In recent years, healthcare has witnessed a shift from traditional decision-making systems to more dynamic, real-time solutions powered by artificial intelligence and advanced analytics. Satya Manesh Veerapaneni‘s research on integrating Business Intelligence (BI) with Clinical Decision Support Systems (CDSS) highlights how this technological evolution is reshaping healthcare. By transforming retrospective data analysis into proactive, real-time clinical interventions, these innovations offer solutions to some of the most pressing challenges in modern medicine.
A Leap Forward: Real-Time Analytics in Healthcare
Real-time analytics in healthcare has revolutionized patient care by eliminating the delays associated with retrospective Business Intelligence tools. In critical settings like emergency departments, this advancement provides immediate access to essential insights, significantly improving patient outcomes. Integrating real-time Clinical Decision Support Systems (CDSS) with Electronic Health Records (EHRs) allows healthcare providers to make informed decisions at the point of care. Studies have shown that real-time decision support reduces diagnostic errors by 20% and treatment delays by over 20 minutes, especially in time-sensitive conditions like strokes, sepsis, and acute coronary syndrome.
From Data to Decision: The Power of Predictive Analytics
Predictive analytics has revolutionized real-time clinical decision support by going beyond static thresholds and general guidelines. Modern Clinical Decision Support Systems (CDSS) analyze data from diverse sources, such as vital signs, lab results, and clinical notes, to make accurate real-time predictions. For instance, machine learning models for sepsis detection have shown sensitivity rates of up to 92%, enabling early intervention and improving patient outcomes.
These systems represent a shift toward personalized medicine, where treatments are tailored to an individual’s unique clinical profile rather than general categories. This approach not only enhances treatment effectiveness but also plays a key role in medication safety. Real-time systems that monitor patient data and adjust medication dosages automatically have reduced medication-related complications by more than 50%. This advancement highlights the potential of predictive analytics in improving patient care and preventing adverse drug events.
Tackling Key Challenges with Smart Technology
Smart technology has the potential to revolutionize healthcare, but key challenges must be addressed. One major issue is alert fatigue, where healthcare professionals receive numerous notifications, making it hard to prioritize critical alerts. To tackle this, organizations are adopting smarter systems that categorize alerts by urgency, reducing redundancy and cognitive overload. Early studies show that multi-tiered alert systems can improve response rates and minimize fatigue. Another challenge is data accuracy and integration, as machine learning models often struggle with real-world variations. Overcoming this requires continuous model refinement and seamless integration into clinical workflows to prevent disruptions.
The Road Ahead: Personalized and Precision Medicine
The future of clinical decision support lies in its ability to provide not just immediate but deeply personalized care. Looking ahead, the integration of genomic data into CDSS promises to bring precision medicine to the forefront of clinical practice. This integration will enable healthcare providers to make treatment decisions based on genetic markers, allowing for more accurate, individualized care. This is particularly important as the healthcare industry shifts from population-based medicine to approaches that consider individual variations in treatment response.
Furthermore, advancements in AI and machine learning will continue to enhance predictive models, moving beyond early detection to forecasting disease progression. This evolution will allow healthcare providers to intervene even earlier, potentially preventing diseases from advancing to critical stages. With the ability to continuously adapt and learn from new data, these systems will become more sophisticated, making healthcare more efficient and effective.
In conclusion, Satya Manesh Veerapaneni’s exploration into real-time clinical decision support underscores a future where healthcare systems not only react to illness but predict and prevent it. As these systems evolve, they will continue to transform the way healthcare is delivered, bridging the gap between data collection and patient care in ways previously thought impossible. However, successful implementation requires overcoming key challenges like alert fatigue, workflow integration, and data accuracy. By addressing these issues, healthcare organizations can realize the full potential of these technologies, ultimately leading to better patient outcomes and a more efficient healthcare system. As this field progresses, the future promises a more personalized, precise, and proactive approach to medicine.
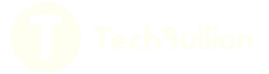