Are you prepared to unlock the mysteries of data analytics? Whether you’re a business owner seeking informed decisions or an aspiring data scientist eager to dive into the realm of numbers, this comprehensive guide serves as your ticket to comprehending and harnessing the power of data. In today’s swiftly evolving digital landscape, unraveling the enigma surrounding data analytics has become more pivotal than ever. Join us on this thrilling journey as we dissect intricate concepts, unveil industry insights, and equip novices with the tools needed to navigate the vast ocean of information.
Understanding Data Analytics
Data analytics entails the process of extracting, transforming, and visualizing data to facilitate informed decision-making. It encompasses an array of techniques and tools, including statistical analysis, machine learning, and data mining.
Data analytics has surged in significance as organizations increasingly depend on data-driven decision-making. While the advent of big data has enabled the accumulation and storage of vast data volumes, it’s through data analytics that this data is transformed into actionable insights.
Several data analytics types exist, with common applications including marketing campaign analysis, customer segmentation, fraud detection, and predictive maintenance. Generally, data analytics can bolster any decision-making process benefiting from deeper comprehension of underlying data.
Advantages of Data Analytics
Data analytics can enhance multiple facets of your business, encompassing but not confined to the following:
Enhanced decision-making: Data analytics enables sifting through copious data to identify patterns and trends, facilitating more informed business decisions.
Elevated customer satisfaction: Profound customer understanding facilitates tailoring products and services to align with their preferences, bolstering customer retention and boosting sales.
Augmented operational efficiency: Data analytics streamlines business processes, eliminating inefficiencies and resulting in cost savings and heightened productivity.
Improved marketing endeavors: In-depth comprehension of the target market facilitates designing more effective marketing campaigns, enhancing conversion rates.
Fraud detection: Advanced data analytics techniques aid in identifying fraudulent activities, safeguarding business finances and reputation by detecting false claims and identity theft.
Embarking on Data Analytics
For newcomers to data analytics, initiating the journey can seem daunting. Encouragingly, numerous resources exist to facilitate a smooth beginning. Here are some tips to commence your data analytics journey:
Grasp the fundamentals: Before delving into data analytics, a grasp of key concepts and terminology is essential. Fortunately, ample online resources are available to facilitate understanding the basics.
Secure a dataset: Following familiarity with fundamentals, practical engagement with real data is essential. Public datasets accessible online provide valuable practice material. Utilize acquired methods to explore and analyze the chosen dataset.
Leverage online resources: An abundance of online resources supports data analytics learning, spanning blogs, online courses, and forums like Stack Overflow.
Engage in hands-on practice: The most effective data analytics learning method is hands-on experience. Source datasets and commence honing your skills.
Varieties of Data Analytics
Data analytics comprises four primary types: descriptive, diagnostic, predictive, and prescriptive.
Descriptive analytics summarizes data to address “What has occurred?” Basic statistics like counts, means, medians, and standard deviations are employed to depict dataset characteristics.
Diagnostic analytics elucidates causality, addressing “Why did this happen?” By scrutinizing data, this form identifies correlations and relationships.
Predictive analytics forecasts potential occurrences by leveraging historical data to answer “What could happen?” Techniques such as regression analysis anticipate future events.
Prescriptive analytics surpasses predictive analytics, answering “What should we do?” This type considers constraints and suggests actions aligned with desired outcomes.
Tools and Technologies for Data Analysis
Diverse tools and technologies are accessible for data analysis. Standard options include spreadsheet programs like Microsoft Excel or Google Sheets. Database management systems, statistical analysis software, and data visualization tools are also prevalent.
Database management systems (DBMS) store, organize, and retrieve data from databases. Notable DBMS options include MySQL, Oracle Database, Microsoft SQL Server, and PostgreSQL.
Statistical analysis software evaluates numerical data. Prominent examples are SAS, SPSS, Minitab, and R.
Data visualization tools craft graphical data depictions. Common tools comprise Tableau, QlikView, and Microsoft Power BI.
Data Analysis Best Practices
Tailoring data analysis approaches to specific datasets and analytical objectives is pivotal, as there’s no universal methodology. Vital best practices encompass:
- Exploratory data analysis: Initial data examination fosters understanding of structure and content, revealing patterns and relationships not immediately apparent.
- Data cleaning and preprocessing: Prior to formal analysis, data cleaning eliminates invalid or absent values. Preprocessing tasks like normalization and feature selection might be necessary.
- Statistical analysis: After data preparation, diverse statistical techniques are applied to address dataset questions, spanning hypothesis testing, correlation analysis, regression analysis, and more.
- Machine learning: Complex datasets may necessitate machine learning for model creation enabling predictions or classifications.
Common Data Analytics Pitfalls to Sidestep
Novices frequently encounter common data analytics pitfalls. Evade these pitfalls for a more effective approach:
Undefined analysis goals: Clear analysis objectives must precede any undertaking. Goals guide data collection and analysis strategies.
Insufficient data collection: More data typically yields better results. Adequate data accumulation enhances pattern identification.
Inadequate data cleansing: Cleaning data by removing erroneous or missing entries is vital. Unclean data skews results.
Premature conclusions: Patterns in data aren’t necessarily conclusive. Employ statistical methods to validate findings.
Conclusion
Data analytics is a gateway to improved business decisions and customer insight. This guide simplifies data analytics basics, debunking misconceptions and providing a foundation for harnessing reliable data for informed choices. Armed with this knowledge, embark on your exploratory journey.
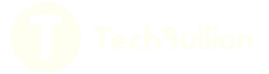