In a rapidly evolving digital landscape, the seamless integration of data and machine learning operations has become a defining factor for business success. Nirmal Sajanraj, an expert in AI/ML implementation, explores this transformation in his recent research, shedding light on how industries are leveraging DataOps and MLOps to optimize performance, enhance compliance, and drive innovation.
The Symbiotic Relationship Between DataOps and MLOps
The convergence of DataOps and MLOps is reshaping how businesses handle data and deploy AI-driven solutions. DataOps ensures high-quality data pipelines through automation and governance, while MLOps extends these principles to machine learning, streamlining development, deployment, and monitoring. Organizations with mature DataOps frameworks report a 60% reduction in data pipeline failures, significantly enhancing AI performance and business outcomes. Collaboration between data scientists and engineers has accelerated AI adoption across enterprises.
Revolutionizing Financial Services with AI Integration
The financial sector has embraced AI-powered analytics to detect fraud, manage risk, and improve customer experience. The adoption of integrated MLOps frameworks has led to a 65% improvement in fraud detection accuracy while reducing false positives by 89%. Real-time transaction monitoring, coupled with automated model validation, ensures compliance and accelerates AI-driven decision-making. These advancements also improve credit scoring models, providing fairer and more accurate financial assessments.
Healthcare’s AI Transformation: Data-Driven Clinical Advancements
In healthcare, AI implementation has revolutionized patient care by enhancing data accuracy and streamlining processes. AI-powered validation systems have increased the accuracy of clinical trial data by 61% while reducing validation time by 52%. Federated learning approaches now allow institutions to collaborate without compromising privacy, leading to improved diagnostics and treatment plans. AI-driven predictive analytics enhances early disease detection and personalized treatment recommendations.
Retail’s Data Revolution: Personalization at Scale
The retail industry has leveraged DataOps and MLOps to enhance customer experiences through hyper-personalization. By integrating multiple data sources into unified customer profiles, businesses have reported a 28% increase in customer value. Real-time experimentation with AI-driven recommendation engines has boosted engagement metrics. AI-powered demand forecasting optimizes inventory management, reducing waste and improving supply chain efficiency.
Manufacturing and the Rise of Smart Factories
In manufacturing, AI-driven predictive maintenance has significantly reduced unplanned downtime, translating into millions in cost savings. The integration of digital twin technology and edge computing has improved equipment effectiveness by up to 25%. These innovations enable real-time analytics, optimizing production efficiency and reducing defects. Smart robotics and automation contribute to precision and quality control in manufacturing.
Telecommunications: AI-Driven Network Optimization
The telecommunications industry is harnessing AI to process vast amounts of real-time data, optimizing network performance. The implementation of AI-driven monitoring has improved anomaly detection accuracy from 72% to 95%, reducing customer-impacting incidents by 83%. Self-healing networks, powered by integrated DataOps and MLOps frameworks, have transformed service reliability while reducing operational costs. AI-enhanced network traffic management improves bandwidth efficiency and service quality for end users.
Ensuring Compliance and Governance Across Industries
Regulatory compliance remains crucial to AI adoption, with financial institutions, healthcare providers, and technology firms implementing governance frameworks to ensure security and transparency. Automated compliance tracking has helped organizations reduce model validation cycles by 60% while improving risk management. The healthcare sector has benefited from end-to-end encryption and automated data masking, leading to an 86% reduction in security incidents. AI-driven monitoring tools help organizations identify and mitigate compliance risks.
The Future of AI Integration: Continuous Improvement and Expansion
As AI adoption matures, organizations recognize the importance of feedback loops between data management and model operations. Industry leaders implementing integrated approaches have seen a 55% improvement in time-to-value for AI initiatives. Future advancements will likely focus on refining industry-specific frameworks, enhancing automation, and expanding AI-driven insights. AI ethics and bias mitigation strategies will gain prominence, ensuring responsible AI implementation across industries.
In conclusion, Nirmal Sajanraj’s research underscores the critical role of DataOps and MLOps in shaping the future of AI-driven business transformations. Companies that embrace these integrated methodologies will continue to experience enhanced efficiency, improved customer experiences, and sustained competitive advantages. The journey towards AI maturity is ongoing, and organizations that prioritize innovation in data and model operations will lead the next wave of digital transformation.
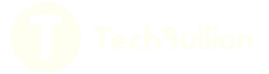