Few names in the dynamic technology scene of today reverberate as strongly as Ashitosh Chitnis, whose significant contributions to data engineering and enterprise analytics have driven him into top positions at tech behemoths including Apple, Google, and IBM. Ashitosh, with more than 17 years of strategic data leadership, has become a visionary profoundly shaping how companies use data and artificial intelligence to generate multimillion-dollar corporate value. “AI-Powered Data Mesh for SAP: Engineering the Future of Enterprise Analytics,” his latest book, confirms his thought leadership in the sector even further. Not long ago, we had the honor to meet Ashitosh to talk about his professional path, ideas, and future vision for artificial intelligence and data management.
Interviewer: Ashitosh, your career has been remarkable, running the gamut from well-known businesses such Apple, Google, and IBM. Could you describe how your career path changed?
Ashitosh Chitnis: Driven by a love of data and its potential to influence choices and corporate development, my path started. From my beginnings as an SAP BW Developer at Deloitte to my current position at Apple, I have always tried to connect business needs with technical solutions by moving through several roles at Delta Systems, IBM, Google, and now Apple. Leading large-scale analytics initiatives across sectors at IBM helped me to first experience different corporate demands and how data may enhance them all.
Interviewer: Overseeing major AI initiatives during your time at Google helped to significantly offset expenses. Could you clarify those encounters?
Ashitosh Chitnis: My job at Google was mostly about productionizing machine learning solutions utilizing Vertex AI, mostly for financial analytics. Business effect was always the main goal. By means of maximized financial modeling, automated data pipelines, and simplified analytics systems, we recovered more than $50 million yearly in costs. Managing worldwide teams of developers also helped me to grasp and negotiate difficult data integration situations, hence guaranteeing compliance and efficiency.
Interviewer: You are now in charge of major Apple initiatives on SAP transformation and AI-driven analytics. In your present position, what major difficulties and possibilities have you faced?
Ashitosh Chitnis: Modernizing the SAP analytics environment for over 10,000 worldwide users is my main challenge—and chance—at Apple. Migrating legacy analytical applications to modern, AI-integrated systems calls for complex data stewardship, democratization of analytics, and strong compliance management. These difficulties, meanwhile, offer special chances to significantly use artificial intelligence and machine learning, thereby improving data integrity, real-time analytics capabilities, and performance optimization.
Interviewer: Your book “AI Powered Data Mesh for SAP” emphasizes a radical new method of corporate analytics. What motivated you to pen this book?
Ashitosh Chitnis: Over my career, I saw consistent problems with centralized data management—lack of agility, segregated insights, and delayed analytics response times. The evolving Data Mesh concept combined with artificial intelligence offered answers to these ongoing issues. Composing this book was my attempt to close the knowledge gap and give companies a strategic plan to move from conventional, centralized models to distributed, AI-powered frameworks.
Interviewer: Could you elaborate on the main advantages Data Mesh offers, especially for SAP-based companies?
Ashitosh Chitnis: Data Mesh changes how companies handle data management by seeing data as a product with distributed ownership. For SAP-based systems, this change directly empowers domain specialists, hence enhancing agility, responsiveness, and insight quality. Artificial intelligence’s integration increases these advantages by automating processes including real-time analytics and data cleaning, hence promoting a culture of strong self-service analytics.
Interviewer: Your book underlines, too, how artificial intelligence improves data quality. Can you give a few illustrations?
Ashitosh Chitnis: Of course. AI-driven data quality solutions can do systematic data cleansing, intelligent lineage tracing, and automated anomaly detection. For instance, algorithms of machine learning integrated with SAP Data Intelligence or SAP HANA can quickly identify abnormalities and discrepancies, therefore greatly enhancing general data integrity and trustworthiness by resolving them proactively.
Interviewer: Your book’s other interesting feature is how artificial intelligence enhances SAP performance and throughput. Could you clarify?
Ashitosh Chitnis: By automating and improving ETL procedures, hence improving cloud integration, and properly controlling load balancing. AI integration maximizes SAP environments. Significant performance increases come from methods like as parallel processing, real-time data access, and automated decision-making driven by artificial intelligence. For companies running at a great size, this is particularly useful since even little increases in efficiency result in significant operational savings.
