How Do Businesses Use Big Data for Risk Management?
In the fast-evolving realm of risk management, CEOs and data scientists are harnessing the power of Big Data to safeguard their operations. From identifying vulnerabilities through continuous monitoring to enhancing the user experience with data insights, we’ve compiled the diverse experiences of thirteen experts. Their real-world examples provide a roadmap for effectively mitigating risks using data-driven strategies.
- Identify Vulnerabilities Through Continuous Monitoring
- Predict Shipping Delays With Historical Data
- Mitigate Risks With Social Media Monitoring
- Prevent Breaches With Real-Time Data Analysis
- Optimize Supply Chain With Data-Driven Insights
- Reduce Churn by Analyzing User Behavior
- Catch Early PR Issues With Online Monitoring
- Make Quick Decisions With Unified Data Reports
- Adjust Risk Models Using Customer Behavior
- Negotiate With Contractors Using Performance Data
- Anticipate Supply Chain Disruptions With Data
- Detect Fraudulent Transactions With Data Patterns
- Enhance User Experience With Big Data Insights
Identify Vulnerabilities Through Continuous Monitoring
Big Data plays a crucial role in our risk management strategy. We collect and analyze large volumes of data from our clients’ networks, which helps us identify potential vulnerabilities and predict future risks. Our approach involves continuous monitoring and analysis to ensure our clients’ IT infrastructure remains secure.
One specific example involved a client’s network experiencing unusual activity patterns. Our data analysis tools detected these anomalies, indicating a possible security breach. We immediately took action, isolating the affected systems and conducting a thorough investigation. Our swift response, driven by data insights, prevented significant damage and loss of sensitive information.
Regularly monitoring and analyzing network data can provide early warnings of potential threats and help make informed decisions quickly.
Konrad Martin, CEO, Tech Advisors
Predict Shipping Delays With Historical Data
In the decade that we’ve existed, we’ve gathered large data sets, including historical shipment data, traffic patterns, weather reports, and port congestion reports. This data makes up the core of our Big Data and is crucial for strategic decision-making and improving the performance of our shipment tracking platform.
An example of the data-driven insights derived from this data at work in mitigating risks is during the Black Friday and Cyber Monday shopping frenzy. Leveraging machine-learning tools to analyze historical shipment data and port congestion reports, we can predict when our customers are most likely to experience shipping delays.
To help avert this, we share insights with our users, advising them on the best tips for route optimization and shipping methods to use to ensure reliable and on-time delivery of goods.
Clooney Wang, CEO, TrackingMore
Mitigate Risks With Social Media Monitoring
We utilize data from our property management software, customer reviews, and social media to anticipate risks. For example, we closely monitor mentions of our company on Twitter and Facebook. If we notice an uptick in negative comments about a specific property or issue, we investigate immediately.
Recently, several guests mentioned problems with the Wi-Fi at one of our oceanfront condos. We contacted the owner and arranged for a technician to evaluate and upgrade the internet equipment right away. By addressing the issue promptly, we mitigated the risk of losing future bookings or receiving more public complaints.
We also analyze trends in our booking data and customer reviews to identify potential risks. If we see many guests expressing frustration over the lack of amenities or activities at a property, for instance, we work with the owner on solutions to improve the guest experience, such as adding amenities or offering activity recommendations in the area. Using data in this strategic way has helped build loyalty among our customers and prevented the loss of business.
Garrett Ham, CEO, Weekender Management
Prevent Breaches With Real-Time Data Analysis
We use Big Data extensively for risk management. It’s crucial in our line of work to stay ahead of potential threats. Our IT engineers monitor data patterns and anomalies across our clients’ networks. This helps us identify and mitigate risks before they become major issues. One time, we detected unusual login attempts on a client’s network, which turned out to be an early sign of a cyber-attack.
I remember a specific case where our data-driven approach saved a client from a significant security breach. Our system flagged some irregular activity late one night. The data showed multiple failed login attempts from various IP addresses. Our team quickly analyzed the data and determined that these attempts were part of a coordinated attack. We implemented additional security measures, including IP blocking and enhanced authentication protocols.
