In today’s realm, Data management and engineering are transforming law enforcement practices, offering groundbreaking capabilities for crime prevention and resolution. The integration of advanced analytics, machine learning, and big data processing has revolutionized traditional policing, creating opportunities for more effective public safety measures. However, these technologies come with their set of ethical challenges. Ramalakshmaiah Panguluri, explores the innovations in data-driven law enforcement, highlighting their potential and the need for responsible implementation.
Real-Time Analytics for Crime Prevention
The integration of real-time data processing has revolutionized crime prevention. Technologies such as edge computing, high-speed networks, and cloud platforms allow law enforcement agencies to gather and analyze data in real time, enabling swift responses and smarter decisions. With tools like real-time facial recognition, officers can be immediately alerted to known suspects in busy areas, potentially stopping crimes before they happen. This proactive approach enhances public safety, improves resource allocation, and fosters more efficient policing strategies overall.
Predictive Analytics and Resource Allocation
Law enforcement agencies increasingly rely on predictive analytics to identify crime patterns and anticipate future criminal activities. Machine learning algorithms analyze historical crime data to reveal trends not easily identifiable by human analysts. Geospatial analysis maps crime data, pinpointing hotspots and tracking the movement of criminal activities. Natural language processing extracts valuable insights from unstructured data, such as police reports and social media posts. Predictive policing models built on these technologies can forecast potential crime locations with high accuracy, allowing for optimized resource allocation and more effective patrol strategies.
Enhanced Crime-Solving Techniques
Data-driven approaches have led to enhanced crime-solving techniques, integrating diverse data sources such as surveillance footage, social media data, mobile phone records, and DNA databases. By synthesizing data from various sources, law enforcement agencies can create a holistic view of criminal networks and activities, uncovering previously unknown connections between suspects and aiding in complex investigations. The ability to prioritize cases based on the likelihood of resolution ensures efficient use of resources. Advanced pattern recognition also allows for linking seemingly unrelated crimes across jurisdictions, aiding in the identification of serial offenders.
Improved Transparency and Accountability
The shift toward data-driven policing has brought about improved transparency and accountability within law enforcement agencies. Data-driven decision-making allows for evidence-based policy formulation and objective criteria for resource allocation. Real-time performance dashboards and objective outcome-based metrics enable continuous improvement through data feedback loops. By basing decisions on empirical data, law enforcement agencies can justify their actions to the public, fostering trust and legitimacy. The CompStat model, which uses data to analyze crime trends and allocate resources, has been widely credited with significant crime reductions.
Ethical Concerns in Data-Driven Policing
Data-driven policing presents potential benefits, such as improved crime prevention, but also raises significant ethical issues. Privacy concerns arise from extensive data collection, including surveillance technologies like facial recognition, which may lead to misuse or overreach. Additionally, predictive policing algorithms can reinforce existing biases, disproportionately targeting marginalized communities and exacerbating systemic inequalities. These concerns highlight the need for transparent, accountable measures to ensure that data-driven practices promote fairness, safeguard privacy, and support equitable law enforcement, rather than deepening social disparities.
Comparative Analysis: Education and Creative Fields
Data management and engineering’s impact extends to education and creative fields. In education, adaptive learning personalizes curricula using student data, similar to predictive policing’s resource allocation. In creative fields, recommendation algorithms tailor content to preferences, akin to law enforcement’s crime pattern focus. However, unique ethical concerns arise, such as student privacy and bias in education, and the balance between artistic vision and data-driven creation in the creative industries.
In conclusion, Ramalakshmaiah Panguluri emphasizes that the integration of data management and engineering in law enforcement signifies a considerable advancement in policing capabilities. While these technologies offer enhanced public safety and crime-solving potential, they also bring forth ethical considerations that necessitate a delicate balance between innovation and the protection of civil liberties. By drawing parallels with education and creative fields, it becomes evident that data-driven approaches carry transformative power across various sectors. Moving forward, collaboration among law enforcement agencies, policymakers, technology developers, and communities will be essential to ensure that data-driven strategies are implemented responsibly, fostering societal progress while upholding democratic values.
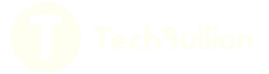