Interviewer: Measuring ROI from using such sophisticated AI and Data Mesh technologies might be difficult. What advice do you give firms?
Ashitosh Chitnis: The book presents a thorough ROI evaluation tool incorporating cost-benefit analysis, industry benchmarking, and unambiguous AI performance measures. Organizations can obviously show the value obtained from their expenditures by methodically tracking results—such as lower latency, higher data accuracy, and quicker analytical cycles.
Interviewer: Your Google Scholar profile shows a spectrum of publications in several disciplines. Could you describe one of your most significant research efforts and its effect on your present work?
Ashitosh Chitnis: One of my most impactful research endeavors is detailed in my 2023 publication, “SOX Compliance and AI: Automating Financial Audits with Explainable AI.” This work explores the integration of artificial intelligence into the financial auditing process, specifically focusing on automating compliance with the Sarbanes-Oxley Act (SOX). By leveraging explainable AI techniques, the study demonstrates how machine learning models can enhance the accuracy and efficiency of financial audits while maintaining transparency—a critical factor in regulatory environments.
The methodologies and insights from this research have profoundly influenced my current role in leading AI-driven analytics initiatives. The principles of integrating explainable AI into complex financial systems have been instrumental in developing robust analytics frameworks that not only process vast amounts of financial data but also provide clear, interpretable insights for decision-makers. This approach ensures that AI-driven recommendations are both actionable and compliant with stringent financial regulations, thereby driving efficiency and trust in enterprise financial operations.
Interviewer: Your ResearchGate profile highlights your knowledge in artificial intelligence and machine learning. What part do you see these technologies playing in changing sectors like finance and banking, and how do you anticipate them developing in the coming few years?
Ashitosh Chitnis: Over the next few years, especially in industries like Finance, FP&A, Operations, and Procurement, artificial intelligence and machine learning will keep spreading. These technologies, in my opinion, will automate complicated decision-making processes, enhance financial risk assessment, and enable more individualized therapy. To maximize their good influence, artificial intelligence systems must ethical, open, and in line with human values as these domains change.
Interviewer: Considering your background, what future trends do you see in data analytics and artificial intelligence?
Ashitosh Chitnis: Emerging developments such as Edge AI, enhanced analytics, and more democratization of data access will profoundly influence the analytics scene. AI-driven real-time decision-making will also become normal instead of unusual. Driven by ever more advanced artificial intelligence technology, companies will always change their data strategy to stay competitive, robust, and adaptable.
Interviewer: Finally, could you tell us about your leadership philosophy particularly in light of your achievements running several worldwide teams?
Ashitosh Chitnis: In my opinion, efficient leadership is a combination of strategic vision, empathy, and lifelong learning. I place great emphasis on teamwork, well-defined objectives, and promoting creativity at all levels. Leading different worldwide teams calls for knowledge of other points of view and promotion of a culture of inclusiveness and openness. This strategy guarantees constant team development and drive as well as outstanding outcomes.
Interviewer: Any last counsel for would-be data leaders?
Ashitosh Chitnis: Keep inquisitive, welcome creativity, and know well how data fits with corporate results. Keep a significant emphasis on ethical issues of data use and constantly improve your abilities, particularly in changing areas like artificial intelligence and machine learning.
From a fervent data lover to a strategic leader shaping corporate behemoths, Ashitosh Chitnis’s path shows the great promise of data and artificial intelligence when properly exploited. His vision, knowledge, and innovative ideas offer priceless insights and practical direction for companies and aspiring professionals equally. Voices like Ashitosh’s will surely influence the future of enterprise analytics as companies negotiate the complexity of digital transformation, so guaranteeing that data stays a strong instrument for creativity and success.
To learn more about Ashitosh Chitnis, explore his LinkedIn profile here, check out his research on Google Scholar, or connect with him on ResearchGate. His latest book, “AI-Driven Enterprise: Scaling Business Success,” is available on Amazon.
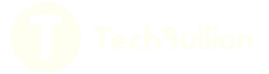