Introduction
In the realm of Artificial Intelligence (AI), the concept of Explainable Artificial Intelligence (XAI) is gaining significant attention. As AI systems become increasingly sophisticated, understanding how they arrive at decisions is crucial for trust, transparency, and accountability. In this article, we delve into the inner workings of XAI, demystifying its principles and shedding light on its importance in today’s AI landscape.
What is XAI?
Explainable Artificial Intelligence (XAI) refers to the ability of AI systems to provide understandable explanations for their decisions and actions. Unlike traditional black-box AI models, which operate without providing insight into their decision-making processes, XAI aims to make AI systems transparent and interpretable to users. By offering explanations, XAI enhances trust in AI systems and enables users to validate, understand, and potentially challenge the decisions made by these systems.
How Does XAI Work?
XAI encompasses a variety of techniques and methodologies aimed at making AI systems interpretable and transparent. These techniques can be broadly categorized into post-hoc explanations and model-specific explanations.
Post-hoc Explanations:
Post-hoc explanations involve generating explanations for AI predictions or decisions after the model has made them. Techniques such as feature importance, saliency maps, and counterfactual explanations fall under this category. These explanations help users understand which features or inputs contributed most to a particular decision, providing valuable insights into the model’s behavior.
Model-specific Explanations:
Model-specific explanations involve designing AI models that inherently provide explanations for their decisions. Techniques such as rule-based systems, decision trees, and symbolic AI fall under this category. These models are designed to prioritize interpretability, making them inherently explainable to users.
Challenges and Limitations
Despite its importance, XAI faces several challenges and limitations. One challenge is the trade-off between transparency and performance. In some cases, making AI systems more interpretable may come at the cost of reduced accuracy or complexity. Balancing transparency with performance is a complex task that requires careful consideration.
Another challenge is the inherent complexity of AI models. Deep learning models, in particular, are highly complex and may be difficult to interpret even with XAI techniques. Simplifying these models without sacrificing performance is an ongoing area of research in the field of XAI.
Additionally, ensuring the fairness and accountability of AI systems remains a challenge. XAI techniques must account for biases in data and mitigate the risk of discrimination or unfairness in AI decisions. Addressing these challenges requires collaboration between researchers, policymakers, and industry stakeholders.
Future Directions
Despite these challenges, the future of XAI looks promising. Continued research and development in the field are driving innovation in interpretable AI techniques. Advancements in areas such as model interpretability, fairness, and accountability are making AI systems more transparent and trustworthy.
Furthermore, regulatory initiatives such as the General Data Protection Regulation (GDPR) and the proposed EU Artificial Intelligence Act are pushing for greater transparency and accountability in AI systems. Compliance with these regulations will likely drive the adoption of XAI techniques across various industries.
Conclusion
In conclusion, Explainable Artificial Intelligence (XAI) plays a crucial role in ensuring transparency, trust, and accountability in AI systems. By providing understandable explanations for AI decisions, XAI enhances user confidence and enables informed decision-making. Despite its challenges, ongoing research and regulatory initiatives are driving progress in the field, paving the way for a more interpretable and trustworthy AI future.
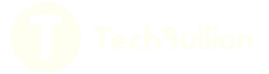