I’d recommend investing in a powerful data monitoring system. Keep an eye on the patterns and be proactive about addressing any anomalies. Real-time data analysis is key to staying ahead of threats. And always have a response plan ready. In our experience, having a well-trained team and a clear plan made all the difference in preventing what could have been a disastrous breach.
Elmo Taddeo, CEO, Parachute
Optimize Supply Chain With Data-Driven Insights
An efficient and robust supply chain for retail operations involves various complex decisions that depend on each other. Viewing the supply chain comprehensively requires integrating large volumes of data from multiple sources (sales, suppliers, market trends, etc.) and implementing real-time monitoring systems to track inventory, supplier performance, and transportation to enable quick responses to any issues. Big data helps with these decisions by identifying potential risks and optimizing supply chain operations.
A recent example of mitigating risks using data-driven insights is when COVID-19 significantly changed the last-mile delivery network for B2B retailers. Due to work-from-home policies, delivery locations were more spread out in the suburbs, with 1-2 boxes per stop, compared to a larger number of boxes in more centralized office locations. This exposed a general underlying risk in last-mile delivery when total route time demand exceeds temporal delivery capacity, where the challenge is to deliver to all customers on the promised delivery day with the retailer driver (RD) staying within the regular shift hours.
Evolving industry practices include outsourcing some deliveries to on-demand drivers (ODDs), such as Uber and Lyft. Big data and complex AI models help determine the route of the RD, the locations that the ODDs will deliver to, and the drop-off locations where the RD will hand over packages to the ODDs.
Debdatta Sinha Roy, Senior Research Scientist, Oracle
Reduce Churn by Analyzing User Behavior
One particular instance stands out where our data-driven approach proved invaluable. A while back, we noticed an unusual spike in customer churn rates. Rather than panicking, we turned to our Big Data analytics. By analyzing various data points, including user behavior, engagement metrics, and feedback patterns, we identified a correlation between churn and a recent change in our user interface.
The data revealed that a specific update had inadvertently made certain features less accessible, leading to user frustration and eventual churn. Armed with this insight, we promptly rolled back the update and initiated a more user-friendly redesign. This proactive approach not only stabilized our churn rates but also enhanced our overall user experience.
This experience reinforced the critical role of Big Data in risk management. By continuously monitoring and analyzing data, we can quickly identify potential risks, understand their root causes, and implement effective solutions. This data-driven approach is now an integral part of our risk management strategy, helping us mitigate risks and make informed decisions.
Dinesh Agarwal, Founder, CEO, RecurPost
Catch Early PR Issues With Online Monitoring
Using Big Data, my company excels in risk management by continuously monitoring social media and online discussions for any negative sentiment. This constant surveillance enables us to catch early signs of PR issues and address them promptly. Data-driven insights provide us with a clear understanding of the nature and scope of the problem, allowing for precise and effective responses. This method helps in mitigating risks and also in maintaining a positive brand image and public trust.
Kyran Schmidt, Cofounder, Outverse
Make Quick Decisions With Unified Data Reports
Risk increases when data is not transparent enough; this is where Big Data analysis makes such a big difference. It allows us as a business to see the bigger picture. By being able to collect data across all departments and unify it into one report, we are able to track trends, understand patterns, and quickly identify anomalies. Even more importantly, what it does is allow us to have a very quick reaction time.
The pandemic was probably the best revealer of how prepared companies were for mitigating risk. We had already invested, at that point, a considerable amount of resources in Big Data. When businesses started switching off overnight, we were able to quickly identify what needed to be turned off, what we needed to prioritize, how our cash flow was about to be affected, and how we could give ourselves the best chance of a best-case scenario.
We could read in minutes data on our spend, existing contracts, forecasted revenue, operational costs, and we could break it down to answer very specific questions, such as how does moving to a remote environment impact our spend? This would normally take weeks of work to be able to answer, but we could do it in minutes. In addition, all the vending data we have enables us to both manage risk in terms of stock—and our customers manage the risk of wastage by being able to see which food and drink are selling and what isn’t. This enables more efficient stock ordering.
Alexandru Samoila, Head of Operations, Connect Vending
Adjust Risk Models Using Customer Behavior
We use Big Data to manage risks in our finance and insurance business.
We look at a lot of data on customer behavior, like transaction patterns and feedback, to spot potential risks early. This helps us catch and fix issues before they become big problems.
