The retail industry is witnessing a paradigm shift, thanks to the transformative power of machine learning. A recent article by Akhilesh Kota sheds light on how machine learning is revolutionizing the dynamic pricing landscape, enabling retailers to make more informed and adaptive decisions. The insights delve into the nuances of this innovative approach, emphasizing its role in reshaping consumer experiences and business profitability.
Understanding Dynamic Pricing
Dynamic pricing is a strategy that adjusts prices in real-time, responding to fluctuations in demand, competition, and external factors. Unlike traditional static pricing, it uses advanced algorithms to analyze and react to market conditions with accuracy and speed. When combined with machine learning, dynamic pricing becomes even more powerful. Machine learning algorithms process vast amounts of data, identify patterns, and refine pricing strategies based on actionable insights. This enables businesses to stay competitive, meet customer expectations, and maximize revenue. By ensuring prices are neither excessively high nor unsustainably low, dynamic pricing balances profitability with customer satisfaction, driving sustainable business growth.
Machine Learning: The Game Changer
The application of machine learning in dynamic pricing brings unprecedented sophistication to pricing strategies, leveraging data-driven insights to enhance decision-making. Machine learning algorithms excel in several key areas:
Analyzing Market Trends: These systems detect subtle shifts in consumer preferences, competitor pricing, and market conditions, enabling proactive and informed pricing adjustments.
Predicting Demand: By forecasting demand patterns for specific products and timeframes, businesses can optimize inventory levels, avoid stockouts, and maximize revenue opportunities.
Enhancing Personalization: Tailored pricing strategies based on individual customer profiles, preferences, and purchase history create a more personalized and engaging shopping experience.
By automating complex analytical processes, machine learning not only reduces human error but also enables faster, more accurate responses to dynamic market conditions, empowering businesses to stay competitive.
Data as the Backbone
The success of machine learning in dynamic pricing hinges on the quality and diversity of data inputs. Retailers need to collect extensive information, such as historical sales trends, customer demographics, competitor pricing, and broader economic indicators. These datasets are essential for training algorithms that evolve and optimize pricing strategies over time. Furthermore, integrating real-time data allows these models to adapt swiftly to changing market conditions, ensuring prices remain competitive and aligned with current demand. This combination of comprehensive data and real-time updates empowers businesses to make informed, agile decisions, helping them gain an edge in today’s fast-paced and competitive markets.
Overcoming Challenges
The potential of machine learning in dynamic pricing is vast, but it comes with challenges that businesses must navigate carefully. Ethical concerns, such as unintentional price discrimination, can erode consumer trust if not addressed transparently. Moreover, the implementation of machine learning systems demands substantial investment in infrastructure, skilled professionals, and technical expertise. These hurdles can be daunting, particularly for smaller organizations. To overcome them, businesses should prioritize transparency in pricing strategies and ensure algorithms adhere to ethical standards. Investing in scalable, user-friendly technologies and fostering a workforce skilled in data science can simplify adoption, ensuring the benefits outweigh the challenges.
Future Prospects
The integration of machine learning into dynamic pricing represents a transformative shift toward a smarter and more adaptive retail landscape. As technology continues to advance, several key developments are expected to shape the future of pricing strategies:
Improved Algorithms: Enhanced models will delve deeper into consumer behavior, identifying subtle trends and preferences for precise price adjustments.
Seamless Integration: Dynamic pricing systems will increasingly connect with inventory management, marketing, and customer engagement tools, creating unified ecosystems.
Global Applications: Machine learning will enable region-specific pricing strategies, catering to the unique demands of diverse markets and cultural contexts.
These advancements will not only empower retailers with better decision-making tools but also foster customer satisfaction by ensuring transparent, fair, and competitive pricing tailored to individual needs.
In conclusion, Akhilesh Kota’s insights into machine learning-powered dynamic pricing reveal its immense potential to transform retail. Leveraging data and advanced algorithms enables businesses to enhance efficiency, boost profitability, and adapt to evolving market demands. Embracing these innovations will be essential for retailers aiming to thrive in a competitive and rapidly changing industry landscape.
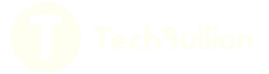