TechBullion invited Pramod Nammi, an accomplished Product Manager, to share his expert insights on leveraging generative AI (GenAI) in product management. In this interview, Nammi discusses the emerging GenAI toolkit and its transformative potential in product development, from enhancing creativity and automating workflows to data-driven decision-making. His expertise sheds light on how GenAI tools are becoming essential assets for modern product managers, driving innovation and efficiency in today’s competitive landscape
- Setting the Stage: The GenAI Revolution:
- The GenAI landscape is expanding at breakneck speed. Can you paint a picture of the essential tool categories that every PM should have in their arsenal to harness the full potential of GenAI?
- At the foundational level, PMs will need to have a basic understanding of the Machine Learning methods used in GenAI. These are : supervised learning, unsupervised learning and mixed learning (semi-supervised). It is not necessary to have a working knowledge of them, but knowing the pros and cons of them, costs associated and use-cases they are better suited for should be part of the tool-kit for PMs.
- Then comes the Large-Language-Models and how they are built and what their advantages and limitations are. For example, GPT-3 is a massive language model with 175 billion parameters. It was trained using unsupervised learning on a large dataset comprising 500 billion tokens of internet text. Its primary function is to predict the next word in a sequence based on preceding words. Here, it is important to have an understanding of the various LLMs out there today, and do a competitive analysis of them regularly to be on top of where the technology is going.
- And lastly, the techniques or ways in which GenAI can be bolted on to products :
- Prompt Engineering : best ways to give instructions and queries to the model to get the right output from it
- Retrieval Augmented Generation (RAG) : useful when there is external data to be accessed and processed by the LLM, which it was not (or cannot be) trained on. Can help in preventing hallucinations by LLMs.
- Fine-tuning an existing large language model
- Bespoke model training
- Synthetic data generation : for when your product or use-case needs high-quality and high-volume data to train on. LLMs can come handy to generate such data without compromising privacy and data-integrity.
- Evolving Skill Sets:
- With GenAI transforming the way we work, how are the core competencies of a product manager being redefined?What skills should PMs prioritize to stay ahead of the curve?
- The number 1 skill is to be able to evaluate how GenAI can accelerate the path to value creation. There are certain times when GenAI is useful and others where it is not suited. Simply adding a chat interface with an LLM is not going to cut it. Being able to assess this fit is a crucial skill that I recommend PMs have.
- I would encourage people to think about where the value of GenAI lies in their product stack.
- Saving costs by accelerating testing, prototyping and research
- Adding to the product value-proposition by solving user-problems with AI assistance
- Using AI brings certain risks to users. This means PMs should have a trust strategy for their products to ensure the highsted standards for user-privacy, integrity and product quality
- Handling hallucinations and bias
- Providing sources or LLM’s chain-of-thought for reasoning to increase transparency
- Giving users the ability to give feedback on the AI interactions and fine-tuning the AI models without compromising user-privacy
- Fueling Growth:
- Let’s talk about tangible results. How can GenAI tools accelerate product growth? Can you share specific examples where GenAI has driven significant improvements in product development, user engagement, or market penetration?
- At a high-level, product growth comes from two levers – 1. reducing costs for users by solving the problems inexpensively. And 2, increasing the value of the product for users by solving more problems or solving the same problems much better than the competition.
- We see GenAI transforming this value-chain at all levels today.
- On one side, we have the large and established organizations like Microsoft, Google and Meta incorporating GenAI in their products : Microsoft with their co-pilot PCs, Google with their search product and Meta with Gen AI content creation inside their consumer apps. These use-cases are strengthening the product value proposition by solving user-problems and reducing user-effort to get their tasks done.
- On the other side, we have entirely new categories of startups focusing on content creation, research, AI assistants and co-pilots for niche categories. We have consumer products like Canva and Grammarly that have new subscription tiers for users that want to use their GenAI offering. Gamma and Tome are AI driven presentation building products that are gaining traction and VC funding. Synthesia and Descript are AI based video avatar generators targeting both business and consumer use-cases.
- Products like Anyword and Writesonic are accelerating time-to-value for marketing use-cases, reducing costs for businesses to create marketing materials, SEO optimization and more.
