In a world where images speak louder than words, machine learning is paving the way for groundbreaking advancements in image recognition technology. From facial recognition to object detection, the possibilities are endless! Join us as we explore how machine learning is revolutionizing the way we see and understand visual data. Get ready to be amazed at what AI can do for our visual world!
Meaning of Machine Learning and Image Recognition
Machine learning is a subset of artificial intelligence that involves the development of algorithms and statistical models that enable computer systems to learn from data without being explicitly programmed. In recent years, machine learning has gained significant attention due to its ability to revolutionize various industries, including image recognition.
Image recognition, also known as computer vision, is the process of using computers and algorithms to identify and classify objects within digital images or videos. This technology has been around for decades but has seen rapid advancement in recent years with the incorporation of machine learning techniques.
The combination of machine learning and image recognition has opened up new possibilities in various fields such as healthcare, transportation, retail, manufacturing, and many others. With the increasing availability of large datasets and more powerful computing capabilities, this technology has become even more accurate and efficient.
One of the key advantages of using machine learning in image recognition is its ability to continuously improve performance through experience. Rather than relying on predefined rules or guidelines like traditional software programs, machine learning models can analyze vast amounts of data and adjust their algorithms accordingly to achieve better results over time.
There are two main types of machine learning techniques used in image recognition: supervised and unsupervised learning. In supervised learning, labeled datasets are provided during training sessions where the model learns from specific input-output pairs. On the other hand, unsupervised learning involves training on unlabeled data sets where patterns within the data are detected by algorithmically clustering them together.
Apart from these traditional methods, newer approaches such as deep learning have also emerged as a powerful tool in image recognition tasks. Deep learning involves training multi-layer neural networks with various layers designed to extract different features from images before making a prediction about what it contains accurately.
History of Image Recognition
The history of image recognition can be traced back to the early 1950s when researchers first started exploring ways to teach computers how to see and interpret images. One of the earliest breakthroughs in this field was made by neuroscientist David Marr, who developed a theoretical framework for understanding visual perception in humans. This inspired many computer scientists to use similar principles to develop algorithms for image recognition.
In the 1960s and 1970s, researchers began experimenting with pattern recognition techniques using handcrafted features such as edges, lines, and shapes. However, these early methods were limited in their ability to accurately identify objects in complex images.
It wasn’t until the introduction of machine learning techniques in the late 1980s that significant progress was made in image recognition. The emergence of artificial neural networks (ANNs) allowed computers to learn from data and improve their performance over time. ANNs are modeled after the structure and function of neurons in the human brain and have proven to be highly effective at recognizing patterns and features within images.
Fast forward to the mid-2000s, when deep learning emerged as a powerful subset of machine learning techniques that propelled image recognition even further. Deep learning involves training complex neural networks with multiple layers on large datasets, allowing them to automatically extract meaningful features from images without being explicitly programmed.
In recent years, deep learning has revolutionized image recognition by achieving unprecedented levels of accuracy on tasks such as object detection, classification, and segmentation. For example, Inception V3 – a deep convolutional neural network (CNN) developed by Google – achieved top scores on ImageNet Challenge’s classification task in 2015 with an error rate lower than that of human experts.
Aside from advancements in machine learning techniques themselves, another crucial factor contributing to the development of image recognition is the growing availability of massive datasets for training models. Companies like Facebook, Google, Microsoft have amassed enormous amounts of labeled data through their vast user bases, facilitating the training of more accurate and robust image recognition models.
How Machine Learning is Changing the Game
The field of artificial intelligence has rapidly evolved in recent years, with machine learning leading the charge. Machine learning, a subset of AI, involves training algorithms to learn and improve from data without being explicitly programmed. This technology has had a significant impact on various industries, including image recognition.
Gone are the days when humans were solely responsible for recognizing objects in images. Thanks to machine learning, computers can now analyze large datasets and accurately identify objects in images at lightning speed. This has revolutionized image recognition in various fields, such as healthcare, security, and e-commerce.
