Madiha Shakil Mirza is an Artificial Intelligence Engineer at Avanade, a global technology consulting firm offering digital, cloud, AI, and advisory services. As an Artificial Intelligence Engineer, she specializes in helping Avanade’s clients build their organizational capabilities for AI.
Madiha earned her Bachelor’s and Master’s degrees from the University of Minnesota. As a graduate student in the Department of Computer Science at the University of Minnesota, Madiha collaborated with her thesis advisor on Generative AI and Natural Language Processing research.
For over seven years, Madiha has applied her expertise in Artificial Intelligence, Generative AI, and Natural Language Processing on various projects specific to the healthcare sector. She has combined her formal education and research background with her industry experience for healthcare modernization and innovation.
TechBullion spoke with Madiha about how new HealthTech tools are revolutionizing patient care, diagnostics, and clinical workflows, and how she is using her experiences at the intersection of technology and healthcare to drive the next generation of healthcare innovation.
Madiha Shakil Mirza
Q: Madiha, tell us about your background. Your academic training was in Computer Science, with research focus on AI, GenAI, and Natural Language Processing (NLP), and you began your professional career as an Analyst, Artificial Intelligence at Avanade. What led you to develop HealthTech expertise?
A: I have a background in Computer Science with a specialization in AI, Natural Language Processing (NLP), and Generative AI. The research experience which I gained during my graduate studies is what inspired me to continue working in this field professionally. I have over seven years of industry experience applying AI to solve real-world problems. For the past several years, I’ve focused specifically on healthcare, working on projects ranging from clinical prediction and clinical virtual assistants to intelligent search chatbots. My work bridges technical development and strategic deployment, ensuring AI solutions are not only accessible but also explainable, compliant, and usable in real clinical environments. My passion lies in using AI responsibly to solve some of healthcare’s most complex challenges.
Q: What healthcare-specific challenges have you encountered when building AI models?
A: Working in healthcare AI involves navigating a unique set of challenges that go far beyond typical machine learning problems. One of the biggest issues is data quality and interoperability. Clinical data often comes from multiple siloed systems with inconsistent formats, incomplete records, and different coding standards. Cleaning and standardizing this data is time-consuming but essential for building reliable models.
Another major challenge is the lack of labeled data. Ground truth in healthcare can be hard to define and diagnoses may be delayed, subjective, or vary across institutions. Additionally, data imbalance is common, especially when dealing with rare diseases or edge cases, making it difficult to train strong models without introducing bias.
Healthcare also demands extremely high standards for safety, interpretability, and regulatory compliance. Unlike other industries, a false positive or negative prediction could lead to real harm. That’s why explainability and transparency are non-negotiable. AI tools must be understandable to clinicians, auditable for regulators, and proven to do no harm across diverse patient populations.
There’s also the challenge of clinical workflow integration. Even the best model can fail in practice if it’s not embedded in a way that supports, rather than disrupts, the provider’s routine. Understanding how clinicians make decisions and designing AI tools that complement rather than replace them is essential for adoption.
Finally, ethical concerns and equity are critical. AI can unintentionally perpetuate health disparities if it’s trained on biased datasets or doesn’t account for underrepresented populations. It’s vital to continuously audit models for fairness and build safeguards that ensure equitable access and outcomes for all patients.
Q: How do you address bias and fairness in healthcare AI models?
A: Bias and fairness are critical considerations in healthcare AI because the stakes are high as unfair models can reinforce disparities and negatively impact patient care. My approach starts with understanding the clinical and social context of the data. During model development, I apply fairness-aware techniques such as re-weighting, stratified sampling, or adversarial debiasing to mitigate known biases. I also incorporate subgroup performance evaluation as a standard part of model validation. It’s not enough for a model to perform well overall as it must perform equitably across diverse patient populations. Finally, transparency is key. I prioritize interpretable models and when using complex architectures, I pair them with explainability tools like SHAP or counterfactual analysis. This helps build trust among users and allows clinicians to understand how the model reaches its conclusions, especially important when decisions affect patient care.
Q: What are the challenges of working with unstructured healthcare data, and how do you tackle them?
A: Unstructured data in healthcare, such as clinical notes, discharge summaries, radiology reports, and even patient messages, presents both a rich source of insight and a significant challenge. The complexity arises from inconsistent formats, medical jargon, abbreviations, and the variability in how clinicians document information.
One of the biggest challenges is contextual ambiguity. For example, “no history of diabetes” and “family history of diabetes” might look similar to a naive model but have very different clinical meanings. This is addressed using domain-specific NLP models, such as BioBERT or ClinicalBERT, which are pre-trained on medical corpora and better understand clinical language. Issues with negation detection, temporal expressions, and entity disambiguation are solved using NLP-based techniques, custom rule-based filters, or transformer-based models trained for named entity recognition and relation extraction. Another hurdle is data quality and labeling. Unstructured text often lacks ground truth labels, so a combination of clinician-validated annotation or semi-supervised learning is used to generate training data efficiently.
