In the ever-evolving landscape of insurance, one technological advancement is making waves like never before – Big Data. Gone are the days of generic policies and rigid risk assessments; now, we’re entering into a world where every policy can be tailored to individual needs and preferences. Welcome to the era of personalized insurance! In this blog post, we’ll dive deep into how harnessing Big Data is transforming the insurance industry from risk assessment to policy offerings. Get ready for an exciting exploration as we unlock the potential of data-driven decisions and discover how they benefit both insurers and customers alike.
Introduction to Big Data and Insurance Technology
In the fast-paced, ever-evolving world of insurance, technology plays a crucial role in shaping the industry. With the rise of big data, insurance companies are now able to harness vast amounts of information and use it to improve their services in almost every aspect.
Big data refers to large sets of complex and constantly evolving data that cannot be analyzed using traditional methods. This includes both structured data (such as numbers or dates) and unstructured data (such as text or images). It is generated from various sources such as social media platforms, customer transactions, online searches, and more.
Insurance companies have always relied heavily on data for risk assessment and policy pricing. However, with advancements in technology and the massive increase in available data, they can now delve much deeper into this process. By leveraging big data analytics tools and techniques, insurers can gain valuable insights that were previously unattainable.
One significant area where big data is making a significant impact is risk assessment. Traditional methods used by insurance companies for assessing risks involved analyzing historical claims data within specific parameters. However, with big data analysis techniques like predictive modeling algorithms and machine learning, insurers can analyze vast amounts of relevant information at a granular level to identify potential risks accurately.
The Role of Big Data in Risk Assessment for Insurance Companies
Big data has completely transformed the way insurance companies approach risk assessment. Traditionally, insurance companies would rely on historical data and general population statistics to determine risk factors and calculate premiums. However, with the advent of big data, insurers now have access to a vast amount of information that provides a more accurate and personalized understanding of risk.
One of the primary benefits of using big data in risk assessment is the ability to gather and analyze large amounts of real-time data from various sources. With advancements in technology, insurers can now collect data from multiple channels such as social media, online transactions, IoT devices, telematics sensors in vehicles, wearable fitness trackers, and more. This wealth of information allows insurance companies to assess risks beyond traditional factors like age or credit score.
Moreover, big data analytics tools utilize complex algorithms and machine learning techniques to process this massive volume of data quickly. These advanced tools can identify patterns and trends that humans may not have noticed during manual analysis. As a result, insurers can make more informed decisions based on extensive analyses rather than intuition or past experiences.
Another key role that big data plays in risk assessment is enabling predictive modeling for identifying potential high-risk customers. By analyzing historical claims records along with other relevant current information like medical history or driving habits using predictive models, insurers can accurately predict future losses for specific individuals. This helps them customize their policies accordingly by offering tailored coverage options at competitive prices.
How Big Data Affects Premiums and Policies
As the insurance industry continues to evolve with advancements in technology, one of the most significant changes has been the use of big data. Big data refers to the large sets of structured and unstructured information that can be analyzed to uncover patterns, trends, and insights. In the insurance sector, this data is collected from various sources such as social media, digital platforms, and customer interactions.
The impact of big data on insurance has been extensive and has led to a shift in how premiums are calculated and policies are designed. Here’s a detailed look at how big data affects premiums and policies.
Risk Assessment: Big data has enabled insurers to gather vast amounts of information about their potential policyholders. This includes factors like demographics, medical records, financial history, and even driving habits. With this rich pool of data, insurers can get a more accurate understanding of an individual’s risk profile. Instead of relying on traditional methods like questionnaires or credit scores alone, big data allows for a holistic view that considers multiple variables. This results in more precise risk assessment which can lead to fairer premiums for customers who may have previously been overcharged due to limited information.
