Data engineering in retail is the backbone of modern supply chain management, revolutionizing the way retailers handle data to enhance efficiency and decision-making. It involves the systematic collection, transformation, and analysis of large volumes of data generated across various touchpoints in the retail supply chain.
This includes everything from inventory levels and customer purchasing behavior to logistics and supplier performance.
By leveraging advanced technologies such as big data analytics, machine learning, and cloud computing, retailers can gain real-time insights and predictive capabilities. This data-driven approach enables more accurate demand forecasting, optimized inventory management, and streamlined logistics, ultimately leading to a more responsive and efficient supply chain. In an era where data is a critical asset, data engineering empowers retailers to stay competitive and meet the evolving demands of consumers.
The Role of Data Engineering in Supply Chain Management
Data engineering plays a pivotal role in supply chain management by transforming raw data into actionable insights, thereby enhancing efficiency and decision-making processes.
In the context of retail, data engineering involves the integration and processing of data from various sources such as sales transactions, inventory systems, logistics networks, and customer feedback.
By utilizing advanced analytics and machine learning algorithms, data engineering helps retailers predict demand more accurately, optimize inventory levels, and streamline logistics operations.
It enables real-time tracking and visibility across the supply chain, facilitating quicker response to disruptions and more effective coordination with suppliers.
Additionally, data engineering supports the automation of routine tasks and the identification of inefficiencies, leading to cost savings and improved service levels. In essence, data engineering provides the analytical foundation that empowers retailers to build more resilient, responsive, and customer-centric supply chains.
Key Technologies in Data Engineering:
- Big Data Analytics: This technology enables the processing and analysis of vast amounts of data generated across the supply chain. It helps in identifying patterns, trends, and insights that can drive decision-making and optimize operations. Big data analytics allows retailers to understand consumer behavior, forecast demand, and manage inventory more efficiently.
- Machine Learning and AI: These technologies are crucial for predictive analytics and automation within the supply chain. Machine learning algorithms can analyze historical data to predict future trends, optimize routing for logistics, and improve demand forecasting. AI-powered systems enhance decision-making by providing actionable insights and automating routine tasks, thereby increasing efficiency and reducing errors.
- Cloud Computing: Cloud platforms provide scalable and flexible infrastructure for storing and processing large datasets. They enable real-time data integration and accessibility, ensuring that stakeholders across the supply chain have up-to-date information. Cloud computing also supports advanced analytics and machine learning applications, offering the computational power needed for complex data processing tasks while reducing IT costs and maintenance burdens.
Enhancing Demand Forecasting and Inventory Management
Enhancing demand forecasting and inventory management through data engineering involves leveraging advanced analytics to predict customer demand accurately and optimize stock levels.
By analyzing historical sales data, market trends, and external factors such as seasonal variations and economic conditions, retailers can develop precise demand forecasts. Machine learning algorithms refine these predictions by continuously learning from new data, allowing for dynamic adjustments.
This accuracy in forecasting helps retailers maintain optimal inventory levels, reducing both stockouts and excess inventory.
Furthermore, real-time data integration across supply chain systems ensures that inventory information is always current, enabling better coordination between purchasing, warehousing, and sales. This not only improves operational efficiency but also enhances customer satisfaction by ensuring that products are available when and where they are needed.
Improving Supplier Relationship Management
Improving supplier relationship management through data engineering involves the use of data analytics solutions to foster better collaboration, transparency, and efficiency between retailers and their suppliers. By integrating and analyzing data from various sources, such as supplier performance metrics, order histories, and market conditions, retailers can gain a comprehensive view of their supply network.
This insight allows for more informed decision-making regarding supplier selection, contract negotiations, and performance evaluations. Predictive analytics can identify potential disruptions or delays, enabling proactive communication and problem-solving.
Additionally, real-time data sharing enhances transparency, ensuring that suppliers have access to up-to-date demand forecasts and inventory levels. This collaborative approach not only strengthens partnerships but also leads to improved reliability, reduced costs, and a more resilient supply chain overall.
Streamlining Logistics and Distribution
- Optimizing Transportation Routes: Utilize machine learning algorithms to determine the most efficient routes, minimizing transit times and reducing fuel costs.
- Enhancing Real-Time Tracking: Implement real-time tracking systems for better visibility and coordination, allowing for quick responses to disruptions and delays.
- Improving Warehouse Operations: Analyze data to streamline warehouse processes, such as picking, packing, and loading, leading to faster and more accurate order fulfillment.
- Efficient Inventory Placement: Use data insights to strategically position inventory across distribution centers, ensuring products are closer to demand points and reducing delivery times.
- Reducing Operational Costs: Identify and eliminate inefficiencies in the logistics network, leading to cost savings in transportation, labor, and storage.
- Increasing Customer Satisfaction: Ensure timely and accurate deliveries by optimizing logistics and distribution processes, resulting in improved service levels and customer loyalty.
- Dynamic Scheduling: Implement data-driven scheduling systems that adjust in real-time to changing conditions, ensuring resources are utilized effectively.
