Generative AI is revolutionizing the field of cybersecurity by providing advanced tools for threat detection, analysis, and response, thus significantly enhancing the ability of organizations to safeguard their digital assets. Leveraging powerful models like generative adversarial networks (GANs) and artificial neural networks (ANNs), generative AI has proven effective in identifying cyber threats, including malware, ransomware, and other malicious activities that traditional methods might miss. This technology allows for the automation of routine security tasks, facilitating a more proactive approach to threat management and allowing security professionals to focus on complex challenges. The adaptability and learning capabilities of generative AI make it a valuable asset in the dynamic and ever-evolving cybersecurity landscape [1][2].
Despite its potential, the use of generative AI in cybersecurity is not without challenges and controversies. A significant concern is the dual-use nature of this technology, as cybercriminals can exploit it to develop sophisticated threats, such as phishing scams and deepfakes, thereby amplifying the threat landscape. Additionally, generative AI systems may occasionally produce inaccurate or misleading information, known as hallucinations, which can undermine the reliability of AI-driven security measures. Furthermore, ethical and legal issues, including data privacy and intellectual property rights, remain pressing challenges that require ongoing attention and robust governance [3][4].
The application of generative AI in cybersecurity is further complicated by issues of bias and discrimination, as the models are trained on datasets that may perpetuate existing prejudices. This raises concerns about the fairness and impartiality of AI-generated outputs, particularly in security contexts where accuracy is critical. However, through collaboration between technologists, legal experts, and policymakers, efforts are being made to address these concerns and ensure that generative AI technologies are developed and implemented responsibly, in alignment with ethical standards and legal frameworks [5][6].
Looking ahead, the prospects for generative AI in cybersecurity are promising, with ongoing advancements expected to further enhance threat detection capabilities and automate security operations. Companies and security firms worldwide are investing in this technology to streamline security protocols, improve response times, and bolster their defenses against emerging threats. As the field continues to evolve, it will be crucial to balance the transformative potential of generative AI with appropriate oversight and regulation to mitigate risks and maximize its benefits [7][8].
Historical Background
The concept of utilizing artificial intelligence in cybersecurity has evolved significantly over the years. One of the earliest types of neural networks, the perceptron, was created by Frank Rosenblatt in 1958, setting the stage for the development of more advanced AI systems like feedforward neural networks or multi-layer perceptrons (MLPs)[1]. With the advent of generative AI, the landscape of cybersecurity has transformed dramatically. Generative AI, particularly models such as ChatGPT that use large-scale language models (LLM), has introduced a new dimension to cybersecurity due to its high degree of versatility and potential impact across the cybersecurity field[2]. This technology has brought both opportunities and challenges, as it enhances the ability to detect and neutralize cyber threats while also posing risks if exploited by cybercriminals [3]. The dual nature of generative AI in cybersecurity underscores the need for careful implementation and regulation to harness its benefits while mitigating potential drawbacks[4] [5].
Generative AI Technologies
Generative AI technologies are transforming the field of cybersecurity by providing sophisticated tools for threat detection and analysis. These technologies often rely on models such as generative adversarial networks (GANs) and artificial neural networks (ANNs), which have shown considerable success in identifying and responding to cyber threats.
Artificial Neural Networks (ANNs)
ANNs are widely used machine learning methods that have been particularly effective in detecting malware and other cybersecurity threats. The backpropagation algorithm is the most frequent learning technique employed for supervised learning with ANNs, allowing the model to improve its accuracy over time by adjusting weights based on error rates[6]. However, implementing ANNs in intrusion detection does present certain challenges, though performance can be enhanced with continued research and development [7].
Generative Adversarial Networks (GANs)
GANs play a crucial role in simulating cyberattacks and defensive strategies, thus providing a dynamic approach to cybersecurity [3]. By producing new data instances that resemble real-world datasets, GANs enable cybersecurity systems to rapidly adapt to emerging threats. This adaptability is crucial for identifying subtle patterns of malicious activity that might evade traditional detection methods [3]. GANs are also being leveraged for asymmetric cryptographic functions within the Internet of Things (IoT), enhancing the security and privacy of these networks[8].