Q: As someone with deep healthcare AI expertise, how do you approach the deployment of HealthTech solutions?
A: Successful deployment of HealthTech solutions requires far more than just technical performance. It’s about ensuring clinical relevance, regulatory compliance, and seamless integration into real-world workflows. My approach begins with co-designing the solution alongside clinicians and end users. Their insights are essential to define the right problem, evaluate usability, and identify where AI can truly augment care rather than disrupt it. From a systems perspective, I ensure that models are integrated into existing infrastructure by working closely with compliance teams to address data privacy (HIPAA/GDPR), auditability, and version control from day one. Finally, I treat deployment as the beginning, not the end. I set up monitoring pipelines to track model drift, user engagement, and real-world outcomes. The goal is to create solutions that are not just innovative, but safe, trusted, and sustainable in the complex, high-stakes environment of healthcare.
Q: How do you stay current with research and development in both AI and healthcare?
A: Staying at the forefront of both AI and healthcare requires continuous learning across two fast-evolving domains. I actively follow top-tier Artificial Intelligence in Healthcare conferences for the latest in AI methodologies, and I also track journals and conferences in medical informatics and digital health for clinical applications. I also spend a lot of time on hands-on exploration by experimenting with new models, open datasets, and toolkits to stay sharp with the latest tools and technologies.
Q: What advice would you give to someone interested in pursuing a career in AI, particularly in healthcare?
A: Start by grounding yourself in both the technical foundations of AI, Machine Learning, and Statistics and the domain knowledge of healthcare. Understanding how the healthcare system works, common healthcare data types, and the clinical relevance of your models is essential. My biggest advice is to build with empathy. In healthcare, lives are at stake. Always ask how your model will be used, who it might leave out, and whether it will actually help someone. The best AI engineers in this space are not just innovative but also empathetic and responsible.
Q: What role do you see Generative AI playing in the future of healthcare?
A: Generative AI has the potential to transform healthcare in both clinical and operational settings. One of the most immediate applications is in clinical documentation, the automatic generation and summarization of notes, discharge instructions, or referral letters, which can significantly reduce clinician burnout and improve data quality. In patient-facing applications, Generative AI can power intelligent health assistants that provide personalized, conversational guidance on symptoms, medications, or care navigation making healthcare more accessible and reducing load on front-line staff. When paired with trusted guardrails, these tools can offer safe and context-aware interactions. On the research side, Generative models are being used to synthesize realistic but de-identified patient data for training, testing, or simulation, which can accelerate innovation while preserving privacy. They also show promise in drug discovery, where models generate candidate molecules or predict protein structures. In radiology and imaging, Generative AI is helping with data augmentation, reconstruction, and even generating synthetic scans for rare conditions, supporting more robust model development and diagnostics. I believe the future lies in collaborative intelligence, where generative AI becomes an invisible co-pilot in the healthcare journey, enhancing but not replacing the human touch.
Q: What is one AI tool or technology you’re currently excited about?
A: I’m excited about the advancements in large language models fine-tuned for clinical use and other domain-specific versions of foundational models. These tools are beginning to understand and generate medical language with a degree of nuance we haven’t seen before. When combined with retrieval-based architectures and clinical validation, they have the potential to power decision support, patient communication, and summarization in truly impactful ways. What excites me most is their ability to bridge the gap between structured data and human-centered care by bringing us closer to making AI a trusted partner in the clinical workflow, not just a backend tool.
Q: How do you see the future of AI evolving in healthcare?
A: The future of AI in healthcare is incredibly promising and will be defined by more intelligent, personalized, and collaborative systems. I see three major trends shaping this evolution.
First, AI will become more integrated into the clinical decision-making process, not as a replacement for human expertise, but as an intelligent assistant that augments it. We’re moving toward a future where AI tools provide real-time, evidence-based insights during patient encounters, whether it’s helping a radiologist detect anomalies in an image or suggesting next best actions based on a patient’s longitudinal health record.
Second, we’ll see a shift from narrow models trained for specific tasks to more generalizable and multimodal AI systems. With the rise of foundation models and advances in LLMs, there’s potential for AI to understand and reason across multiple data types, such as structured EHR data, imaging, genomics, and even clinician notes, within a single architecture. This opens the door to richer clinical insights and whole-patient modeling that mirrors how providers think holistically.
Third, AI will play a critical role in enabling personalized and preventive care. Instead of reacting to illness, health systems will leverage predictive models to proactively intervene. AI can help identify high-risk patients early, suggest tailored interventions, and track treatment effectiveness in real-time. This shift will support better health outcomes, reduced costs, and more empowered patients.
Of course, this future also comes with responsibility. As AI becomes more powerful, we must ensure its use is ethical, equitable, and transparent. That includes rigorous validation, bias auditing, patient consent frameworks, and strong model governance.
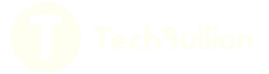