Introduction:
Artificial Intelligence (AI) has become an integral part of our daily lives, impacting various industries and transforming the way we work. One of the key elements in the development and deployment of AI systems is the process of Artificial Intelligence Training. In this comprehensive guide, we’ll unravel the complexities surrounding AI training, providing beginners with a clear understanding of its importance, processes involved, and practical applications.
Understanding the Basics:
What is Artificial Intelligence Training?
Artificial Intelligence Training refers to the process of teaching machines to perform tasks by exposing them to vast amounts of data and allowing them to learn patterns and make decisions. This training enables AI systems to improve their performance over time without explicit programming, mimicking human-like cognitive functions.
The Role of Data in AI Training:
At the heart of AI training is data. Machines analyze and learn from diverse datasets, recognizing patterns and gaining insights. Quality, quantity, and diversity of data are crucial for effective AI training, as they directly impact the system’s ability to generalize and make accurate predictions.
Key Components of AI Training:
Algorithms:
The Building Blocks:
Algorithms serve as the backbone of AI training. These mathematical models process input data, identify patterns, and make predictions or decisions. Choosing the right algorithm depends on the nature of the task, whether it’s image recognition, natural language processing, or something else.
Neural Networks:
Emulating the Human Brain:
Neural networks, inspired by the human brain, play a significant role in AI training. These interconnected layers of nodes process information, allowing the system to recognize complex patterns. Understanding the architecture of neural networks is essential for grasping the nuances of AI training.
Training and Testing Phases:
AI training is a two-step process involving training and testing phases. During training, the model learns from labeled datasets, adjusting its parameters to minimize errors. The testing phase assesses the model’s performance on new, unseen data, ensuring its ability to generalize and make accurate predictions in real-world scenarios.
Challenges in AI Training:
Overfitting and Underfitting:
One common challenge in AI training is finding the right balance between overfitting and underfitting. Overfitting occurs when the model learns the training data too well but struggles with new data, while underfitting indicates the model’s inability to capture the underlying patterns.
Lack of Quality Data:
The success of AI training heavily relies on the quality of available data. Incomplete or biased datasets can lead to skewed results, impacting the model’s effectiveness and generalization capabilities.
Choosing the Right Framework:
TensorFlow vs. PyTorch:
Two popular frameworks for AI training are TensorFlow and PyTorch. TensorFlow, developed by Google, offers a comprehensive ecosystem, while PyTorch, favored for its simplicity, is gaining popularity. Choosing the right framework depends on factors such as project requirements, ease of use, and community support.
Real-world Applications:
Healthcare:
Revolutionizing Diagnostics:
AI training has made significant strides in healthcare, particularly in diagnostics. Machine learning models, trained on vast medical datasets, can analyze images and identify abnormalities with a level of accuracy that surpasses traditional methods.
Finance:
Enhancing Fraud Detection:
In the financial sector, AI training is employed to enhance fraud detection. By analyzing transaction patterns and identifying anomalies, AI systems can alert financial institutions to potential fraudulent activities, mitigating risks and safeguarding customers.
The Future of AI Training:
Continuous Learning and Adaptability:
The field of AI training is dynamic, with continuous advancements shaping its future. The concept of lifelong learning for AI systems, allowing them to adapt to evolving circumstances, is gaining prominence. This adaptability ensures AI’s relevance in an ever-changing technological landscape.
Ethical Considerations:
As AI becomes more ingrained in society, ethical considerations surrounding AI training are paramount. Ensuring fairness, transparency, and accountability in AI systems is crucial to prevent biases and address the ethical challenges associated with automated decision-making.
Conclusion:
Demystifying Artificial Intelligence Training is essential for beginners seeking to understand the intricacies of this transformative technology. From the basics of algorithms and neural networks to real-world applications and ethical considerations, AI training is a multifaceted process that shapes the future of technology. As we navigate this ever-evolving landscape, a solid understanding of AI training becomes not only beneficial but imperative for anyone looking to be part of the AI revolution.
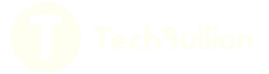