The rapid integration of Artificial Intelligence (AI) and Machine Learning (ML) into financial technology (FinTech) has revolutionized the industry. These technologies have enhanced efficiency, accuracy, and customer experience in unprecedented ways. However, the ethical implications of their use are vast and complex, necessitating a thorough examination. This article explores these ethical concerns, providing insights into their potential impact on society.
The Rise of AI and ML in FinTech
The adoption of AI and ML in FinTech has grown exponentially over the past decade. These technologies are employed in various applications, from customer service chatbots to sophisticated trading algorithms. Their ability to analyze vast amounts of data and make decisions with speed and accuracy has made them invaluable.
Benefits of AI and ML in FinTech
AI and ML offer numerous benefits in the financial sector:
Improved Efficiency:
Automation of routine tasks reduces human error and speeds up processes.
Enhanced Customer Service:
AI-powered chatbots and virtual assistants provide 24/7 customer support.
Fraud Detection:
Advanced algorithms can identify fraudulent activities in real-time, protecting customers and financial institutions.
Investment Strategies:
ML models can analyze market trends and develop profitable trading strategies.
Despite these advantages, the ethical implications of AI and ML must be addressed to ensure their responsible use.
Ethical Concerns in AI and ML
The use of AI and ML in FinTech raises several ethical issues. These concerns revolve around fairness, accountability, transparency, and privacy.
Fairness and Bias
One of the most significant ethical concerns is the potential for bias in AI and ML algorithms. Bias can arise from the data used to train these models or the algorithms themselves. If not addressed, this bias can lead to unfair treatment of certain groups, particularly in areas like credit scoring and loan approvals.
Case Study:
Bias in Credit Scoring
A notable example is the bias observed in credit scoring algorithms. Studies have shown that these algorithms can disproportionately disadvantage minority groups. For instance, if the training data reflects historical biases in lending, the AI system may perpetuate these biases, leading to discriminatory practices.
Accountability and Responsibility
Another critical issue is determining accountability when AI systems make mistakes or cause harm. Unlike human decision-makers, AI lacks the capacity for moral judgment. This raises questions about who should be held responsible for the actions of AI systems.
Example:
Algorithmic Trading Mishaps
In the realm of algorithmic trading, AI-driven systems can execute trades in milliseconds, sometimes leading to market disruptions. When such incidents occur, pinpointing accountability can be challenging. Was it the fault of the developers, the data scientists, or the financial institution?
Transparency and Explainability
AI and ML models, particularly deep learning algorithms, are often considered “black boxes” due to their complexity. This lack of transparency makes it difficult to understand how decisions are made, posing a challenge for regulatory compliance and trust.
The Need for Explainable AI
Explainable AI (XAI) seeks to address this issue by making AI systems more transparent. By understanding how AI models arrive at their decisions, stakeholders can ensure that these systems operate fairly and ethically.
Privacy Concerns
The use of AI and ML in FinTech often involves processing large amounts of personal data. This raises significant privacy concerns, particularly regarding how data is collected, stored, and used.
Data Privacy Regulations
Regulations like the General Data Protection Regulation (GDPR) in Europe and the California Consumer Privacy Act (CCPA) in the United States aim to protect consumers’ data privacy. FinTech companies must navigate these regulations to ensure compliance while leveraging AI and ML technologies.
Ethical Frameworks and Guidelines
To address these ethical concerns, several frameworks and guidelines have been developed. These aim to provide a roadmap for the ethical development and deployment of AI and ML in FinTech.
The Role of Ethical AI Principles
Ethical AI principles often focus on fairness, accountability, and transparency. These principles guide the design and implementation of AI systems to ensure they align with societal values.
Key Ethical AI Principles
Fairness:
Ensuring that AI systems do not perpetuate or amplify biases.
Accountability:
Clearly defining who is responsible for the outcomes of AI systems.
Transparency:
Making AI systems and their decision-making processes understandable to stakeholders.
Industry Initiatives and Standards
Several industry initiatives and standards have emerged to promote ethical AI use in FinTech. These include guidelines from organizations like the Institute of Electrical and Electronics Engineers (IEEE) and the European Commission.
IEEE’s Ethically Aligned Design
The IEEE’s Ethically Aligned Design initiative provides comprehensive guidelines for ethical AI development. It emphasizes the importance of human-centric values in AI systems, promoting transparency, accountability, and fairness.
European Commission’s Ethical Guidelines
The European Commission has also developed ethical guidelines for trustworthy AI. These guidelines focus on ensuring that AI systems are lawful, ethical, and robust, addressing key concerns like privacy, bias, and accountability.
Balancing Innovation and Ethics
The challenge for FinTech companies lies in balancing innovation with ethical considerations. While AI and ML offer significant benefits, their ethical implications cannot be ignored. Companies must adopt a proactive approach to address these concerns, integrating ethical considerations into their AI strategies.
Best Practices for Ethical AI Implementation
To ensure the ethical use of AI and ML, FinTech companies can adopt several best practices:
Diverse and Inclusive Data:
Using diverse datasets can help mitigate biases in AI models.
Regular Audits:
Conducting regular audits of AI systems can identify and address potential ethical issues.
Stakeholder Involvement:
Involving stakeholders in the design and deployment of AI systems ensures that diverse perspectives are considered.
Transparency Measures:
Implementing measures to enhance transparency, such as explainable AI, can build trust and ensure compliance with regulations.
The Role of Regulation
Regulation plays a crucial role in ensuring the ethical use of AI and ML in FinTech. Governments and regulatory bodies must establish clear guidelines and
standards to govern the development and deployment of these technologies.
Regulatory Approaches
Regulatory approaches can vary, from prescriptive regulations that dictate specific requirements to principles-based regulations that provide broad guidelines. A balanced approach, combining both prescriptive and principles-based elements, may be most effective in addressing the ethical implications of AI and ML in FinTech.
Conclusion
The integration of AI and ML in FinTech offers numerous benefits, from improved efficiency to enhanced customer service. However, these technologies also raise significant ethical concerns, including bias, accountability, transparency, and privacy. Addressing these concerns requires a proactive approach, involving the adoption of ethical AI principles, industry standards, and regulatory measures.
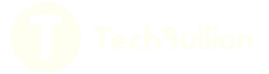