In healthcare, accurate and timely diagnosis is crucial for effective treatment. Traditional methods of medical imaging require a trained radiologist or technician to manually review each image, which can be time-consuming and prone to human error. With machine learning-based image recognition systems, this process is significantly improved. These systems can quickly analyze medical images such as X-rays or MRIs and flag any abnormalities for further review by a human expert.
In the security industry, surveillance cameras are a common tool used to monitor public spaces and prevent crime. However, monitoring these cameras manually is an impossible task due to their sheer volume. That’s where machine learning-powered image recognition comes into play. These systems can detect anomalous behavior or suspicious individuals in real-time using facial recognition technology, alerting authorities before any potential threats escalate.
E-commerce companies have also been heavily impacted by machine learning-based image recognition technology. Product recommendation engines are one example of how this technology is changing the game for online retailers. By analyzing customer data such as browsing history and purchase behavior through machine learning algorithms, these engines can suggest personalized product recommendations that increase sales.
Moreover, with the rise of social media platforms and influencer marketing strategies on them, it has become essential for businesses to track their brand presence online visually accurately. Traditionally this task would involve manually searching through thousands of images with relevant tags; now it’s possible to use machine learning-powered image recognition systems to quickly and accurately identify brand logos or products in social media posts.
Applications of Machine Learning in Image Recognition
Machine learning has made significant strides in the field of image recognition, transforming the way we interact with visual data. From identifying objects and faces to recognizing patterns and detecting anomalies, machine learning algorithms have opened up endless possibilities in image recognition.
One of the most prominent applications of machine learning in image recognition is object detection. Traditional computer vision techniques were limited to specific types of objects and required a large amount of manual coding. Machine learning algorithms, on the other hand, can identify a wide variety of objects with high accuracy by analyzing vast amounts of training data.
This has led to various real-world applications such as self-driving cars, security surveillance systems, and even medical imaging for diagnosis and treatment planning. With machine learning-enabled object detection, these systems can efficiently detect obstacles or abnormalities in images and take appropriate actions or provide accurate diagnoses.
Another crucial use case of machine learning in image recognition is facial detection and recognition. This technology uses deep neural networks to analyze facial features, including distance between eyes, shape of the jawline, and unique characteristics like moles or scars. By analyzing these features from thousands of faces, machines can accurately recognize individuals with remarkable precision.
This technology has found its application not only in smartphone unlocking but also in security systems at airports and border control for identity verification. Facial recognition technology has also been used for sentiment analysis in marketing by reading micro-expressions to gauge emotional reactions towards products or services.
Moreover, pattern recognition is another vital aspect where machine learning algorithms excel at identifying recurring patterns within an image dataset. This capability makes it possible to classify images based on different attributes such as color composition or texture similarity automatically.
For instance, e-commerce companies can use pattern classification techniques to recommend similar products based on users’ previous purchases or browsing history. Similarly, healthcare providers can utilize these algorithms to identify cancerous cells by recognizing specific patterns in medical images like mammograms or MRIs.
Anomaly detection is another valuable application enabled by machine learning in image recognition. Traditional methods were often tedious and required manual inspection of images, making it impractical for large datasets. Machine learning algorithms can learn normal patterns from data and flag any unusual instances, leading to more accurate identification of anomalies.
Advantages and Limitations of AI in Image Recognition
The development of Artificial Intelligence (AI) has brought about many revolutionary advancements in the field of image recognition. With the help of machine learning algorithms, computers are now able to accurately recognize and classify images with an efficiency that was previously unimaginable. However, like any other technology, AI also has its own set of advantages and limitations when it comes to image recognition.
Advantages:
1. Speed and Accuracy: One of the key advantages of AI in image recognition is its speed and accuracy. Unlike humans who may take a considerable amount of time to analyze an image, machines equipped with AI can perform this task almost instantly with unmatched accuracy. This makes it ideal for applications where quick and precise analysis is crucial.
2. Scalability: Another major advantage of using AI for image recognition is its scalability. Once trained on a large dataset, the same algorithm can be used to process thousands or even millions of images without any additional effort or cost.