Personalized Policies: One-size-fits-all policies are becoming obsolete as insurers utilize big data to create personalized policies based on an individual’s specific needs and lifestyle choices. For example, car insurance companies can now offer usage-based policies where premiums vary depending on how often someone drives or even their driving behaviors like speed or braking habits recorded by telematics devices installed
Case Studies: Examples of Successful Implementation of Big Data in Insurance
Big data has revolutionized the insurance industry, allowing for more accurate risk assessment and personalized policies for customers. But how exactly have companies implemented big data to improve their operations? In this section, we will look at some real-life case studies of insurance companies that have successfully harnessed big data to transform their business.
1. Lemonade: Using AI for Faster Claims Processing
Lemonade is a digital insurance company that has effectively used big data and artificial intelligence (AI) to improve its claims processing. With traditional insurance companies, claims can take weeks or even months to process, causing frustration and inconvenience for customers. However, by harnessing big data and AI algorithms, Lemonade is able to provide faster claims processing with minimal human intervention.
The company’s AI assistant named Jim collects data from various sources such as social media, government records, and weather reports to assess the validity of a claim quickly. For example, if a customer files a claim for theft but had checked into a hotel on social media at the time of the incident, Jim can detect this and flag it as suspicious. This reduces fraud attempts and speeds up legitimate claims processing.
As a result of using big data and AI technology in its operations, Lemonade can settle 30% of its claims instantly without any human involvement.
2. Metromile: Providing Personalized Policies Based on Driving Habits
Metromile offers usage-based car insurance where premiums are based on how much a customer drives. The company uses big data and telematics devices to collect real-time information on driving behavior, such as distance traveled, speed, and time of day. This allows them to tailor policies specifically for each customer based on their individual driving habits.
Through big data analysis, Metromile can accurately predict the risk profile of each driver and adjust premiums accordingly. For example, if a driver is known to take frequent long trips during peak traffic hours, their premium may be higher than someone who only drives short distances during off-peak times.
This approach benefits both the customer and the insurance company – customers pay for what they use and can potentially save money if they drive less, while Metromile can accurately price their policies and mitigate risk.
3. Oscar Health: Predictive Analytics for Better Risk Assessment
Oscar Health is a health insurance startup that uses big data analytics to improve risk assessment and provide personalized healthcare services. The company considers various sources of data such as claims history, lab results, doctor visits, prescription drug usage, and more to identify patterns in patients’ health conditions.
By analyzing this vast amount of data with predictive analytics algorithms, Oscar Health can accurately assess an individual’s risk profile and suggest preventive measures to avoid future health problems. This not only helps them provide better coverage and care for their customers but also reduces healthcare costs for both the company and its clients.
4. Prudential: Improving Underwriting with Data Analytics
Prudential, a leading insurance company, has harnessed big data to improve underwriting – the process of assessing risks and determining premium prices. Using data from sources such as medical records, pharmacy claims, and driving records, Prudential has developed predictive models that can accurately evaluate an individual’s risk profile.
By analyzing this data, the company can identify potential health risks, such as chronic conditions or lifestyle habits, that may affect a person’s life expectancy. This allows them to adjust premiums accordingly and make more informed decisions when insuring individuals.
As a result of implementing big data analytics in their underwriting process, Prudential has increased efficiency and accuracy while reducing cost and mitigating risks.
Addressing Privacy Concerns and Ethical Issues
In recent years, the use of big data in the insurance industry has become increasingly prevalent. With the ability to collect and analyze vast amounts of information, insurance companies are able to gain valuable insights into their customers’ behaviors and risks. This has led to the development of innovative products such as personalized policies that cater to individual needs and risk profiles.
While there are certainly many benefits to be gained from harnessing big data in insurance, it is important to address privacy concerns and ethical issues that may arise. In this section, we will discuss some of these concerns and provide insight on how they can be addressed.
1. Data Privacy Protection:
As with any type of personal data collection, there are legitimate concerns about how insurers handle sensitive information obtained through big data analysis. Customers may worry about their personal information being shared with third parties without their consent or used for discriminatory purposes.
To address these concerns, insurance companies must adhere to strict legal regulations surrounding data privacy protection. They must also clearly communicate their policies on data collection, usage, and sharing with customers in a transparent manner. Additionally, insurers should implement strong security measures to safeguard customer data against cyber threats.
