In this modern era, data preparation is a crucial yet time-intensive component of modern analytics workflows, requiring significant effort, precision, and attention to detail. In his latest work, Praneeth Thoutam introduces a transformative framework leveraging artificial intelligence (AI) to automate data cleansing and standardization processes with enhanced efficiency. By integrating supervised and unsupervised learning with deep learning models, natural language processing, and domain-specific insights, this innovation promises to redefine the standards of data quality, operational efficiency, scalability, and adaptability across industries.
The Growing Need for Automated Data Preparation
In today’s data-driven world, enterprises grapple with unprecedented data volume, velocity, and variety, which challenge traditional processes and workflows significantly. Manual data preparation techniques—ranging from spreadsheet manipulations to custom script development—are increasingly inadequate, plagued by scalability issues, human errors, inefficiencies, and limited adaptability to evolving datasets. Traditional methods often consume up to 80% of data professionals’ time, delaying insights, decisions, and project execution in fast-paced and competitive environments.
The AI-Driven Approach: Core Innovations
The framework Praneeth presents employs a combination of machine learning, deep learning, and rule-based automation to tackle critical challenges. By integrating supervised learning models for pattern recognition and unsupervised models for anomaly detection, the system significantly reduces reliance on manual intervention and enhances accuracy. Deep neural networks with advanced architectures handle complex patterns and relationships, ensuring adaptability to dynamic and evolving datasets while maintaining high precision.
The framework also incorporates knowledge graphs, enabling domain-specific reasoning and decision-making for unique industry needs. This feature allows the system to dynamically learn from new data patterns and apply validation rules, fostering consistency, scalability, and adaptability in diverse environments.
Key Features of the Framework
- Automated Inconsistency Detection: The system utilizes cross-platform validation techniques to identify inconsistencies in real-time, reducing data errors by up to 95%.
- Missing Value Handling: Sophisticated imputation methods, including machine learning-based predictions, address missing values efficiently while maintaining the data’s overall integrity and usability.
- Format Standardization: A rule-based transformation engine supported by machine learning ensures seamless normalization of data formats across diverse datasets and environments.
- Output Validation: Multi-layered validation processes ensure that processed data adheres to predefined quality standards, delivering accuracy rates of over 97%, reducing downstream errors.
Performance Metrics: A Leap Ahead
Experimental results demonstrate the framework’s effectiveness in handling large-scale data with efficiency and precision.
- Processing Time: Achieved a 73% reduction compared to manual methods, delivering faster results.
- Accuracy: Maintained a 95.7% detection accuracy for inconsistencies across various data types and sources.
- Scalability: Supported datasets exceeding 500 terabytes with minimal performance degradation, ensuring high reliability.
- Resource Utilization: Optimized CPU and memory usage, reducing operational costs, improving system efficiency, and minimizing environmental impact.
Driving Sustainability Through Automation
Beyond efficiency, the framework emphasizes sustainability by integrating energy-aware practices and resource-efficient processes. By optimizing computational resources and reducing redundancies, it minimizes the environmental footprint of data preparation tasks. These advancements align with global sustainability goals and address operational, ecological, and societal imperatives, making it both innovative and responsible.
Real-World Applications and Implications
The framework’s versatility is evident in its adaptability across industries, from healthcare and finance to retail and manufacturing operations. Its ability to handle structured, semi-structured, and unstructured data ensures broad applicability and supports diverse organizational needs. By automating labor-intensive processes, organizations can redirect resources toward high-value analytical tasks, accelerating innovation, decision-making, and time-to-market strategies.
The Road Ahead: Challenges and Opportunities
While the framework offers groundbreaking advancements, challenges remain in areas such as integration with legacy systems, rare data pattern detection, and data security. Future enhancements may include more robust models, expanded support for diverse data types, and applications in real-time streaming environments. Addressing these challenges will unlock additional opportunities and ensure long-term success for this transformative solution.
In conclusion, Praneeth Thoutam underscores the transformative potential of AI in automating data preparation and cleansing tasks across industries. By combining advanced machine learning techniques, neural networks, and domain-specific knowledge integration, this framework sets a new standard for efficiency, scalability, and sustainability. As organizations face increasing data complexity and quality demands, such innovations pave the way for smarter, faster, and more sustainable data management practices worldwide.
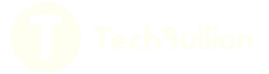