3.Cost-Effective: Compared to manual labor, employing AI for image recognition can be significantly more cost-effective in the long run. It eliminates the need for hiring a large number of employees for repetitive tasks, therefore reducing operational costs.
4.Adaptability: The beauty of AI-based systems is their adaptability. As they continue to process images, they constantly learn from their mistakes and improve their accuracy over time. This makes them highly versatile and capable of handling diverse types and styles of images without any human intervention.
Future Developments in the Field
As machine learning continues to advance and revolutionize image recognition, the future developments in this field are seemingly endless. Researchers are constantly working on new techniques and algorithms to further improve the accuracy and speed of image recognition systems.
One major area of focus for future developments is reinforcement learning. This branch of machine learning involves training an algorithm through a process of trial and error, where it receives feedback and adjusts its actions accordingly. This technique has shown promise in improving image recognition by continuously learning from its mistakes and refining its predictions.
Another exciting development is the use of deep neural networks, a subset of machine learning that mimics the structure and function of human brain cells. Deep neural networks have been used successfully in various fields, including image recognition, but there is still much potential for improvement. As technology advances, we can expect to see even more sophisticated deep neural networks being developed for improved accuracy and efficiency in image recognition tasks.
In addition to these techniques, researchers are also exploring ways to incorporate natural language processing into image recognition systems. This would allow machines not only to recognize images but also understand and describe them in human language. This could have significant applications in areas such as medical imaging, where detailed descriptions are crucial.
Furthermore, efforts are being made to develop more efficient methods for training models using smaller datasets. Currently, large amounts of data are required to train accurate machine learning models for image recognition tasks. However, with advancements in transfer learning – where knowledge from one model can be applied to another – researchers hope to reduce the amount of data needed while maintaining high levels of accuracy.
The integration of artificial intelligence (AI) with other emerging technologies such as augmented reality (AR) and virtual reality (VR) is also expected to play a vital role in the future development of image recognition systems. With AI-powered AR/VR devices becoming increasingly popular among consumers, we can expect seamless integration between these technologies providing enhanced visual recognition and interaction with the user’s environment.
Ethical Considerations with Intelligent Computer Vision
With the rapid advancement of machine learning and artificial intelligence, intelligent computer vision has become increasingly prevalent in various industries. From self-driving cars to facial recognition technology, these systems are continuously improving their ability to understand and interpret visual data. But as with any emerging technology, there are ethical considerations that must be addressed in order to ensure responsible use and development.
Machine Learning
One significant ethical consideration is bias within intelligent computer vision algorithms. These systems learn from existing datasets, which may contain inherent biases due to human-created training data or societal stereotypes. If not properly addressed, these biases can perpetuate discrimination and reinforce societal inequalities. It is crucial for developers to constantly monitor their datasets for bias and actively work towards creating more diverse and inclusive training sets.
Additionally, there are concerns about transparency and explainability when it comes to intelligent computer vision algorithms’ decision-making processes. As these systems often operate on complex deep learning models, it can be challenging for humans to understand exactly how a decision was made. This lack of transparency can create mistrust among users who may feel uneasy about relying on AI-driven systems without being able to fully comprehend how they arrived at a particular conclusion.
In order to address these ethical considerations, companies must prioritize developing responsible AI practices when implementing intelligent computer vision technologies. This includes actively seeking out diverse perspectives during development stages, regularly auditing datasets for bias, ensuring transparency in decision-making processes through explainable AI techniques, and providing clear guidelines on data usage for both consumers and employees.
Moreover, governments also play a vital role in regulating the use of intelligent computer vision to protect individual rights and privacy. As these technologies continue to evolve, it is essential for policymakers to establish ethical guidelines and regulations that hold companies accountable for the responsible development and use of intelligent computer vision systems.
Conclusion
In conclusion, it is clear that machine learning has played a crucial role in revolutionizing image recognition technology. With its ability to constantly learn and improve, machine learning algorithms have significantly increased the accuracy of image recognition systems while also making them more efficient and adaptable. As we continue to advance in this field, we can expect even greater advancements and applications of machine learning in various industries.
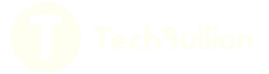