2. Biased Algorithms:
One major concern with using big data in insurance is the potential for biased algorithms to create discrimination against certain groups or individuals. This can happen if the algorithms are trained on biased historical data or contain inherent biases due to societal factors.
Future Predictions for the Use of Big Data in Insurance
There is no doubt that big data has transformed various industries, including insurance. With the exponential growth of data being generated every day, the potential for its use in insurance continues to expand. As we look towards the future, it is clear that big data will play a crucial role in shaping the insurance landscape.
Here are some key predictions for how big data will be used in insurance in the years to come:
1) Improved risk assessment:
Big data analytics have already proven to be useful in accurately assessing risks. As more and more sources of data become available, such as social media activity and Internet of Things (IoT) devices, insurers will have an even better understanding of their customers’ behaviors and needs. This will enable them to price policies more accurately and tailor coverage based on individual risk profiles.
2) Personalized policies:
In line with improved risk assessment, personalized policies are expected to become more common in the insurance industry. Utilizing big data analysis, insurers can offer customized products that meet specific customer needs. For example, a fitness tracker could provide real-time health information which would enable an insurer to offer lower premiums for those leading healthy lifestyles.
3) Fraud detection:
One major challenge faced by insurance companies is detecting fraudulent claims. Big data analytics can assist in this area by identifying patterns and anomalies that indicate fraud or unusual behavior from policyholders or third parties involved in a claim. As technology advances and datasets grow bigger, fraud detection algorithms will become even more sophisticated.
4) Real-time pricing: Traditionally, insurance premiums have been set on an annual basis. However, with the use of big data, insurers can move towards a more dynamic pricing model. Real-time data on risk factors such as weather conditions, traffic patterns, and consumer behavior can be used to adjust premiums accordingly. This will not only benefit insurers but also customers who will receive fairer prices based on their actual risk at the time.
5) Predictive analytics:
With the help of predictive analytics, insurers can forecast future trends in the industry and make data-driven decisions about underwriting, pricing, and marketing strategies. This will enable them to stay ahead of the curve and provide better services to their customers.
6) Improved customer experience:
Big data can also be utilized to enhance customer experience by providing timely and personalized services. For example, chatbots powered by machine learning algorithms can handle simple queries and claims processing, freeing up human agents to focus on more complex tasks. Big data analysis can also alert insurers of potential claims before they are filed, leading to faster resolution times for customers.
7) New business models:
The use of big data is expected to open up new business models for insurance companies. With improved risk assessment capabilities and real-time pricing methods, insurers could potentially offer pay-as -you-go or on-demand policies, catering to customers’ changing needs and lifestyles.
Conclusion: Is the Use of Big Data Beneficial for the Insurance Industry?
The use of big data in the insurance industry has proven to be highly beneficial for insurance companies and their customers. Through harnessing vast amounts of data and utilizing advanced analytics, the insurance industry is now able to streamline processes, reduce costs, and provide more personalized policies to consumers.
One of the key advantages of using big data in insurance is its ability to improve risk assessment. Insurance companies can collect and analyze large volumes of data from multiple sources such as social media activity, credit scores, driving habits, and health records. This enables them to gain a more comprehensive understanding of an individual’s risk profile and accurately assess their likelihood of making a claim. As a result, insurers are better equipped to price policies accordingly, ensuring that premiums are fair for both low-risk and high-risk individuals.
Moreover, with the help of big data analytics tools, insurers can also identify fraudulent claims more efficiently. By analyzing patterns in claims data and customer behavior, algorithms can flag suspicious activities and alert fraud investigators. This not only saves insurance companies millions in losses but also helps keep premiums low for honest policyholders.
The implementation of big data has also led to significant improvements in customer experience within the insurance industry. With access to vast amounts of data on consumer preferences, behaviors, and expectations, insurers can now offer personalized policies tailored to each individual’s specific needs. This means that customers no longer have to pay for coverage they do not need or want but instead receive solutions that cater precisely to their requirements.
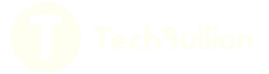