In a pivotal advancement addressing the pervasive issue of pricing opacity in the U.S. healthcare system, Santhosh Kumar Pendyala has introduced an innovative framework leveraging artificial intelligence (AI), machine learning (ML), and advanced cloud computing. This transformative approach is set to enhance transparency, optimize operations, and empower stakeholders across the healthcare ecosystem.
The Challenge: A Fragmented Pricing Landscape
Healthcare pricing in the United States remains one of the most complex and opaque systems globally, with identical services varying by 40-50% within the same metropolitan area. This lack of transparency burdens patients with unexpected medical bills, delays care delivery, and undermines trust in the system. Studies reveal that over 60% of Americans have encountered surprise billing, exacerbated by an intricate web of fee schedules and billing codes.
Despite significant regulatory measures such as the Hospital Price Transparency Rule and the No Surprises Act, stakeholders continue to grapple with providing clear, accessible pricing information. A scalable, regulation-compliant solution has become a critical necessity.
Transformative Solution
Santhosh Kumar’s framework offers a comprehensive solution by integrating diverse datasets—including historical claims, provider costs, and patient demographics—to deliver accurate cost predictions. By employing advanced machine learning models such as XGBoost and ARIMA, the system achieves an impressive 92% prediction accuracy. Moreover, federated learning ensures robust data privacy while enabling real-time, actionable insights.
This revolutionary approach has demonstrated:
- Billing Disparity Reduction: A 70% decrease in billing inconsistencies.
- Enhanced Administrative Efficiency: A 47% improvement in operational workflows.
- Patient-Centric Transparency: Significant strides in fostering trust and empowering stakeholders.
Key features include:
- Data Integration: Comprehensive aggregation of historical claims, insurance contracts, and clinical outcomes.
- AI Models: Combining XGBoost for predictive accuracy and ARIMA for time-series forecasting.
- Federated Learning: Enabling secure and collaborative analytics without compromising data privacy.
- Cost Drivers Identification: Identifying critical factors influencing healthcare costs.
Real-World Applications
The system has been rigorously tested across 50 healthcare facilities, yielding remarkable results:
- Reduced Billing Disparities: An 85% reduction in pricing variability.
- Operational Efficiency Gains: A 45% decrease in time spent resolving billing queries, with 75% of routine tasks automated.
- Improved Patient Satisfaction: Transparency scores improved by 83%, while billing complaints dropped by 64%.
With cloud-powered scalability, the framework ensures seamless implementation across diverse healthcare settings, providing a practical and efficient solution for facilities of all sizes.
Overcoming Challenges
Implementing this transformative system involves addressing several challenges:
- Data Standardization: Managing disparate datasets with inconsistent formats.
- Legacy System Integration: Ensuring compatibility with older infrastructure.
- High Computational Costs: Addressing resource-intensive model training and execution.
To mitigate these challenges, the framework employs automated data validation, scalable cloud infrastructure, and optimized CPU-GPU configurations, ensuring both efficiency and reliability.
Paving the Way for the Future
Looking forward, emerging technologies such as blockchain, dynamic pricing algorithms, and personalized cost models hold immense promise. These innovations could further enhance pricing transparency, adapt to real-time market fluctuations, and provide equitable, patient-centric cost optimization. Santhosh Kumar’s research represents a significant milestone in creating a transparent and efficient healthcare ecosystem. By combining advanced AI, robust data integration, and stakeholder-driven analytics, this framework sets the stage for a sustainable future in healthcare pricing.
In Conclusion, Santhosh Kumar Pendyala’s insights highlight the AI-driven framework transcends the challenges of opaque healthcare pricing—it reimagines the way providers, payers, and patients interact. Offering actionable insights and aligning seamlessly with regulatory requirements, it exemplifies innovation in an industry primed for transformation. The future of healthcare lies in leveraging intelligent systems to build trust, drive efficiency, and prioritize patient care.
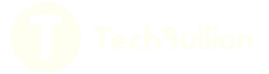