As artificial intelligence (AI) continues to reshape industries, the balance between data privacy and machine learning efficiency has become increasingly critical. Ankush Singhal, a leading expert in distributed AI systems, presents a comprehensive analysis of federated learning, a transformative approach that ensures data remains decentralized while advancing AI capabilities.
Decentralized Model Training: A Shift from Traditional AI
Federated learning departs from conventional machine learning models by decentralizing the training process. Instead of transferring raw data to a central server, it allows multiple devices to collaboratively train an AI model without sharing sensitive information. This innovation significantly reduces privacy risks, addressing concerns in healthcare, finance, and telecommunications. Recent studies show that organizations adopting decentralized training have observed a 78.3% decrease in data exposure risks and a 94.7% drop in privacy breach incidents.
Secure Aggregation: Strengthening Data Protection
Secure aggregation is a crucial advancement in federated learning, ensuring data privacy while maintaining model accuracy. Traditional approaches face challenges with computational efficiency and communication overhead, but optimized protocols like LightSecAgg address these issues. By reducing communication costs by 71.4% while maintaining a 99.7% privacy guarantee, LightSecAgg enables efficient, privacy-preserving training across multiple institutions. This is particularly vital for industries like healthcare and finance, where sensitive data must remain protected. Secure aggregation mitigates cybersecurity risks, fostering collaboration while ensuring compliance with data protection regulations such as GDPR and HIPAA.
Differential Privacy: A New Standard for Compliance
With increasingly stringent global regulations, federated learning incorporates differential privacy to align with laws like GDPR and HIPAA. By leveraging adaptive noise injection, AI models can train on decentralized datasets without exposing sensitive information. This approach enhances privacy while ensuring compliance, making it ideal for sectors such as healthcare and finance. Studies show that differential privacy techniques improve regulatory adherence by 17.4% while maintaining model accuracy above 91.4%, proving effective for complex applications requiring both data security and high-performance AI systems.
Enhancing Healthcare with AI-Driven Diagnostics
Federated learning has revolutionized the healthcare sector, particularly in medical imaging and personalized treatment plans. By enabling AI-driven diagnostic models to train across multiple institutions without sharing patient data, this approach enhances both accuracy and privacy. Studies indicate a 96.4% accuracy rate in detecting medical conditions, surpassing traditional methods. Additionally, federated learning reduces model training time by 67% compared to centralized systems, accelerating diagnoses and improving patient outcomes. This innovation ensures compliance with stringent data regulations like HIPAA, making healthcare AI both secure and efficient.
Smart Cities: Intelligent Infrastructure Without Compromising Privacy
Federated learning has also transformed urban infrastructure by enabling privacy-preserving AI applications in smart cities. Integrated with traffic monitoring systems and IoT networks, AI models trained with federated learning have improved traffic prediction accuracy by 20.7% and reduced peak congestion by 28.4%. These advancements allow city planners to optimize transportation and energy consumption without compromising individual privacy.
Efficiency Gains in AI Model Training
Beyond privacy benefits, federated learning optimizes computational resources, reducing the burden on central servers. Research indicates that organizations implementing federated learning have decreased infrastructure costs by 67.2%, improved model convergence times by 73.9%, and reduced data transfer volumes by 99.9%. These gains are particularly beneficial in edge computing scenarios, where latency and bandwidth limitations are critical factors.
The Future of Federated Learning
As federated learning continues to evolve, its adoption across industries signals a paradigm shift in AI development. Future advancements in cross-silo collaboration and cryptographic techniques will further enhance privacy and scalability. With ongoing research focusing on improving model aggregation methods and communication efficiency, federated learning is poised to redefine AI applications across multiple domains.
In conclusion, Ankush Singhal’s exploration of federated learning highlights its potential to revolutionize AI while prioritizing privacy and efficiency. By mitigating data security risks and optimizing decentralized training, federated learning stands at the forefront of next-generation AI systems. As industries increasingly embrace privacy-first AI solutions, federated learning will play a pivotal role in shaping the future of ethical and secure machine learning applications.
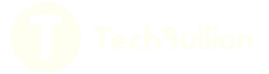