Federated Deep Learning
The integration of federated deep learning in cybersecurity offers improved security and privacy measures by detecting cybersecurity attacks and reducing data leakage risks. Combining federated learning with blockchain technology further reinforces security control over stored and shared data in IoT networks[8].
Natural Language Processing and Analysis
Generative AI technologies utilizing natural language processing (NLP) allow analysts to ask complex questions regarding threats and adversary behavior, returning rapid and accurate responses[4]. These AI models, such as those hosted on platforms like Google Cloud AI, provide natural language summaries and insights, offering recommended actions against detected threats[4]. This capability is critical, given the sophisticated nature of threats posed by malicious actors who use AI with increasing speed and scale[4].
These advanced technologies demonstrate the powerful potential of generative AI to not only enhance existing cybersecurity measures but also to adapt to and anticipate the evolving landscape of cyber threats.
Applications of Generative AI in Cyber Security
Generative AI has emerged as a pivotal tool in enhancing cyber security strategies, enabling more efficient and proactive threat detection and response mechanisms. As the shortage of advanced security personnel becomes a global issue, the use of generative AI in security operations is becoming essential. For instance, generative AI aids in the automatic generation of investigation queries during threat hunting and reduces false positives in security incident detection, thereby assisting security operations center (SOC) analysts[2].
Threat Detection and Incident Response
In the realm of threat detection, generative AI models are capable of identifying patterns indicative of cyber threats such as malware, ransomware, or unusual network traffic, which might otherwise evade traditional detection systems [3]. By continuously learning from data, these models adapt to new and evolving threats, ensuring detection mechanisms are steps ahead of potential attackers. This proactive approach not only mitigates the risks of breaches but also minimizes their impact. For security event and incident management (SIEM), generative AI enhances data analysis and anomaly detection by learning from historical security data and establishing a baseline of normal network behavior [3].
Enhancing Intrusion Detection Systems
In a novel approach to cyber threat-hunting, the combination of generative adversarial networks and Transformer-based models is used to identify and avert attacks in real time. This methodology is particularly effective in intrusion detection systems (IDS), especially in the rapidly growing IoT landscape, where efficient mitigation of cyber threats is crucial[8].
Mitigating Malicious Uses of AI
While generative AI offers robust tools for cyber defense, it also presents new challenges as cybercriminals exploit these technologies for malicious purposes. For instance, adversaries use generative AI to create sophisticated threats at scale, identify vulnerabilities, and bypass security protocols. Notably, social engineers employ generative AI to craft convincing phishing scams and deepfakes, thus amplifying the threat landscape[4]. Despite these risks, generative AI provides significant opportunities to fortify cybersecurity defenses by aiding in the identification of potential attack vectors and automatically responding to security incidents[4].
Industry Applications
Security firms worldwide have successfully implemented generative AI to create effective cybersecurity strategies. An example is SentinelOne’s AI platform, Purple AI, which synthesizes threat intelligence and contextual insights to simplify complex investigation procedures[9]. Such applications underscore the transformative potential of generative AI in modern cyber defense strategies, providing both new challenges and opportunities for security professionals to address the evolving threat landscape.
Advantages of Using Generative AI
Generative AI offers significant advantages in the realm of cybersecurity, primarily due to its capability to rapidly process and analyze vast amounts of data, thereby speeding up incident response times. Elie Bursztein from Google and DeepMind highlighted that generative AI could potentially model incidents or produce near real-time incident reports, drastically improving response rates to cyber threats[4]. This efficiency allows organizations to detect threats with the same speed and sophistication as the attackers, ultimately enhancing their security posture[4].
Moreover, generative AI’s ability to simulate various scenarios is critical in developing robust defenses against both known and emerging threats. By automating routine security tasks, it frees cybersecurity teams to tackle more complex challenges, optimizing resource allocation [3]. Generative AI also provides advanced training environments by offering realistic and dynamic scenarios, which enhance the decision-making skills of IT security professionals [3].
The adaptability of generative AI is another crucial advantage. As it continuously learns from data, it evolves to meet new threats, ensuring that detection mechanisms stay ahead of potential attackers [3]. This proactive approach significantly reduces the risk of breaches and minimizes the impact of those that do occur, providing detailed insights into threat vectors and attack strategies [3].
