In the world of product development, artificial intelligence (AI) has become an indispensable tool for scaling up operations and improving efficiency. Rajeshkumar Rajubhai Golani, a software engineer, explores how AI technologies are transforming the development process in his recent article. His detailed examination of AI’s integration into product development workflows highlights the innovative approaches reshaping how organizations design, test, and refine their offerings.
The Rise of AI in Product Development
Artificial intelligence is no longer a futuristic concept but a present-day reality driving innovation across industries. Product development, in particular, is undergoing a radical transformation through AI integration. AI’s ability to automate repetitive tasks, personalize user experiences, and provide deep data insights has revolutionized traditional development processes. AI-powered tools such as code generators, design platforms, and testing frameworks have allowed development teams to focus on higher-level strategic challenges while leaving the mundane tasks to intelligent systems.
Building a Strong Data Foundation
The foundation of AI-driven product development is built on robust data collection and management systems. Tools like event-streaming technologies (Apache Kafka and AWS Kinesis) allow real-time capture and analysis of user interactions. With vast volumes of data being generated daily, it is crucial for companies to implement scalable data platforms like Snowflake or Google BigQuery.
Leveraging Machine Learning for Insights
Machine learning (ML) models are at the heart of AI’s capabilities in product development. By utilizing these models, teams can extract actionable insights from large datasets, moving beyond basic analytics to predictive and prescriptive analysis. ML models like XGBoost and LightGBM are used to prioritize features that have the most significant impact on user satisfaction, while deep learning techniques help personalize user experiences by recognizing complex patterns in behavior.
Accelerating Development with Generative AI
Generative AI is playing a pivotal role in streamlining the development process. This innovative technology aids in code generation, UI design, and even testing automation. Tools powered by large language models (LLMs) are now capable of generating code snippets and documentation, reducing the time spent on repetitive tasks. automatically creating interface variations based on user preferences and brand guidelines.
AI-Powered Testing and Performance Analysis
One of the most significant innovations driven by AI is in the field of testing. Traditional testing methods are being replaced by intelligent frameworks capable of autonomously discovering issues and optimizing testing resources. Reinforcement learning systems are particularly useful for exploring application states and discovering edge cases that human testers may overlook. AI is also improving performance testing by processing vast amounts of performance data, enabling teams to identify regressions with greater accuracy and speed.
Personalized User Experiences at Scale
Personalization has become a key focus for many organizations aiming to differentiate their products in the market. AI technologies enable the delivery of tailored experiences to users by analyzing behavior patterns and preferences. Distributed computing frameworks, such as Apache Spark, play a crucial role in processing large user datasets in real-time. By leveraging vector-based recommendation systems, companies can offer personalized content and product suggestions at scale, improving user engagement.
Predicting Trends with AI-Driven Analytics
AI is also transforming the way organizations predict market trends and consumer behavior. Predictive analytics, fueled by ensemble forecasting methods, allows companies to anticipate changes in demand before they occur. By combining multiple forecasting approaches, including machine learning and traditional time series models, businesses can achieve greater accuracy in their predictions.
Overcoming AI’s Challenges
While AI offers tremendous benefits, its implementation does not come without challenges. Issues related to data privacy and algorithmic fairness are at the forefront of discussions on responsible AI use. Differential privacy and homomorphic encryption are two key technologies being explored to address privacy concerns.
A Strategic Approach to AI Integration
Successful integration of AI into product development requires a strategic approach. The implementation process should be incremental, starting with foundational data architectures and progressing to more advanced capabilities like predictive analytics and real-time personalization systems. It is also essential to build cross-functional teams with expertise in machine learning, data science, and domain knowledge to ensure AI solutions are applied effectively.
In conclusion,AI is reshaping how products are developed, offering powerful tools that improve efficiency, personalization, and decision-making. Rajeshkumar Rajubhai Golani’s exploration of AI in product development reveals a clear roadmap for organizations seeking to harness its potential. By adopting AI-driven strategies, companies can scale their development processes, create more personalized user experiences, and stay ahead of market trends. As AI technologies continue to evolve, the organizations that successfully integrate these innovations will be well-positioned for sustained success in an increasingly competitive landscape.
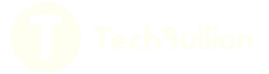