Introduction
For the banking industry that is evolving rapidly, it has become indispensable to integrate Artificial Intelligence (AI) in risk management.
Sanjay Moolchandani, a distinguished Risk Technology Expert in Banking with close to two decades of rich experience examines how banking risk technology can adopt AI, giving banks and financial institutions a competitive edge. Sanjay has pioneered and guided technology strategies in Banking and is optimistic about the future of AI in banking risk management.
The Role of AI in Risk Management
By analyzing a massive amount of data, AI technologies, specifically machine learning, predictive analytics, and natural language processing, can help improve risk assessment by identifying changes, or patterns. This can lead to better decision-making and risk control.
- Data Aggregation and Analysis: Using AI, data can be collected and analyzed from various sources to provide a more comprehensible risk profile. Real-time data from social media, transaction history, and many other data sources, that traditional models in most cases ignore, can be used in case of AI.
- Predictive Modeling: AI allows for predictive risk management by creating models to predict credit risk, default probabilities, and borrower behavior. These models can continuously learn to adjust and alter with new data, improving their accuracy over time.
- Automated Decision-Making: Automation of a lot of risk management processes like credit scoring can be done through AI, which helps in saving time and cost.
- Fraud Detection: AI can protect banks from potential losses by identifying anomalies and behavior patterns that correspond to fraudulent activities. Sophisticated machine learning models can even pick up on the faint clues of fraud that human analysts would miss.
- Real-Time Monitoring: AI enables to monitor in real-time the changes in cases like credit portfolios and provides instant insights & alerts which eases the automation of risk mitigation.
Implementing AI in Banking: Strategies and Considerations
- Technology Integration: Successfully implementing AI requires a strategic approach to ensure seamless integration with existing systems and frameworks. Banks should focus on modular AI solutions that can be gradually integrated into their risk management systems.
- Training and Adaptation: Equipping staff with the necessary skills to utilize AI tools effectively is crucial. Banks should invest in training programs that help employees understand AI outputs and incorporate them into decision-making processes.
- Regulatory Compliance: AI assists banks in adhering to regulatory requirements by providing accurate and timely risk assessments, ensuring compliance with standards. Banks must work closely with regulators to ensure that AI models meet regulatory expectations.
Real-World Applications
Sanjay Moolchandani has initiated several AI-driven initiatives in risk management. Here he talks about some of the main areas where AI can be used.
- Credit Scoring: AI improves traditional credit scoring methods, by including new data forms and sources to get a better assessment of a borrower’s creditworthiness.
- Stress Testing and Scenario Analysis: AI can be used for more advanced Stress Testing and Scenario Analysis which will help banks to assess the impact of economic down cycle/some adverse event on their credit portfolios.
- Regulatory Reporting: AI can help streamline and automate regulatory reporting.
What do Banks need to focus on for AI Implementation?
- Data Management and Quality: Implement a strong data governance framework to ensure that the data feeding the AI model is clear of issues.
- Infrastructure and Technology: Invest in an IT infrastructure that is scalable and flexible to support AI technologies and integrate new solutions with existing systems.
- Talent and Training: Develop a capable workforce skilled in operationalization of AI tools, through targeted training programs and hiring efforts.
- Ethical and Regulatory Compliance: Develop ethical guidelines, and governance practices around AI and a process to ensure regulatory compliance.
- Change Management: Deploy change management tactics such as pilot projects, phased releases, and feedback loops to support the AI transition.
What Banks can benefit from AI Implementation?
- Improved Accuracy: Analysis of vast amounts of data collected by AI helps in reducing the mistakes so there are true conclusions to be derived and decisions to be made; all leading to more accurate risk assessments.
- Efficiency Gains: Using AI to automate routine tasks cuts down on processing times and costs, contributing to more efficient risk management services.
- Proactive Risk Management: AI helps with real-time monitoring and predictive analytics which allows banks to mitigate risks before they turn into a major issue.
- Enhanced Customer Insights: AI can help gain a better understanding of customer behavior and creditworthiness, giving birth to more customized risk strategies.
- Scalability: Banking operations might grow or expand and so AI solutions scale very well to deal with growing volumes of data and transactions.
What are some of the key Challenges in AI Implementation?
- Quality of Data: It is vital to ensure that the data given to AI for analysis is of high quality. To tackle these data quality challenges, banks need to put in place a sound data governance framework.
- Integration with Legacy Systems: AI can be difficult to integrate with traditional systems. Banks or Financial institutions may consider adopting a phased approach, starting with pilot projects to facilitate a smoother transition.
- Ethical and Bias Concerns: Allowing transparency, fairness, and accountability, Banks would need to establish clear guidelines and oversight mechanisms by which to responsibly navigate these ethical implications.
- Regulatory Hurdles: Navigating this space would require collaboration with regulators to ensure AI models meet the compliance requirements.
- Cost and Resource Allocation: Many AI solutions are expensive and require large amounts of computing and data storage capabilities. This would require thoughtful planning and resource allocation by the banks to ensure success and continued sustainability in the long run.
Conclusion
Banks need to adopt AI in risk management to remain competitive and compliant. Sanjay Moolchandani highlights the transformative potential of AI in enhancing risk assessment accuracy, efficiency, and effectiveness. As the banking industry continues to mature, AI will play an increasingly vital role in managing risk and ensuring financial stability.
Final Thought by Sanjay Moolchandani
The integration of AI with banking risk management is a strategic requirement in today’s financial institutions, says Sanjay Moolchandani. Not only does AI improve the accuracy and speed of risk assessment, and it also gives banks the ability to proactively address potential risks before they happen. Taking steps like this in advance can dramatically reduce risk losses, meaning Our Banking System will be much stronger and resilient.
Embracing Innovation: The Banks also need to Embrace Innovation and Invest in AI technologies if they want to have a competitive advantage. This is about building a learning culture and empowering staff to learn, evolve, and use AI most advantageously in their daily working environment. Sanjay Moolchandani calls for a collaborative effort between banks and technology firms, AI experts, regulators, etc. to build well-crafted risk management frameworks powered by AI.
Regulatory Collaboration: Regulation in the case of AI in banking is not easy; therefore, regulatory collaboration is crucial. To ensure their AI models are compliant and are also transparent and fair, Sanjay Moolchandani says it is important for banks to make regulators a part of the development processes very early. Through this partnership, you are not only contributing to reducing your regulatory risks but also increasing trust with customers and stakeholders.
Future Outlook: Going forward, Sanjay is positive about further advancements in AI technologies which will provide organizations with even more robust risk assessment and management tools. This will enable banks to manage the complex financial landscape with agility and accuracy.
Through AI, banks can transform their risk management practices, saving on costs which will make them highly competitive. The future of banking is interconnected with AI, and those institutions that successfully integrate these technologies will be well-positioned to thrive in the years to come.
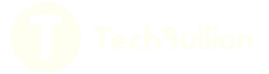