In today’s fast-paced digital world, maintaining the health and performance of complex IT systems is critical for businesses. Pradeep Sambamurthy, a leading expert, explores how artificial intelligence (AI) transforms systems observability, offering advanced capabilities such as anomaly detection, root cause analysis, predictive maintenance, and automated remediation. AI enhances key areas such as anomaly detection, root cause analysis, predictive maintenance, and automated remediation. This innovation is reshaping the landscape of observability, driving greater resilience, reliability, and efficiency across digital infrastructures.
The Need for Enhanced Observability
The growing complexity of IT infrastructures requires a more sophisticated approach to system management. Traditional monitoring tools often fall short in handling the volume of data generated by modern systems. Enterprises, with their large-scale data centers, can produce over 10 terabytes of operational data daily, far exceeding the capabilities of manual analysis.
AI-driven observability addresses the challenges of modern system management by using machine learning to analyze vast amounts of metrics, logs, and traces. This enables organizations to detect patterns and correlations that are often missed by manual methods. As a result, businesses can benefit from improved reliability, faster issue resolution, and a more proactive approach to managing systems. This approach represents not just an evolution but a revolution in how systems are monitored and maintained, providing a comprehensive view of system health and performance and enabling faster, more informed decision-making.
Anomaly Detection: Identifying Issues Before They Escalate
One of AI’s most significant contributions to observability is its ability to detect anomalies in real-time. Unlike traditional systems that rely on pre-defined rules, AI-powered tools continuously learn and adapt, identifying abnormal behavior that may signal potential system failures.
According to IBM Research, enterprises generate over 1.5 petabytes of log data per day, but only 1% of this data is actively analyzed. AI-driven anomaly detection systems can process all of this data, identifying subtle patterns and correlations that human analysts might overlook. Beyond cybersecurity, AI-driven anomaly detection plays a critical role in application performance monitoring (APM).
Root Cause Analysis: Faster Resolutions, Reduced Downtime
AI excels in root cause analysis (RCA), a critical aspect of incident management. Traditional RCA processes, relying on manual analysis, are often slow and prone to errors, especially in complex environments with multiple causes for a single issue. AI, with advanced algorithms, can quickly analyze system metrics, logs, and traces to identify the root cause of problems. A major e-commerce platform reported a 40% reduction in the mean time to resolution (MTTR) after implementing AI-driven RCA. AI’s ability to perform continuous, real-time analysis enables it to detect potential issues before they escalate, making it a game changer compared to traditional post-mortem RCA methods.
Predictive Maintenance: Proactive, Not Reactive
AI’s predictive capabilities allow organizations to shift from reactive to proactive maintenance strategies. By analyzing historical data and identifying patterns that precede system failures, AI can forecast potential issues before they occur, preventing costly downtime and optimizing resource allocation.
According to the IEEE Reliability Society, unplanned downtime costs organizations an average of $260,000 per hour. AI-driven predictive maintenance offers a powerful solution that significantly reduces downtime.
Automated Remediation: The Path to Self-Healing Systems
One of the most exciting developments in AI-driven observability is the integration of automated remediation. These systems detect and diagnose issues and can implement corrective actions autonomously, minimizing downtime and reducing the need for human intervention.
Amazon Web Services (AWS) is a prime example of how AI-driven auto-scaling systems can optimize resource allocation. AWS’s system adjusts compute resources based on real-time demand, reducing over-provisioning by 45% while maintaining a 99.99% service level agreement (SLA) for customers.
Pradeep Sambamurthy highlights that the future of automated remediation lies in cognitive automation—AI systems that learn from past incidents and improve their remediation strategies over time. As these systems evolve, they will drive the development of fully autonomous, self-healing IT infrastructures.
To wrap up, AI-driven systems observability represents a significant leap forward in managing and optimizing digital infrastructures. By harnessing AI’s power for anomaly detection, root cause analysis, predictive maintenance, and automated remediation, organizations can achieve unprecedented levels of system reliability and performance. As this technology continues to evolve, we can expect even more innovative solutions that will transform the way we manage complex IT environments.
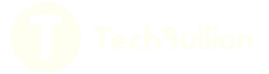