In an era where real-time data processing is becoming increasingly vital, the convergence of edge computing and cloud infrastructure is paving the way for transformative innovations in artificial intelligence (AI) applications. This article explores these innovations as discussed by Srinivas Chennupati, whose research sheds light on how edge-cloud architectures can significantly enhance the efficiency of AI systems.
A Groundbreaking Hybrid Approach
The core innovation presented is the hybrid approach that leverages the complementary strengths of edge and cloud computing. Edge computing, by processing data closer to its source, significantly reduces latency and minimizes bandwidth usage. The cloud, on the other hand, offers vast computational resources and scalability. This convergence creates an ideal environment for handling real-time AI applications, particularly in fields like autonomous vehicles, smart cities, and healthcare.
Recent statistics reveal the tangible benefits of this integration. For instance, hybrid architectures have reduced data processing latency by up to 73% and improved AI model accuracy by as much as 24% compared to traditional cloud-only solutions. Such dramatic improvements are particularly vital in industries like manufacturing, where operational efficiency is crucial.
The Research Methodology Behind the Findings
His research is grounded in comprehensive quantitative analysis, leveraging both theoretical frameworks and real-world case studies. The study examines various resource allocation strategies, computational offloading techniques, and the bandwidth requirements that influence edge-cloud systems. Through experimental data, the research demonstrates how edge devices can filter up to 85% of raw data, drastically reducing the amount sent to the cloud and, in turn, improving system efficiency.
In addition to the performance metrics, the research also considers machine learning-based algorithms that dynamically allocate resources. These strategies have been proven to enhance the overall efficiency of edge-cloud systems by up to 37%, offering a significant leap over traditional static methods.
Technical Innovations in AI Workload Distribution
At the heart of this innovation is workload distribution. Traditional centralized cloud systems struggle to keep up with the ever-growing demand for AI processing power. Edge-cloud synergy, however, uses a hierarchical approach, with edge devices handling time-sensitive tasks and offloading computationally intensive workloads to the cloud.
Advanced task partitioning strategies, as detailed in the research, have proven effective in optimizing response times and reducing energy consumption. Fine-grained task partitioning, in which applications are divided into smaller subtasks, can improve task completion times by 18-27% over coarser methods. This level of optimization is critical in applications like autonomous vehicles, where split-second decisions can make the difference between life and death.
Addressing the Challenges of Edge-Cloud Integration
Despite the clear advantages, there are still several technical challenges to overcome. Latency management remains one of the most critical obstacles, particularly for time-sensitive applications. The hybrid edge-cloud approach can reduce latency by up to 47% through dynamic task partitioning that adjusts based on real-time network and processing conditions.
A Glimpse into the Future of Edge-Cloud Synergy
Looking ahead, the future of edge-cloud synergy is promising. The integration of next-generation 5G networks will further enhance the potential of this hybrid architecture. With 5G’s ultra-low latency and high bandwidth, edge-cloud systems will be able to support even more complex and real-time AI applications, such as autonomous drones and advanced healthcare monitoring systems.
Overcoming Limitations and Moving Forward
While the integration of edge and cloud computing holds immense potential, challenges such as resource allocation optimization, privacy concerns, and network limitations must still be addressed. However, the ongoing research and development in edge-cloud architectures offer promising solutions that could help overcome these hurdles. As technologies like machine learning, 5G, and privacy-preserving techniques evolve, edge-cloud synergy will continue to redefine the capabilities of real-time AI applications.
In conclusion, Srinivas Chennupati’s research highlights the transformative potential of edge-cloud computing for AI applications. This synergy enables faster, more efficient, and secure processing of real-time data, enhancing performance across diverse industries. As technologies like 5G, advanced orchestration frameworks, and privacy-preserving techniques continue to evolve, the scope of edge-cloud integration will only expand. The future of AI-driven industries looks promising, driven by these innovations that empower systems to be smarter, faster, and more adaptable than ever before.
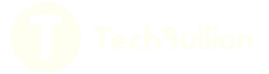