Fraud detection in financial transactions has evolved significantly with technological advancements. Avinash Rahul Gudimetla, an expert in financial security, has introduced an innovative decentralized fraud detection system powered by federated learning. His research highlights how this approach enhances detection accuracy while maintaining data privacy.
Rethinking Fraud Detection with Federated Learning
Traditional fraud detection systems rely on centralized databases, which makes them vulnerable to data breaches and inefficient at detecting evolving fraud patterns. By contrast, this decentralized model uses federated learning to enable financial institutions to collaborate on fraud detection without sharing sensitive customer data. This approach ensures privacy compliance while improving fraud detection accuracy by 28.7% compared to conventional systems.
Advanced Privacy Protection with Homomorphic Encryption
A major innovation in this system is the use of homomorphic encryption, which enables encrypted data processing without decryption. This approach prevents unauthorized access while allowing real-time fraud detection. The system operates with an impressive latency of just 0.65 milliseconds per encrypted operation, proving its efficiency even under high transaction volumes.Additionally, the architecture implements multi-layered security protocols with zero-knowledge proofs to validate transactions without exposing sensitive information. The system scales horizontally across distributed nodes, maintaining performance integrity during peak periods while adapting dynamically to emerging threat vectors through continuous machine learning optimization of detection algorithms.
Adaptive Model Architecture for Scalability
The decentralized framework incorporates an adaptive model architecture that can process up to 1.8 million transactions per second. It also features a multi-tiered batch processing strategy to manage extreme load conditions, ensuring seamless fraud detection even during high-traffic periods.
To further enhance performance, the system implements dynamic sharding across distributed nodes, automatically redirecting computational resources based on real-time demand patterns. This load-balancing mechanism operates alongside specialized memory caching protocols that prioritize frequently accessed transaction templates. The framework’s self-healing capabilities detect and isolate processing bottlenecks, initiating automatic recovery procedures without compromising security or data integrity, making it ideal for mission-critical financial operations with unpredictable volume fluctuations.
Mitigating Emerging Fraud Patterns
Fraudsters continuously adapt their tactics, making it essential for fraud detection systems to evolve. These systems employ continuous learning mechanisms that maintain a 96.2% detection accuracy even during high-risk periods. By leveraging temporal correlation and cross-institutional analysis, they identify 94.2% of emerging fraud patterns within the first 125 transactions, reducing response time by 41.8%.The systems also deploy advanced behavioral biometrics that analyze typing patterns, device handling, and transaction sequencing to create unique digital fingerprints for users.
Balancing Security and Regulatory Compliance
Financial institutions must follow strict rules, but these rules often conflict about how data can be shared. Federated learning solves this problem by keeping sensitive transaction data locally while still allowing different institutions to work together on training AI models. This approach, combined with automated checks to verify compliance with regulations, has made the compliance process 84% more efficient.
This solution implements adaptive governance protocols that dynamically adjust to regulatory changes across different regions. The system’s audit trail creates immutable records of all model updates and data interactions, satisfying oversight requirements without compromising security. Cross-border transactions benefit from jurisdiction-specific rule engines that automatically enforce local regulations while maintaining global consistency, significantly reducing manual compliance reviews and associated operational costs..
Reducing False Positives and Enhancing Accuracy
A key challenge in fraud detection is balancing security with customer experience by minimizing false positives. While traditional fraud detection systems average a 2.8% false positive rate, advanced AI-powered fraud detection systems reduce this to just 0.97%, ensuring legitimate transactions proceed while maintaining high fraud detection accuracy.
In Conclusion,As digital transactions continue to grow, financial fraud is becoming more sophisticated. The integration of federated learning, homomorphic encryption, and adaptive processing architectures presents a transformative shift in fraud prevention. Future developments in quantum-resistant cryptography and blockchain-based verification may further enhance security and efficiency. Avinash Rahul Gudimetla’s work lays the foundation for a more secure and privacy-preserving approach to fraud detection. By addressing key challenges such as data privacy, regulatory compliance, and real-time fraud mitigation, this decentralized system represents a significant advancement in financial security.
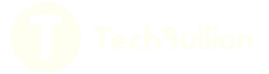