Artificial Intelligence has fundamentally changed how we approach data analytics, offering unprecedented capabilities in processing and interpreting large datasets. For tech professionals, understanding these transformations is crucial for staying competitive in the rapidly evolving digital landscape. The integration of AI into analytics has not only accelerated data processing but has also unveiled patterns and insights that were previously impossible to detect through traditional methods.
The Evolution of Data Analytics
The journey of data analytics has been marked by significant transformations over the years. Traditional analytics relied heavily on predetermined rules and human interpretation, often limiting the depth and speed of insights. However, the integration of AI has introduced more sophisticated approaches to data analysis, revolutionizing how we process and understand information.
Key Transformations in Modern Analytics
- Automated pattern recognition that identifies subtle trends in complex datasets
- Predictive modeling with significantly improved accuracy rates
- Real-time analysis capabilities for instant decision-making
- Natural Language Processing for unstructured data analysis
Machine Learning’s Impact on Analytics
Machine learning represents one of the most significant advancements in modern analytics. Unlike traditional analytical methods that require explicit programming for each scenario, ML algorithms can learn from data, adapting and improving their accuracy over time. This self-learning capability has revolutionized how we handle complex, multi-dimensional datasets, making it possible to uncover insights that would be impossible to find through conventional analysis.
The real power of machine learning in analytics lies in its ability to scale. As datasets grow larger and more complex, ML algorithms continue to perform effectively, often improving their accuracy with additional data. This scalability has made it possible to analyze entire business ecosystems in real-time, providing insights that drive both operational efficiency and strategic planning.
Real-World Applications and Implementations
The practical applications of AI in analytics span across numerous industries, each demonstrating unique and innovative uses of the technology. Modern platforms like betzoid.com showcase how AI-powered analytics can transform traditional data analysis into dynamic, predictive insights that drive business value. Financial institutions use these advanced analytics for risk assessment and fraud detection, while healthcare providers leverage them for patient outcome prediction and treatment optimization.
Industry-Specific Applications
- Financial services: Risk assessment and fraud detection systems that process millions of transactions in real-time
- Healthcare: Patient outcome prediction and treatment optimization through analysis of complex medical data
- Manufacturing: Predictive maintenance systems that reduce downtime and optimize production efficiency
Deep Learning and Advanced Analytics
Deep learning has emerged as a particularly powerful tool in the analytics landscape. Its neural network architectures excel at processing unstructured data like images, text, and voice recordings – types of data that traditional analytics struggle to handle effectively. This capability has opened up entirely new possibilities for data analysis, enabling organizations to extract meaningful insights from previously untapped data sources.
The impact of deep learning extends beyond just handling complex data types. These systems can identify subtle patterns and relationships within data that might escape even experienced human analysts. For instance, in medical imaging analysis, deep learning systems have demonstrated the ability to detect patterns indicative of diseases at early stages, sometimes outperforming human experts in accuracy.
Implementation Challenges and Best Practices
While the benefits of AI-powered analytics are clear, organizations face several significant challenges in implementation. Data quality and preparation remain critical concerns, as AI systems require high-quality, well-structured data to function effectively. Organizations must invest in robust data governance frameworks and quality control measures to ensure their AI analytics can produce reliable insights.
Essential Implementation Steps
- Establish clear business objectives and success metrics before implementation
- Invest in proper data governance and quality control measures
- Develop comprehensive training programs for team members
- Implement robust testing and validation procedures
Future Trends and Opportunities
The future of AI in data analytics promises even more revolutionary developments. Automated analytics is becoming increasingly sophisticated, with systems capable of handling the entire analytics pipeline from data preparation to insight generation with minimal human intervention. Edge analytics is gaining prominence, bringing computational power closer to data sources and enabling real-time decision-making with reduced latency.
Perhaps most significantly, the field is moving towards more explainable AI systems. These advanced analytics platforms can not only provide insights but also clearly explain their reasoning process. This transparency is crucial for building trust in AI-driven decisions and ensuring accountability in critical applications.
Conclusion
The transformation of data analytics through AI represents more than just a technological advancement – it’s a fundamental shift in how we understand and utilize data. For tech professionals, staying current with these developments is not optional but essential for future success. As AI continues to evolve, we can expect even more powerful and sophisticated analytical capabilities to emerge, further revolutionizing the field of data analytics and its applications across industries.
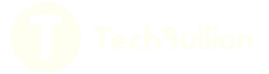