In this modern era, Reinforcement Learning (RL) has evolved from theoretical research to a transformative force driving significant changes in industrial applications. Debu Sinha, a recognized authority in machine learning and reinforcement learning, has led multiple real-world deployments of RL systems across industries, helping enterprises transition RL from theory to production. This article dives into the key innovations in RL, highlighting the groundbreaking techniques and their expanding impact on various industries. These innovations are paving the way for smarter systems across sectors, enhancing both efficiency and decision-making.
The Evolution of Reinforcement Learning: From Theory to Practice
Reinforcement Learning (RL) has historically struggled with issues such as sample inefficiency and safety, especially in complex, real-world environments. However, recent innovations have revolutionized how RL is applied, allowing systems to tackle increasingly complicated tasks. This transition from academic exploration to industrial adoption has led to the rise of more effective RL strategies like hierarchical reinforcement learning (HRL), offline learning systems, and model-based approaches. These advancements are empowering industries to implement RL solutions that are both scalable and adaptable to diverse applications.
Hierarchical Reinforcement Learning: Simplifying Complex Tasks
Hierarchical reinforcement learning (HRL) addresses the challenge of learning complex tasks by decomposing them into simpler subtasks. This approach allows RL systems to learn faster and more efficiently, making it possible to handle tasks that would otherwise be computationally infeasible. Techniques such as Meta-Learning Shared Hierarchies (MLSH) improve learning rates by up to 10 times compared to traditional methods, particularly in environments with sparse rewards. HRL is especially valuable in real-world applications where efficiency and speed are critical.
Offline Reinforcement Learning: Learning from the Past
Offline reinforcement learning allows systems to learn from historical data instead of requiring real-time interaction with the environment. This is useful where real-time exploration is costly. Offline learning systems, when combined with hierarchical structures, enhance sample efficiency and stabilize learning. By leveraging past experiences, these systems reduce the need for extensive data collection, making them more practical for complex environments. Learning from historical data enables faster adaptation and better decision-making in dynamic scenarios.
Model-Based Reinforcement Learning: Enhancing Sample Efficiency
Model-based reinforcement learning has made substantial strides in improving sample efficiency and robustness. These approaches reduce the number of interactions needed to achieve high performance, making them suitable for applications where data collection is expensive or time-consuming. By simulating the environment internally, model-based methods allow for faster learning and better planning. This makes them particularly effective in situations where real-time feedback is limited or difficult to obtain.
Real-World Impact: Transforming Industries Through RL
The implementation of RL across various sectors has led to measurable improvements in efficiency, decision-making, and automation. For instance, RL systems have reduced energy consumption by 15% in the energy sector, while in manufacturing, RL-based systems have optimized robotic manipulation tasks and improved quality control.
Integrating Domain Knowledge: Enhancing RL’s Practical Applicability
Recent advances in RL focus on incorporating domain knowledge through constraint satisfaction and human-in-the-loop learning. These innovations enable RL systems to incorporate expert insights, making them more applicable in real-world environments.
Challenges Ahead: Scalability, Safety, and Interpretability
While RL continues to deliver impressive results, several challenges remain, including scalability and safety. RL systems struggle to handle increasing complexity in large-scale applications. Moreover, RL in safety-critical fields, such as autonomous vehicles, requires robust safety protocols.
Future Directions: Towards Robust and Scalable RL Systems
The future of RL lies in overcoming these challenges and pushing the boundaries of what these systems can achieve. Building on these foundational techniques, efforts will focus on developing scalable solutions, improving safety mechanisms, and ensuring RL systems remain interpretable and reliable in diverse applications. As RL continues to mature, early successes like those seen in energy and robotics point to its vast potential in finance, personalized medicine, and logistics automation. These emerging frontiers offer exciting opportunities for RL systems to deliver precision, efficiency, and adaptability at scale.
Debu Sinha’s work continues to bridge the gap between cutting-edge RL research and enterprise-grade deployment. His exploration of the latest innovations in reinforcement learning highlights a field on the cusp of transformative change—already impacting high-stakes domains like autonomous vehicles and healthcare diagnostics. With the continued evolution of hierarchical learning, offline RL, and model-based approaches, RL is poised to solve complex challenges across industries. His insights reflect the direction in which applied AI is headed—toward robust, safe, and scalable systems that deliver measurable business value. As a thought leader shaping best practices in industrial RL adoption, his influence is already helping define the next phase of intelligent automation.
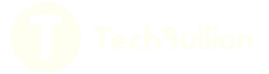