In his latest work, Shivendra Kumar, a forecasting expert with extensive experience in applied analytics, proposes a bold shift in how organizations evaluate predictions. With a career rooted in industry application, He highlights why strategy must take precedence over precision.
Rethinking Forecasting: Why Accuracy Isn’t Enough
Traditional forecasting methods have long emphasized accuracy metrics such as MAPE or RMSE. While these statistical tools help quantify error, they often fall short of supporting real business needs. He argues that overly focusing on error minimization may paradoxically hinder growth, especially in fast-moving or ambitious business environments. Instead, strategic alignment should be the guiding principle when evaluating forecasts.
Embracing Intentional Bias
One of the most provocative ideas presented is the strategic use of intentional bias. While bias has historically been viewed as a forecasting flaw, it can serve as a catalyst for performance in the right context. By intentionally over-forecasting in growth-driven sectors, organizations create stretch targets that inspire action. The key lies in understanding when bias promotes positive behavior versus when it masks underlying issues. Rather than eliminating bias, businesses should harness it intentionally and contextually.
Differentiating Forecasts from Plans
He stresses a crucial conceptual divide: forecasts predict likely outcomes based on data, while plans reflect organizational intentions and strategic direction. Mixing the two can lead to confusion, diluted accountability, and misaligned goals. Successful organizations treat forecasts and plans as separate entities—reconciling them through structured review cycles and using their differences to spark strategic discussion. The synergy lies in treating forecasts as input to planning, not as its justification.
Strategic Use of Forecast Errors
Another innovation highlighted is the reframing of forecast errors as learning opportunities. Rather than seeing deviations as failures, encourages companies to analyze why forecasts were off and what those gaps reveal about their environments. In fact, consistent over- or under-forecasting can surface inefficiencies or emerging trends that would remain hidden under an accuracy-only mindset. This transformation turns variance analysis into a continuous improvement mechanism, rather than a blame game.
Forecasts as Organizational Glue
Forecasts, when designed strategically, serve more than just predictive functions—they align departments, allocate resources, and establish shared benchmarks.draws attention to the ability of forecasts to coordinate action across teams, making them a vital communication tool. An accurate forecast that fails to spark the right decisions is ultimately less valuable than a biased one that drives effective action.
Evolving Evaluation Frameworks
He advocates for multi-dimensional evaluation frameworks tailored to different business units and objectives. For instance, a stable product line may need forecasts with minimal variance, while a new initiative may benefit more from stretch targets. Customizing metrics based on context improves responsiveness and decision-making. Additionally, companies that formalize variance review protocols and maintain distinct performance indicators for forecast quality versus planning success are better positioned to adapt and grow.
Integrating Quantitative Models with Human Judgment
Forecasting is often seen as a data science exercise, but pushes for the integration of statistical models with expert judgment. Quantitative methods excel at pattern recognition, but they struggle with unprecedented situations or qualitative insights. Human judgment, though prone to bias, can add contextual richness. The key is a structured integration—using data-driven models as a foundation, then refining them with domain expertise.
Feedback Loops as Engines of Forecasting Maturity
Establishing robust feedback loops is another pillar of his framework. By consistently comparing predicted versus actual outcomes, and reviewing the rationale behind adjustments, organizations can identify patterns, correct systematic errors, and build institutional memory. This practice supports both model refinement and better decision-making, evolving forecasting from a periodic task to an ongoing organizational learning process.
Tailoring Metrics to Strategic Objectives
Finally, underscores the importance of aligning forecasting metrics with the organization’s strategic goals. A one-size-fits-all evaluation metric ignores the nuanced trade-offs in different functions.
In conclusion, By shifting the lens of evaluation from accuracy to strategic utility, Shivendra Kumar offers a compelling roadmap for modernizing forecasting practices. His work suggests that the most valuable forecasts are those that drive alignment, action, and learning—beyond simply matching predictions to outcomes. In today’s complex business landscape, this forward-thinking approach positions forecasting as a vital contributor to sustainable success.
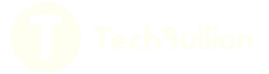