The fourth industrial revolution is here and it means more than just automating the production line. After all, the propelling volume of real-time data generated in the factories must fuel high-grade analytics, make valuable predictions and eventually find solutions to the gravest of inconsistencies on the factory floor. In fact, foreseeing the possible downtimes in the production line and being able to make repairs before it’s too late has strengthened the revolution called Industry 4.0. Momentously, IIoT, (Industrial Internet of Things) is the leading symbol of the emblem of the said era.
Since the adoption of IIoT is anticipated to add USD 1.7 trillion to US GDP by 2030, there’s a lineup of impressive use cases that have affluently embraced the technology.
Take Seebo for example, one of the pioneers to have mastered the craft of process analytics by leveraging AI on the entire process and manufacturing data. With a mission to build smart factories, Seebo is empowering manufacturers in preventing and predicting damage by process-driven AI systems. This means, a robust industrial AI solution comprised of a digital prototype of the production line in operation is designed, proprietary machine learning algorithms and easy to use interface that was designed for the process engineer and operators; that predicts when and why a particular issue could occur and the exact area to rework upon.
With an impressive line-up of industry 4.0 initiatives, they are breaking new grounds with contemporary solutions to age-old complexities in the manufacturing processes. So, be it the incompetency in handling downtimes or controlling the excessive waste, their expertise in implementing AI on manufacturing processes is a pertinent representation of industry 4.0.
Predictive maintenance & fault prediction
Getting maximum value from machines is the crux of a successful factory unit and manufacturing engineers know it well. And data science here can empower them to make insightful comparisons of sensor data with machine failures in the past. What results is a recognizable pattern that can hint at a possible breakdown? In fact, engineers can outline the parts that are most likely to fail or need replacement soon.
If such predictions are acted upon in time, manufacturers could halt the production for a while to perform immediate repairing. Not just considerable delays in the future are avoided but inventories are managed more efficiently.
As per the maintenance survey, the running equipment to the point of failure had dropped from 61% to 57% in setups where predictive maintenance scheduling was combating unplanned downtime.
Today, skillfully using solution modeling & simulation, data fetching through IoT and pushing it forward for deep learning and ultimately data visualization, predictive maintenance techniques can impact the bottom line. This leads to better identification of process bottlenecks, testing alternative units and attain maximized throughput rates. Not to miss, the time-to-repair & cost-to-repair go down with accurate issue reorganization and notifications.
Predictive Waste Reduction
There’s no refusal to the graving concerns about industrial wastes; accounting for 11 billion tones all over the world. Over the decades, factories have been locking horns to contain the waste either by processing it or dumping it appropriately. However, today, when data science is more responsive, process-based machine learning systems have been instrumental in producing lesser waste in the first place. Using machine learning, engineers are able to perform deep quality checks until standards for optimized throughput are achieved.
By identifying specific points of repetitive loss occurrence, the Seebo system gives out timely alerts while reducing production defects and waste alongside end output. When thoroughly analyzed data from historian systems and PLCs are fed to the digital twins, the machine learning algorithms are able to predict peaks in production waste and the deviations (if any) in the regular parameters that normally affect waste. As the root cause of excessive waste levels is redlined, predictive simulation can finally guide about the reworking in the settings required.
Predictive Quality
With Industry 4.0, comes Quality 4.0, an essential function to boost operation excellence, performance and innovation in manufacturing. To be able to foresee and prevent quality blunders in the production line is the newest addition to the bandwagon; combining existing QA expertise with predictive analytics to lessen production costs.
Using an intuitive code-free modeling tool, predictive quality simulation is performed to visually map the production processes. Furthermore, the model is transformed into a digital twin that captures quality metric data such as OEE, first passed yield, reject ratio, etc. Ultimately, quality alerts driven by business rules are used to eliminate uncertainty while accelerating quality issue investigations.
Given such insightful depth, a predictive system does root causes analysis while making actionable predictions and improving machine throughput.
And when quality issues in the machine can be predicted with utmost accuracy, enterprises benefit by minimized scrap and operational costs.
At length, it is the asset utilization and yield that improves, exactly what adheres to. Capturing a holistic view of the entire manufacturing setup, the system helps production engineers, quality managers and production line managers in improving machine efficiency. Unlike most attempts, Seebo has had a triumphant stint at skillfully aligning IoT, AI & simulation in an industrial ecosystem. From predictive maintenance to predictive waste handling, predictive quality and production optimization, their system lets you seek a comprehensive view of the plant on a single dashboard.
Going Ahead – Industry 4.0
This is an exciting decade as enterprise-level adoption of emerging technologies is creating a smarter ecosystem. However, enterprises, especially those in manufacturing, must embrace deploying quality devices (IoT) & software systems (AI) to reap long term benefits from predictive quality.
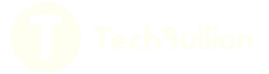