For example, we noticed a spike in insurance claims in a specific area. By digging into the data, we found these claims were linked to certain weather events. We adjusted our risk models and updated our underwriting criteria for that area. We also reached out to our clients with tips on protecting their properties, which helped reduce future claims and strengthened our client relationships.
Big Data also helps us detect fraud. By analyzing patterns in claims data, we can spot unusual activities early and prevent fraud. This saves us money and keeps our services trustworthy.
Rhett Stubbendeck, CEO & Co-Founder, Leverage Planning
Negotiate With Contractors Using Performance Data
I implement Big Data analytics to identify potential hazards and risks in the real estate industry. We are able to accurately assess the level of risk posed by each contractor by leveraging data from various sources, such as project timelines, contractor performance history, financial records, and market trends.
For instance, there was a recent project where our data analysis showed that a contractor had a history of delays and cost overruns on previous projects. This insight allowed us to negotiate stricter deadlines and contract terms with the contractor, ultimately reducing our risk exposure.
Big Data also helps us identify patterns and trends in the real estate market that could pose potential risks to our business. By continuously monitoring market data such as interest rates, housing prices, and consumer behavior, we can anticipate and prepare for potential risks and make informed decisions. I recently used this approach to mitigate the risk of a housing market crash by diversifying our investments and adjusting our pricing strategy.
I often prefer a predictive analytics tool that uses machine learning to assess the risk level of different contractors. This has helped us identify high-risk contractors and prioritize them for closer monitoring, allowing us to proactively address any issues and minimize potential risks.
Michael Benoit, Founder, ContractorBond.org
Anticipate Supply Chain Disruptions With Data
The Big Data analysis carried out in our company enables us to anticipate disruptions in the supply chain and prepare effective contingency plans in advance.
We collect data from various sources, including suppliers, market trends, and logistics networks, to identify potential risks. These data-driven insights enable us to foresee issues such as delays, shortages, or geopolitical impacts that could affect our supply chain. By proactively addressing these risks, we ensure continuity and efficiency in our operations.
To maintain the accuracy and reliability of our data, we use robust data-validation processes and continuously monitor data quality. By doing so, we ensure that our risk management strategies are based on reliable information.
Adam Tishman, Co-Founder, Helix Sleep
Detect Fraudulent Transactions With Data Patterns
Big data and data analytics enable us to identify and mitigate risks more effectively. We leverage data from multiple sources to gain valuable insights into potential risks. An example is using customer transaction data to detect fraudulent activities early. By analyzing patterns in transaction volumes, amounts, times, and locations, our data models can accurately flag suspect transactions.
We then review the flagged transactions and take appropriate action, such as freezing suspect accounts until customers can verify the transactions. This data-driven approach allows us to mitigate fraud risks proactively, minimizing losses and protecting customers.
The key is to continuously improve our data models by feeding in new transaction data and flagged cases. A unique tip is to also leverage external data sources like IP address locations and customer credit histories to enrich our data models and further boost their effectiveness. The combination of big data, data analytics, and domain expertise allows us to mitigate risks in a smart and cost-efficient manner.
Anupreet Kaur, Content And Digital Marketing Manager, Mitt Arv
Enhance User Experience With Big Data Insights
We use Big Data to spot potential risks and better understand our customers’ behaviors and needs. By analyzing trends and customer interactions, we can predict and mitigate issues before they arise. For instance, we monitor patterns in how our customers use our tool and the common challenges they face. This helps us tweak our features and support in real time, preventing minor issues from snowballing into bigger problems.
A clear example of this was when we noticed a recurring drop-off point in our customer onboarding process. We identified a particular step that was causing confusion. We then revamped that part of the onboarding, added more intuitive guidance, and offered additional support resources. As a result, we saw a significant improvement in completion rates and a reduction in support requests related to onboarding. This proactive approach, powered by Big Data, allowed us to enhance the user experience and mitigate the risk of losing new customers.
Josh Bluman, Co-Founder, Hoppy Copy
Related Articles
- How Do Businesses Leverage Big Data to Respond to Emerging Market Trends?
- Enhancing Decision-Making: How Has Big Data Influenced Your Strategic Choices?
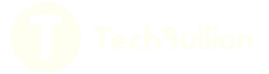