In a broader context, generative AI can enhance resource management within organizations. Over half of executives believe that generative AI aids in better allocation of resources, capacity, talent, or skills, which is essential for maintaining robust cybersecurity operations[4]. Despite its powerful capabilities, it’s crucial to employ generative AI to augment, rather than replace, human oversight, ensuring that its deployment aligns with ethical standards and company values [5].
Challenges and Limitations
Generative AI, while offering promising capabilities for enhancing cybersecurity, also presents several challenges and limitations. One major issue is the potential for these systems to produce inaccurate or misleading information, a phenomenon known as hallucinations[2]. This not only undermines the reliability of AI-generated content but also poses significant risks when such content is used for critical security applications.
The legal and ethical challenges associated with generative AI are substantial. The current legal frameworks addressing intellectual property rights and privacy concerns have significant gaps and limitations, making it difficult to tackle issues specific to generative AI, such as the origin of AI-generated content and jurisdictional complexities[10]. Furthermore, companies face ethical obligations when implementing generative AI, which require them to balance the potential benefits against cybersecurity risks[11].
There are also concerns regarding bias and discrimination embedded in generative AI systems. The data used to train these models can perpetuate existing biases, raising questions about the trustworthiness and interpretability of the outputs [5]. This is particularly problematic in cybersecurity, where impartiality and accuracy are paramount.
Moreover, generative AI technologies can be exploited by cybercriminals to create sophisticated threats, such as malware and phishing scams, at an unprecedented scale[4]. The same capabilities that enhance threat detection can be reversed by adversaries to identify and exploit vulnerabilities in security systems [3]. As these AI models become more sophisticated, the potential for misuse by malicious actors increases, further complicating the security landscape.
Addressing these challenges requires proactive measures, including AI ethics reviews and robust data governance policies[12]. Collaboration between technologists, legal experts, and policymakers is essential to develop effective legal and ethical frameworks that can keep pace with the rapid advancements in AI technology[12].
Case Studies
Generative AI has been increasingly applied to address various cybersecurity challenges, with several case studies highlighting its potential and limitations. One significant area of application is in threat intelligence, where generative AI tools are employed to detect and counteract complex cyber threats like phishing and malware. For instance, IBM’s Generative AI: Boost Your Cybersecurity Career course on Coursera delves into how these advanced AI tools are used in real-world scenarios to enhance cybersecurity measures against such threats[13].
Another case study focuses on the integration of generative AI into cybersecurity frameworks to improve the identification and prevention of cyber intrusions. This approach often involves the use of neural networks and supervised learning techniques, which are essential for training algorithms to recognize patterns indicative of cyber threats. However, the application of neural networks also introduces challenges, such as the need for explainability and control over algorithmic decisions[14][1].
Moreover, a thematic analysis based on the NIST cybersecurity framework has been conducted to classify AI use cases, demonstrating the diverse applications of AI in cybersecurity contexts[15]. This classification not only provides a comprehensive understanding of AI’s potential in cybersecurity but also identifies areas for future research, such as the development of new infrastructures and advanced AI methods for successful adoption in the face of digital transformation and polycrisis[15].
While these case studies showcase the promising applications of generative AI in combating cybersecurity threats, they also underscore the importance of addressing associated ethical, legal, and societal challenges. These include potential biases in AI models and the inadvertent disclosure of sensitive information, which could undermine trust and result in legal consequences [5][16]. Therefore, companies are advised to implement clear guidelines and governance structures to ensure the responsible use of generative AI in cybersecurity initiatives [5].
Future Prospects
The future of generative AI in combating cybersecurity threats looks promising due to its potential to revolutionize threat detection and response mechanisms. As organizations continue to leverage deep learning models, generative AI is expected to enhance the simulation of advanced attack scenarios, which is crucial for testing and fortifying security systems against both known and emerging threats [3]. This technology not only aids in identifying and neutralizing cyber threats more efficiently but also automates routine security tasks, allowing cybersecurity professionals to concentrate on more complex challenges [3].
Looking forward, generative AI’s ability to streamline security protocols and its role in training through realistic and dynamic scenarios will continue to improve decision-making skills among IT security professionals [3]. Companies like IBM are already investing in this technology, with plans to release generative AI security capabilities that automate manual tasks, optimize security teams’ time, and improve overall performance and effectiveness[4]. These advancements include creating simple summaries of security incidents, enhancing threat intelligence capabilities, and automatically responding to security threats[4].