- Proactive Problem-Solving: Use predictive analytics to anticipate potential issues in the supply chain, allowing for proactive measures to mitigate disruptions.
Real-Time Data Integration and Decision Making
Real-time data integration and decision-making are critical components of modern supply chain management, enabled by data engineering techniques.
By integrating data from various sources, such as sales transactions, inventory levels, and external market factors, retailers can create a unified view of their supply chain in real-time.
This ensures that stakeholders have access to accurate and up-to-date information, allowing for quicker and more informed decision-making. With real-time insights, retailers can respond rapidly to changes in demand, supply, or market conditions, optimizing operations and minimizing risks.
Additionally, advanced analytics and machine learning algorithms enable retailers to automate decision-making processes, such as dynamic pricing or inventory replenishment, based on real-time data streams. This agile and data-driven approach not only improves operational efficiency but also enhances agility, resilience, and competitiveness in today’s dynamic retail landscape.
Challenges and Considerations in Implementing Data Engineering:
- Data Quality and Consistency: Ensuring the accuracy, completeness, and consistency of data across multiple sources can be a significant challenge. Incomplete or inconsistent data can lead to inaccurate analysis and decision-making.
- Data Security and Privacy: Retailers must address concerns regarding data security and privacy, especially when handling sensitive customer information. Compliance with regulations such as GDPR and CCPA is crucial to protect customer data and maintain trust.
- Integration Complexity: Integrating data from disparate sources and systems can be complex and time-consuming. Retailers may face challenges in harmonizing data formats, resolving data conflicts, and maintaining data integrity throughout the integration process.
- Scalability and Performance: As the volume of data grows, retailers need scalable data engineering solutions that can handle increasing data loads while maintaining performance and responsiveness.
- Skills Gap and Talent Shortage: Data engineering requires specialized skills in areas such as data analytics, machine learning, and cloud computing. Retailers may struggle to find and retain skilled professionals to design, implement, and maintain data engineering solutions.
- Cost Considerations: Implementing data engineering solutions can involve significant upfront costs for infrastructure, software licenses, and personnel. Retailers must carefully evaluate the return on investment and long-term sustainability of data engineering initiatives.
- Change Management: Introducing data engineering initiatives may require cultural and organizational changes within the retail organization. Resistance to change and lack of buy-in from stakeholders can hinder the successful implementation of data engineering projects.
The Future of Supply Chain Management with Data Engineering:
Predictive Analytics: Data engineering will enable the widespread adoption of predictive analytics in supply chain management, allowing retailers to anticipate demand, identify potential disruptions, and optimize operations proactively.
Real-Time Decision-Making: Advances in data engineering will facilitate real-time decision-making across the supply chain, enabling retailers to respond quickly to changing market conditions, customer preferences, and supply chain events.
AI-Powered Automation: AI and machine learning technologies will play a central role in automating routine tasks and optimizing processes within the supply chain. This includes tasks such as demand forecasting, inventory replenishment, and route optimization.
Blockchain Integration: Data engineering will enable the integration of blockchain technology into supply chain management, providing greater transparency, traceability, and security throughout the supply chain.
Supply Chain Collaboration Networks: Data engineering will facilitate the creation of collaborative supply chain networks, allowing retailers to share data and insights with suppliers, logistics partners, and other stakeholders to improve coordination and efficiency.
Sustainable Supply Chains: Data engineering will enable retailers to analyze environmental impact data and optimize supply chain operations for sustainability. This includes reducing waste, minimizing carbon emissions, and promoting ethical sourcing practices.
Personalized Supply Chains: Data engineering will enable retailers to create personalized supply chains tailored to individual customer preferences and behaviors, providing a more seamless and personalized shopping experience.
Overall, the future of supply chain management with data engineering will be characterized by increased agility, efficiency, and innovation, enabling retailers to stay competitive in an increasingly complex and dynamic marketplace.
The Impact of Data Engineering on Retail Supply Chains
In conclusion, the impact of data engineering on retail supply chains is transformative and far-reaching. By harnessing the power of advanced analytics, machine learning, and real-time data integration, retailers can revolutionize their supply chain management practices. Data engineering enables retailers to make more informed decisions, optimize operations, and respond quickly to changing market dynamics.
From enhancing demand forecasting and inventory management to streamlining logistics and improving supplier relationships, data engineering drives efficiency, agility, and competitiveness throughout the retail supply chain.
Looking ahead, continued advancements in data engineering technologies promise even greater opportunities for innovation and improvement in retail supply chain management, ensuring that retailers can meet the evolving demands of consumers and maintain their competitive edge in the marketplace.
Author Bio:
Stella M is the Lead SEO Analyst and a seasoned tech content writer at Indium Software. With over 8+ years of experience, she excels in driving organic growth and crafting engaging, high-ranking content. Stella combines expert SEO strategies with compelling writing to boost online visibility and audience engagement.
Read More From Techbullion
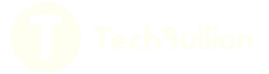