Introduction
Artificial intelligence (AI) has made remarkable strides across various fields, transforming the way we live, work, and create. Among its most fascinating applications is AI image generation, where advanced algorithms produce stunningly realistic images. This blog explores the advancements in AI image generation technology, delving into the innovations that have propelled this field forward.
Understanding AI Image Generation
AI image generation involves the use of sophisticated algorithms to create new images or modify existing ones. These systems rely on key technologies such as Generative Adversarial Networks (GANs), Variational Autoencoders (VAEs), and diffusion models. At their core, these technologies enable computers to learn from vast amounts of data and generate images that mimic real-world visuals.
Generative Adversarial Networks (GANs) are particularly notable for their role in AI image generation. GANs consist of two neural networks—the generator and the discriminator—that work together in a dynamic, adversarial process. The generator creates images, while the discriminator evaluates their authenticity. Over time, the generator improves, producing increasingly realistic images.
Variational Autoencoders (VAEs) take a different approach. They encode input images into a lower-dimensional latent space and then decode them back into images. This process allows for the generation of new images by sampling from the latent space, enabling creativity and variation.
Diffusion models, on the other hand, generate images by iteratively refining random noise. Starting with an image of pure noise, the model progressively improves the image over multiple steps, guided by a learned distribution. This method offers a unique approach to image generation, producing high-quality results.
Major Advancements in AI Image Generation
Generative Adversarial Networks (GANs)
Since their introduction, GANs have undergone significant advancements. Researchers have developed various GAN architectures, such as StyleGAN, which excels at generating high-resolution, photorealistic images. StyleGAN’s ability to manipulate facial features and create diverse variations has made it a cornerstone in the field of AI image generation.
Variational Autoencoders (VAEs)
VAEs have also seen considerable improvements. Modern VAEs incorporate sophisticated techniques to enhance the quality and diversity of generated images. Innovations such as hierarchical VAEs and conditional VAEs have expanded their capabilities, enabling more complex and nuanced image generation.
Diffusion Models
Diffusion models represent a newer frontier in AI image generation. These models leverage the principles of thermodynamics and probability to create images by refining noise over time. Recent breakthroughs have improved the efficiency and quality of diffusion models, making them a promising alternative to GANs and VAEs.
Transformers and Other Emerging Techniques
Transformers, originally designed for natural language processing, have made inroads into image generation. Vision transformers (ViTs) apply the transformer architecture to visual data, offering new possibilities for generating and understanding images. Other emerging techniques, such as neural radiance fields (NeRFs), are pushing the boundaries of 3D image generation and rendering.
Applications of AI Image Generation
Art and Design
AI is transforming the creative process in art and design. Artists are leveraging AI image generators to push the boundaries of traditional art forms, creating pieces that are both innovative and visually striking. For example, AI-generated artworks have been showcased in prestigious galleries and auctions, fetching high prices and sparking debates about the nature of creativity and authorship. Platforms like DeepArt and Artbreeder allow users to blend and morph images, offering new tools for artistic expression.
Entertainment and Media
In the entertainment industry, AI image generation is revolutionizing film, animation, and gaming. AI-generated special effects and characters enable filmmakers to create more immersive and visually stunning experiences. For instance, Disney Research has utilized GANs to create high-quality visual effects that blend seamlessly with live-action footage. In gaming, AI-generated environments and characters enhance realism and interactivity, offering players dynamic and engaging experiences.
Healthcare and Medical Imaging
AI image generation is making significant strides in healthcare, particularly in medical imaging and diagnostics. AI-generated images can assist radiologists in identifying abnormalities and diagnosing diseases with greater accuracy. Techniques such as GAN-based data augmentation help enhance the quality and diversity of medical images, improving the performance of diagnostic models. Additionally, AI-generated 3D models of organs and tissues aid in surgical planning and medical education.
Marketing and Advertising
AI image generation is transforming marketing and advertising by enabling the creation of personalized and engaging content. Brands are using AI to generate customized product images, advertisements, and social media posts that resonate with their target audiences. For example, AI-generated influencers and virtual models are being used in advertising campaigns, offering a novel way to connect with consumers. The ability to quickly generate high-quality visuals helps marketers stay agile and responsive to market trends.
Architectural Design and Real Estate
In architectural design and real estate, AI image generators are being used to create realistic visualizations of buildings and interiors. These tools help architects and developers present their designs in a more compelling way, aiding in decision-making and client presentations. AI-generated renderings can showcase different design options, materials, and lighting conditions, providing a comprehensive view of the final product.
Ethical Considerations and Challenges
Deepfakes and Misinformation
One of the most pressing ethical concerns is the misuse of AI image generation to create deepfakes—highly realistic but fake images or videos. Deepfakes can spread misinformation, manipulate public opinion, and harm reputations. Mitigating this risk requires robust detection tools, regulatory measures, and public awareness campaigns.
Bias and Fairness
Bias in AI training data can lead to unfair and unrepresentative generated images. Addressing this issue involves ensuring diversity in training datasets and implementing fairness algorithms. Ethical AI development must prioritize inclusivity and equity.
Intellectual Property and Copyright
AI image generation raises complex questions about intellectual property and copyright. Ensuring that AI-generated content respects existing copyrights and provides appropriate attribution is essential. Legal frameworks must evolve to address the unique challenges posed by AI.
Future Directions and Innovations
The future of AI image generation is bright, with emerging trends pointing to even greater advancements. Continued research in GANs, VAEs, diffusion models, and transformers will push the boundaries of what AI can achieve. Interdisciplinary collaboration and ethical considerations will play a crucial role in shaping the future of this technology.
Conclusion
AI image generation technology has made significant strides, offering transformative potential across various fields. From revolutionizing art and entertainment to advancing healthcare and marketing, the applications are vast and varied. However, addressing ethical concerns and challenges is paramount to ensuring responsible and beneficial use. By fostering a culture of ethical AI development and embracing ongoing innovation, we can harness the power of AI image generation for a brighter future.
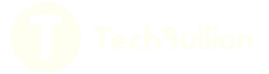