However, the deployment of generative AI in cybersecurity is not without challenges. The potential for legal and ethical issues, especially concerning data use and model outputs, must be addressed[16][10]. It is essential for legal frameworks to adapt continuously to these technological advancements, ensuring that AI is governed responsibly while remaining transformational[12]. A comprehensive approach, including a clearly defined strategy and good governance, will be necessary to manage the risks associated with generative AI and to support a corporate culture that embraces AI ethics [5].
References
[1] IBM. (n.d.). What is a neural network?. IBM. https://www.ibm.com/think/topics/neural-networks
[2] Sekine, T. (n.d.). Security risks of generative AI and countermeasures. NTT DATA. https://www.nttdata.com/global/en/insights/focus/security-risks-of-generative-ai-and-countermeasures
[3] Palo Alto Networks. (n.d.). What is generative AI in cybersecurity?. https://www.paloaltonetworks.com/cyberpedia/generative-ai-in-cybersecurity
[4] Fitzgerald, A. (2024, May 15). How can generative AI be used in cybersecurity? 10 real-world examples. Secureframe. https://secureframe.com/blog/generative-ai-cybersecurity
[5] Lawton, G. (2024, July 23). Generative AI ethics: 8 biggest concerns and risks. TechTarget. https://www.techtarget.com/searchenterpriseai/tip/Generative-AI-ethics-8-biggest-concerns
[6] Khraisat, A., Gondal, I., Vamplew, P., & Kamruzzaman, J. (2019). Survey of intrusion detection systems: techniques, datasets and challenges. Cybersecurity, 2(1), 20. https://doi.org/10.1186/s42400-019-0038-7
[7] Pawlicki, M., Kozik, R., & Choraś, M. (2022). A survey on neural networks for (cyber-) security and (cyber-) security of neural networks. Neurocomputing, 500, 1075–1087. https://doi.org/10.1016/j.neucom.2022.06.016
[8] Arifin, M. M., Ahmed, M. S., Ghosh, T. K., Zhuang, J., & Yeh, J. (2024). A survey on the application of generative adversarial networks in cybersecurity: Prospective, direction and open research scopes. arXiv. https://arxiv.org/abs/2407.08839
[9] SentinelOne. (2024, June 25). What is generative AI in cybersecurity? https://www.sentinelone.com/cybersecurity-101/data-and-ai/generative-ai-cybersecurity/
[10] Yoong, G. S. (2023, July 28). Intersection of generative AI, cybersecurity and digital trust. TechTarget. https://www.techtarget.com/searchsecurity/post/Intersection-of-generative-AI-cybersecurity-and-digital-trust
[11] Humphreys, D., Koay, A., Desmond, D., & Mealy, E. (2024). AI hype as a cyber security risk: the moral responsibility of implementing generative AI in business. AI and Ethics, 4, 791–804. https://doi.org/10.1007/s43681-024-00443-4
[12] Epilogue Systems. (n.d.). 5 key AI legal challenges in the era of generative AI. https://epiloguesystems.com/blog/5-key-ai-legal-challenges/
[13] Ticong, L. (2024, October 21). How can generative AI be used in cybersecurity? (Ultimate guide). eWeek. https://www.eweek.com/artificial-intelligence/generative-ai-and-cybersecurity/
[14] Copado Team. (2021, September 15). Data security using neural networks can provide additional security layers. Copado. https://www.copado.com/resources/blog/data-security-using-neural-networks-can-provide-additional-security-layers
[15] Kaur, R., Gabrijelčič, D., & Klobučar, T. (2023). Artificial intelligence for cybersecurity: Literature review and future research directions. Information Fusion, 97, 101804. https://doi.org/10.1016/j.inffus.2023.101804
[16] Walsh, D. (2023, August 28). The legal issues presented by generative AI. MIT Sloan. https://mitsloan.mit.edu/ideas-made-to-matter/legal-issues-presented-generative-ai
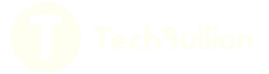