Introduction:
In the dynamic realm of technology, one term that often takes the spotlight is “Artificial Intelligence” (AI). Within this expansive field, there exists a powerful subset that has been pivotal in reshaping our digital landscape—Machine Learning. To delve deeper, this article aims to demystify Machine Learning, providing a comprehensive understanding of its basics, applications, and the transformative impact it has on various industries.
The Foundation:
Understanding Artificial Intelligence
Before delving into Machine Learning, it’s crucial to grasp the broader concept of Artificial Intelligence. AI encompasses the development of intelligent agents that can perform tasks requiring human-like cognitive functions such as learning, problem-solving, and decision-making. It’s the overarching field that houses Machine Learning as one of its key components.
Defining Machine Learning:
Unveiling the Essence
Machine Learning is a subset of AI that focuses on creating algorithms capable of learning from and making predictions or decisions based on data. Unlike traditional programming, where explicit instructions dictate actions, Machine Learning systems leverage data to improve their performance over time. The essence of Machine Learning lies in its ability to enable machines to learn patterns and make decisions without explicit programming.
Types of Machine Learning:
Supervised, Unsupervised, and Reinforcement Learning
Machine Learning operates through various paradigms, each catering to specific use cases. Supervised Learning, the most common type, involves training a model on a labeled dataset, where the algorithm learns to map inputs to corresponding outputs. Unsupervised Learning, on the other hand, deals with unlabeled data, allowing the algorithm to identify patterns and relationships on its own. Lastly, Reinforcement Learning involves an agent learning to make decisions by interacting with an environment, receiving feedback in the form of rewards or penalties.
Applications Across Industries:
Where Machine Learning Thrives
The versatility of Machine Learning has propelled its integration into diverse industries, revolutionizing processes and unlocking new possibilities. In addition, in healthcare, predictive models analyze patient data to assist in disease diagnosis and treatment planning. Similarly, in finance, fraud detection algorithms sift through transactions to identify irregularities. Likewise, the marketing sector benefits from recommendation systems that offer personalized content based on user behavior. By understanding the basics of Machine Learning, one unveils its potential to enhance efficiency and decision-making across the board.
Key Components of Machine Learning:
Algorithms and Data
At the heart of Machine Learning are algorithms, which act as the driving force behind learning and decision-making processes. These algorithms can be categorized into various types, such as decision trees, support vector machines, and neural networks, each suited for specific tasks. However, the effectiveness of these algorithms hinges on the quality and quantity of data available for training. Data, often referred to as the “fuel” for Machine Learning, plays a pivotal role in shaping the accuracy and performance of models.
The Training Process:
From Data to Inference
Machine Learning models undergo a training process where they learn patterns from historical data to make accurate predictions on new, unseen data. During training, the algorithm adjusts its parameters iteratively, minimizing the difference between predicted and actual outcomes. Once adequately trained, the model enters the inference phase, where it applies the learned patterns to new data, making predictions or decisions.
Challenges and Considerations:
Navigating the Complexities
While Machine Learning brings about transformative capabilities, it is not without its challenges. Ensuring the ethical use of AI and addressing issues related to bias in algorithms require careful consideration. Moreover, the need for large datasets and computing power can pose barriers to entry for smaller organizations. Demystifying Machine Learning involves acknowledging these challenges and fostering responsible development practices.
Looking Ahead:
The Future of Machine Learning
As technology continues to advance, the future of Machine Learning holds exciting prospects. Advances in deep learning, a subset of Machine Learning, promise to unlock new levels of complexity and abstraction. Explainable AI, an emerging field, aims to enhance transparency in machine decision-making processes. The integration of Machine Learning with other technologies like the Internet of Things (IoT) and edge computing is set to further expand its applications.
Conclusion:
In conclusion, understanding the basics of Machine Learning is essential for grasping the transformative potential it holds within the broader spectrum of Artificial Intelligence. From its foundational principles to real-world applications, Machine Learning has become a driving force behind innovation. As we demystify the complexities surrounding this powerful technology, it becomes clear that Machine Learning is not just a tool; it’s a catalyst for reshaping how we approach problem-solving, decision-making, and the very fabric of our digital future.
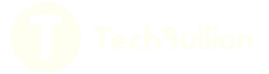