite increased computing demand, these improvements have helped stabilize global data center energy consumption.
Sustainable Infrastructure: The Role of Renewable Energy
Integrating renewable energy sources into cloud computing is a crucial step toward sustainability. Many data centers are now adopting solar, wind, and hydroelectric power to reduce their reliance on fossil fuels. Smart grid technologies and AI-driven energy management systems further optimize energy usage, ensuring a greener approach to computing.
Cooling Systems: A Key Factor in Energy Savings
Cooling inefficiencies account for a substantial portion of a data center’s energy use. Traditional cooling methods often consume excessive power, but liquid and free cooling solutions advancements have led to significant energy savings. By leveraging these innovations, data centers can improve their efficiency while reducing their environmental footprint.
The Future of Green AI and Cloud Computing
The future of sustainable AI and cloud computing hinges on continuous innovation. Green AI technologies, such as carbon-aware scheduling and intelligent workload distribution, will optimize energy consumption and reduce digital waste. The industry is also moving toward standardized sustainability metrics, fostering transparency and accountability in environmental impact. Advancements in energy-efficient hardware, renewable-powered data centers, and AI-driven resource management will further drive sustainability. Collaboration between tech leaders, policymakers, and researchers will be essential in building an eco-friendly digital ecosystem.
The Need for Industry-Wide Collaboration
Achieving long-term sustainability in computing requires a collective effort. Organizations must adopt best practices, from data lifecycle management to hardware efficiency measures. Regulatory frameworks and certification programs can provide guidelines for responsible digital operations. By fostering a culture of environmental consciousness, the tech industry can ensure that innovation does not come at the cost of sustainability.
In conclusion, Durga Rao Manchikanti’s research emphasizes reducing digital waste in AI and cloud computing. Smarter data management, optimized resource use, and renewable energy adoption can curb environmental impact, ensuring a future where computing remains powerful and sustainable.
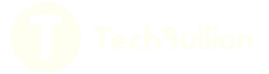