In the ever-evolving landscape of AI/ML solutions, the potential applications for organizational forecasting have become increasingly apparent. Karthika Gopalakrishnan, a seasoned professional in the field, has been at the forefront of developing a forecasting engine aimed at predicting cash inflows and outflows for organizations. Her work has not only highlighted the significance of data integrity and adaptability but has also underscored the critical importance of effective communication with business stakeholders.
Unveiling the Challenge
In a world where data is abundant but not always conducive to AI/ML applications, Gopalakrishnan and her team recognized the pressing need for a robust forecasting engine. They understood that while organizations have been amassing substantial volumes of data related to cash flows, the real challenge lay in discerning its value for accurate future forecasting. The availability and integrity of this data emerged as a major hurdle in the development of an AI/ML solution.
Iterative Design and Resilience
Gopalakrishnan’s journey was not devoid of obstacles. The initial attempts at developing the solution encountered setbacks, leading the team to realize that a singular model was not universally compatible with the diverse array of available data. This realization prompted them to devise a system of models, with the selection of the most suitable model contingent upon specific metrics. Moreover, they encountered the issue of outliers skewing results, prompting them to innovate methods to handle outliers without compromising the overall forecast accuracy.
The Power of Iterative Collaboration
A pivotal aspect of Gopalakrishnan’s approach was the continuous engagement with business stakeholders. By immersing themselves in extensive discussions to comprehend the intricacies of cash flows and business requirements, her team molded the forecasting engine to seamlessly align with the organization’s needs. This iterative collaboration not only expedited the learning process but also facilitated the creation of more effective solutions.
Educating and Adapting
Amid the surging trend of AI/ML, Gopalakrishnan recognized the imperative to bridge the gap between traditional business approaches and cutting-edge models. She emphasized the need to educate business operations and management about the inherent biases in models and the possibility of varying prediction accuracies. This proactive communication and education were pivotal in fostering trust and synergy between the technical team and the business stakeholders.
Data-Centric Approach and Technical Adaptability
Gopalakrishnan’s work underlines the paramount importance of comprehensively understanding the data before embarking on an ML-based solution. She underscored the necessity of tailoring the solution to meet diverse business requirements, ranging from decision-critical predictions to scenarios where minor errors are permissible. Additionally, her team confronted the challenge of scaling the solution across different types of clients and data formats, emphasizing the significance of rigorous data preprocessing and extensive testing.
Conclusion
The development of Karthika Gopalakrishnan’s cash forecasting engine serves as a testament to the intricate interplay between technical acumen, adaptability, and effective stakeholder engagement. Her invaluable insights and experiences not only shed light on the complexities inherent in AI/ML-driven forecasting but also offer indispensable lessons for professionals navigating similar terrain. As organizations continue to harness the potential of AI/ML solutions, Gopalakrishnan’s work stands as a beacon of guidance, advocating for a holistic approach that amalgamates technical prowess with unwavering collaboration and understanding of business intricacies.
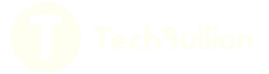