- More examples can be found here to get a glimpse of the scale of GenAI based startups in 2024 : https://startupsavant.com/startups-to-watch/generative-ai
- Navigating Ethical Waters:
- As PMs embrace GenAI, what ethical considerations should be top of mind? How can we ensure responsible use and avoid potential pitfalls like bias or misinformation?
- A big factor today is how the foundational models are being trained with increasingly large and complex data that humans have produced so far. It goes without saying that there is as much bad and dangerous data as there is good and productive data. So the imperative is to exercise caution in not only selecting the data used for training, but also being pragmatic in assessing the results that the models output. There is a lot of gray area on what is considered good or bad. So I think the big area here is policy making and governance both at a corporate and governmental level.
- In terms of what PMs should consider, I would encourage establishing trust strategy that comprehensively addresses bias, data integrity, privacy and prevention of inadvertent harm
- Security is another key factor to bear in mind. Things like prompt injection, circumvention of filters put in place to prevent harmful output are a reality that PMs should address when working with GenAI for their products.
- Seamless Integration:
- Successfully integrating GenAI tools into existing workflows can be challenging. What strategies or best practices can PMs adopt to ensure seamless adoption and maximize the value derived from these tools?
- Here is a checklist I would use :
- What user-problem is the GenAI feature solving?
- Why is GenAI the best tool to solve this problem?
- What are the costs associated with solving this problem at scale with GenAI?
- What are the risks associated with GenAI in the scenarios that users would interact with it?
- Which model best suits the scenarios in terms of price to performance ratio?
- Do you need the model to be trained on new data to better suit your product’s jobs-to-be-done? Then consider fine-tuning the model.
- Do you need the model to access new information regularly to answer user queries? Then consider implementing Retrieval-Augmented-Generation(RAG) along with the model you picked above.
- Do you have a trust strategy defined and implemented?
- Can users give feedback on the quality of the GenAI output? How would you use this feedback to make the model better?
- This is not a comprehensive checklist, but covers the most important factors involved.
- Here is a checklist I would use :
- Gazing into the Crystal Ball:
- The future of GenAI and product management is full of possibilities. What emerging trends or technologies should PMs keep an eye on to stay at the forefront of innovation?
- I think we are still at the beginning of the GenAI trend life-cycle. If you think about it in terms of the Gartner hype-cycle, we may still be at the peak of inflated expectations phase, before the trend goes into the trough of disillusionment and then back up into being the norm and accessible by everyone at scale.
- And so there are a lot of ways GenAI could go. Here are some things what I’m excited about:
- Right now the interactions with GenAI are either textual or image based for the most part. But I think we’re very close to multi-modal GenAI products where people can share videos, images and multimedia as part of their prompts to the LLMs, and have all of that processed to solve their problems. This will unlock a lot of value in jobs that require deep-research, high-productivity and content creation.
- Another area is personal assistants: to be able to perform actions on behalf of the user automatically, like booking tickets, making reservations, making appointments and even advanced tasks like finding and negotiating the best deals on behalf of users for things like buying goods online, securing loans etc.
- SImilar to personal assistants, we could see professional assistants that are trained on highly-specialized skills : architecture, interior decor, design and education. Users can interact with these assistants or agents to have a personalized experience when solving problems.
- In the entertainment sector, production of video games and movies will be accelerated with AI co-pilots assisting developers and producers. And the content itself might be dynamically generated to be personalized for the user.
- Words of Wisdom:
- What advice would you give to aspiring or seasoned product managers who are looking to master the GenAI tool-kit and accelerate product growth in this new era of technological advancement?
- Be curious about the technology and its applications. As always, starting with the user-problem to solve is the right track to go with.
- Try your hands on various GenAI tools and applications. Keep a catalog of the skills and capabilities that these tools unlock.
- Regularly audit your product to identify gaps that GenAI can address. Not every gap can be solved by bolting on a chatbot or GenAI solution.
- Develop your own frameworks to assess the GenAI skill ←→ Jobs-to-be-done fit
- Force an exercise where you try to solve the gap in your product without GenAI, and assess the merits and drawback of that approach. This will build conviction on whether GenAI is the right fit for the product.
- Keep track of the GenAI space by following newsletters, podcasts and blogs.
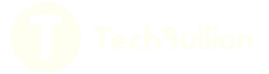