Welcome to the realm of Amazon, where personalized recommendations reign supreme! Have you ever wondered how this e-commerce giant seems to magically anticipate your needs? The answer lies in Amazon’s cutting-edge recommendation systems, fueled by artificial intelligence (AI). In this exposé, we’ll delve into the inner workings of Amazon’s AI algorithms, uncovering the secrets behind their unparalleled ability to suggest products tailored to each individual customer. Buckle up for an enlightening journey through the intricate world of data analytics and machine learning that powers Amazon’s eerily accurate recommendations.
Introduction to Amazon’s AI and Machine Learning Initiatives
Amazon has consistently led the charge in harnessing AI and machine learning to enhance the customer experience. In this article, we’ll closely examine some of Amazon’s advanced recommendation systems powered by these technologies.
Let’s begin with a brief overview of Amazon’s AI and machine learning programs. Amazon’s AWS platform provides developers with a rich array of services for building AI and machine learning applications. Moreover, Amazon offers SageMaker, its fully managed machine learning platform, facilitating model creation, training, and deployment.
Beyond developer tools, Amazon employs AI and machine learning behind the scenes to improve the customer experience. These include Amazon Personalize, which crafts personalized recommendations based on user behavior data like purchases and searches; Amazon Rekognition, an image recognition and analysis service; and Amazon Polly, which converts text into real-time audio.
With this background, let’s explore how these technologies drive recommendations on Amazon.
Amazon Personalize is a machine learning-powered service using algorithms to generate tailored product recommendations. It leverages user behavior data, such as past purchases and search history, to suggest relevant products, aiding customers in discovering new items aligned with their preferences.
Amazon Rekognition, on the other hand, is an image recognition and analysis service capable of identifying objects or text within user-uploaded images. For instance, it can recognize products in a customer’s photo, enabling the system to recommend similar items or related products.
Lastly, Amazon Polly is a text-to-speech synthesis service that transforms written content into real-time audio files. This technology enhances customer experiences by generating voiceovers for videos or delivering spoken product and service information.
In summary, Amazon’s AI and machine learning technologies power a diverse range of recommendation systems, enhancing the customer journey and simplifying product discovery through user data and sophisticated algorithms.
How Amazon Leverages AI and Machine Learning for Recommendations
Amazon has been a trailblazer in utilizing AI and machine learning for recommendations since 1995 when they pioneered the first online recommendation system based on collaborative filtering. This system analyzed customer purchasing behavior and provided recommendations to new customers based on similar customer choices.
Over the years, Amazon continued to invest significantly in improving its recommendation systems. In 2006, they introduced the Amazon ProductGraph, a vast database containing relationships between billions of items, enabling recommendations across various product categories.
In 2012, Amazon acquired Goodreads, a social networking site for book enthusiasts, which enriched their data sources. Goodreads allows users to rate and review books, providing valuable insights to enhance recommendations.
Amazon has also developed proprietary algorithms that go beyond traditional collaborative filtering, incorporating factors like time decay, freshness, and recency into their recommendation systems. These elements combine in Amazon’s algorithms to deliver more precise and personalized recommendations, contributing to the company’s growth and success.
Analyzing the Impact of Amazon’s AI/ML Recommendations on Customer Behavior
Amazon’s AI/ML-powered recommendation system has played a pivotal role in its e-commerce dominance. In 2018, Amazon generated over $232 billion in revenue, representing nearly half of all online sales in the United States. A significant portion of this success can be attributed to their cutting-edge recommendation system, which employs AI and ML to provide personalized suggestions to each customer.
This article delves into how Amazon’s AI/ML recommendations function and their influence on customer behavior. It also touches upon ethical concerns that have arisen regarding these systems.
Amazon’s recommendations are generated by a sophisticated algorithm that considers various factors such as purchase history, browsing behavior, search queries, and abandoned shopping carts. This data is then used to create customized recommendations for each customer.
Amazon’s recommendation system has proven highly effective, driving 35% of all items sold on the platform. For digital products like books and music, this number surges to 50%. These recommendations not only boost Amazon’s sales but also foster customer loyalty. In a recent survey, 60% of respondents expressed that they would not return to Amazon if it didn’t provide personalized recommendations, underscoring their importance to the company’s success.
However, concerns have emerged, including worries that these algorithms might encourage excessive consumerism and potential biases in recommendations. The long-term impact of Amazon’s AI/ML recommendations on customer behavior remains uncertain, but it is undeniable that these systems are pivotal in the realm of e-commerce and are likely to continue shaping it for years to come.
Understanding the Benefits of Amazon’s AI/ML Platforms
Amazon, as one of the world’s largest online retailers, possesses an immense reservoir of data. This data fuels a wide array of AI/ML algorithms that offer diverse benefits to the company.
Prominent among these is Amazon’s use of AI/ML in recommendation systems. These systems leverage historical purchase data and browsing behavior to craft personalized recommendations for each customer. These recommendations improve the customer experience by offering more relevant suggestions, which, in turn, lead to increased sales and customer retention. Moreover, they reduce human curation and manual labor, resulting in cost savings for Amazon.
Amazon has also harnessed AI/ML in various other areas, including automated fulfillment warehouses, fraud detection, and product search ranking. In each case, AI/ML has enhanced efficiency while cutting costs.
Exploring Different Types of Recommendation Systems Utilized by Amazon
Amazon employs two distinct recommendation systems: one for products and another for sellers.
The product recommendation system relies on a customer’s purchase history and browsing behavior to formulate personalized suggestions. The seller recommendation system, on the other hand, examines a customer’s purchase history and identifies patterns by comparing it to other customers’ histories. Based on these patterns, the system recommends sellers from whom the customer might want to purchase.
Both systems harness artificial intelligence (AI). The product recommendation system employs a machine learning algorithm known as collaborative filtering, which analyzes the past behavior of all Amazon customers to identify similarities. The seller recommendation system also utilizes machine learning but employs a different algorithm called content-based filtering, which examines a customer’s past purchases to recommend sellers with similar items.
Examining the Role of Human Intelligence in Combination with ML and AI at Amazon
Human intelligence has played a pivotal role in Amazon’s advanced recommendation systems powered by AI. Amazon’s engineers and data scientists work collaboratively to ensure the recommendations are highly accurate.
The Recommender team at Amazon has consistently sought ways to enhance the customer experience using machine learning (ML) and artificial intelligence (AI). An essential aspect of their strategy involves incorporating human intelligence into the process. Human evaluation and data labeling improve accuracy, surpassing what ML or AI can achieve independently.
To enhance scalability, the team utilizes AWS Lambda, enabling them to run recommendation algorithms in a serverless environment. This flexibility allows for easy scaling without the need to provision or manage servers.
The combination of human intelligence with ML and AI has enabled Amazon’s recommender team to create a more precise and scalable system, delivering superior results for customers.
Conclusion
Amazon’s advanced recommendation system powered by artificial intelligence is a formidable tool benefiting both shoppers and merchants. Leveraging AI’s capabilities, Amazon tailors the experience for individual users, offering personalized recommendations that simplify product discovery. This technology streamlines online shopping, making it more enjoyable for everyone involved while amplifying opportunities for merchants to showcase their products to potential buyers.
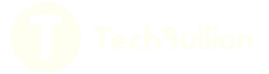