Introduction to Kodanda Rami Reddy Manukonda
Given the ever-increasing rates of innovation in software development, reliability and efficiency in the test automation frameworks should be a priority. All in all, it is understood how performance-sensitive APIs and project management affect large-scale enterprise projects. Due to his efforts, Kodanda Rami Reddy Manukonda has set a good foundation for this field. His unique frame works designs and integration of Agile-Waterfall techniques are evidence of his efforts that have elevated the standards of the industry’s practices and boosted the performance and operations standards.
Manukonda, a highly professional and well-experienced Test Architect, has discussed challenges of the project management and API testing in particular with clarity and accuracy. On the aspects of test automation and telecommunication, he has set new standards for the industry. The author is highly experienced and this can be felt through his ideas which range from the technical part of implementing Agile and the strategic part of implementing Waterfall.
His views on
Improving Quality and Efficiency Using Advanced Machine Learning Techniques in the Telecommunications Industry
The telecommunication industry especially within the internet and data service seeks to improve the standard of the service delivery while increasing operational productivity. The growth in data traffic volumes and customers’ expectations create pressure on telecom providers and force them to look at the ML approach more and more seriously using complex techniques. This article discusses how ML is disrupting the telecom sector leveraging real-life examples and occasional diagrams for enhancement of understanding.
Key Areas Where Machine Learning Drives Telecom Efficiency
Machine learning is applying its impact on telecom operations in several fields but with more emphasis on network management, customer service, and data. The following are the major areas through which ML is enhancing the efficacy and quality of services within the internet and data
- Network Traffic Management
Traffic management has therefore become greatly important in today’s world where networks dominate everything. It is identified that telecom providers are applying machine learning algorithms that can help identify congestion in the network in a more effective and real-time manner. Some of these algorithms are capable of predicting traffic congestion, helping providers allocate bandwidths, and ensuring Internet connection does not become interrupted or slow.
Case Study 1: Dynamic Network Traffic Management
One of the world’s largest telecommunication companies used machine learning to forecast usage patterns during peak hours by applying the analysis results. This was achieved by a 35% reduction in network congestion by the use of cars, which greatly improved customer satisfaction especially during congested days like on the weekends or holidays.
- Predictive Maintenance for Internet Infrastructure
Telecoms are primarily huge physical plants running both above and beneath the ground in the form of fiber-optic cables, routers, and data centers among others. Predictive maintenance is achieved through Machine Learning; this means that instead of the telecom provider concentrating on their infrastructure and waiting for something to go wrong, there is a way of predicting when the infrastructural system will require attention.
Diagram 1: Predictive Maintenance for Fiber-Optic Cables
The following diagram outlines the predictive maintenance process:
Case Study 2: Fiber-Optic Infrastructure Maintenance
In the U. S., a telecom firm used ML for managing and predicting maintenance needs for optical fibers in its telecommunication network. Various parameters that include, temperature, vibrations, and the strength of signals were also employed to give the ML algorithms a means of identifying potential failures. This meant that repair costs were cut by 25% and there was also less service disruption thus enhancing the reliability of the social network.
- Optimizing Data Transmission and Compression
Because of the high growth rates in data consumption, transmission, and detailed compression play a crucial role. Case in point, in data compression, powerful machine learning models are now used to ensure that the transfer speed is enhanced without having to reduce the quality of the transfer. This is desirable for video streaming, transfer of large files, and cloud-based applications.
Case Study 3: ML-Driven Data Compression for Video Streaming
A telecom provider operating in Europe received the task of sending high-quality video to millions of people and at the same time the load on a network should be as low as possible. It developed an ML model that adjusted the video compression ratio: Thus, it decreased data usage by a quarter while maintaining high video quality and preventing disruptions during ‘peaks’.
Diagram 2: ML-Driven Data Compression Process
This diagram shows how ML optimizes video streaming:
- Customer Experience and Personalization
It is also revolutionizing the way customers interact with it by the introduction of ML. Through understanding how customers are using the services, the kind of services they prefer, and the common service issues customers are likely to face, telecom providers are in a position to create more customized plans and also be in a position to address the service issues before customers raise their complaints.
Case Study 4: Personalized Data Plans and Customer Retention
An Asian telecom operator used Machine Learning to understand customers’ behavior in terms of usage and the kind of devices used. He made data packages flexible and sold new data packages to customers, which increased their satisfaction level while increasing the rate of their retention by 15% and thus increased the company’s overall revenue.
Diagram 3: ML in Customer Personalization
Below is a diagram showing the personalization process:
- Anomaly Detection in Data Networks
Telecom networks today are very complex; hence, there is a need to identify such issues as access violations or high traffic. If the network data is processed in an unsupervised manner, the ML models can constantly monitor the data stream and notice the signs of an attack in real-time.
Case Study 5: Anomaly Detection for Cybersecurity in Data Networks
A Latin American telecom company used an unsupervised ML to analyze internet traffic and identify differences. The system was able to detect traffic changes meaning that when the incidences increased, it was categorized as a DDoS attack. Such problems detected at an early stage helped the company to avoid a large-scale degradation of services.
Conclusion
The increasing use of higher-level machine learning algorithms in the telecommunications business sector, especially in the internet and data business segments has been responsible for enhanced network utilization, positive customer relations as well as dependable and efficient business operations. Based on the ongoing improvements to the ML models, the telecom providers that use these technologies will be ahead in developing better, faster, and dependable services for their customers to compete well in this progressive field successfully.
For More Details: https://www.linkedin.com/in/kodanda-rami-reddy-manukonda-9a2487148/
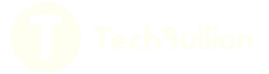