We are excited to welcome Metin Sarikaya, a distinguished expert in the realm of data, business intelligence, and analytics. With an impressive 15-year track record, Metin has been at the forefront of data warehouse projects across the Telecommunications and Finance sectors, bringing a wealth of experience to our conversation today.
Currently serving as the Head of Data Warehouse, Business Intelligence and Big Data at Akbank, one of Turkey’s leading financial institutions, Metin has climbed the ranks from DWH Architect to his current leadership role. His journey through various positions at Akbank, coupled with his prior experience at Avea in the Telco sector, provides a unique perspective on the evolution and application of data technologies across industries.
As someone with 15 years of experience in data, BI, and analytics, how have you seen the field evolve, particularly in the Telco and Finance sectors?
Over the past 15 years, I’ve seen data, BI, and analytics undergo a major transformation, especially in the Telco and Finance sectors. Initially, data was something static—mainly used for historical reporting and simple trend analysis. But today, it’s become a dynamic, strategic asset that influences decision-making at every level of an organization.
In Telco, the amount and complexity of data have grown dramatically, especially with mobile devices, IoT, and 5G. It used to be about simple metrics like call durations or subscription counts. Now, it’s about using real-time data to predict customer behavior, improve user experiences, and optimize network performance. Advanced analytics, AI, and machine learning have become essential for making sense of this data, allowing Telco companies to offer more personalized services and streamline their operations.
In Finance, data has shifted from being a tool for regulatory compliance to becoming a competitive edge. It’s no longer just about reporting numbers—now it’s about forecasting trends, managing risks, and building better customer relationships. The rise of big data platforms and cloud solutions has made real-time data processing possible, helping banks become more agile in their decision-making. The growth of fintech has further pushed this shift, as traditional banks now face competition from nimble, data-driven startups.
One common trend across both sectors is the increased focus on data governance and quality. With data being central to decision-making, it’s crucial to maintain accuracy, security, and compliance. Additionally, self-service BI tools have become important, enabling business users to derive insights without having to always depend on IT teams.
You’ve been with Akbank for over 9 years, progressing to Head of Data Warehouse, Business Intelligence and Big Data. What has been your most significant achievement in this role?
During my time at Akbank, I’ve had the opportunity to work across many teams and roles, each one presenting unique challenges. One of the biggest challenges we’ve faced was the complexity of our Data Warehouse. Over time, department-specific, project-based data models were created, which led to problems like confusion over data definitions, data duplication, and ultimately, reduced data quality.
My biggest achievement in this role has been restructuring our data environment by implementing a Common Data Mart Layer. We designed this layer with data governance principles in mind—grouping datasets, ensuring consistent definitions, maintaining measurable quality, and reducing redundancies. This has helped us make our analytics, machine learning, and AI processes much faster, more reliable, and easier to execute.
Can you describe a challenging data warehouse project you’ve worked on in Telco or Finance and how you overcame the main obstacles?
One of the toughest projects I’ve worked on was modernizing our Data Warehouse at Akbank to create a more integrated architecture. Over the years, the data infrastructure had grown into something complex and fragmented, with department-specific silos. This made it difficult to ensure consistency, avoid redundancies, and get access to reliable data for analytics and reporting.
The first challenge was dealing with the sheer volume of legacy data, which was spread across countless isolated data marts. To tackle this, we implemented data governance and worked on defining over 30 key data sets across the organization. We collaborated closely with different business units to establish these data domains, ensuring that their needs were met while enhancing data clarity and consistency.
Another major challenge was the cultural resistance to change. Many business units had grown comfortable with their own systems, so getting everyone on board with a unified data platform required a shift in mindset. We overcame this by engaging stakeholders from the start, showing them the advantages through proof-of-concept projects, and ensuring the new approach would ultimately make their work easier and more efficient.
The cornerstone of our solution was creating the Common Data Mart Layer (CDM), which unified all those disparate data models into a cohesive structure. By applying data governance principles, we standardized definitions, continuously measured quality, and eliminated redundancy. This has vastly improved our reporting and analytics capabilities, resulting in faster and more accurate insights. Overall, this project not only boosted the operational efficiency of our Data Warehouse but also helped cultivate a more data-driven culture within the organization.
How do you approach integrating Big Data technologies with traditional data warehouse systems in a large financial institution like Akbank?
Integrating Big Data technologies with a traditional data warehouse at a large financial institution like Akbank is all about balancing the best of both worlds. Traditional data warehouses are great for handling structured, transactional data—ideal for reporting, regulatory compliance, and standard BI tasks. Big Data platforms, on the other hand, excel at handling massive volumes of unstructured or semi-structured data, which makes them perfect for real-time analytics and machine learning.
At Akbank, we’ve built a hybrid architecture that combines the reliability of our traditional data warehouse with the flexibility and power of Big Data technologies. We use Hadoop-based systems to manage and process large amounts of unstructured data, such as logs from our mobile apps and customer interactions. Insights derived from this Big Data environment are then integrated back into our traditional data warehouse for comprehensive reporting and analysis.
In your role as DWH Architectural Team Manager, what strategies did you employ to ensure your team stayed current with rapidly evolving data technologies?
When I took on the role of Architectural Team Manager, one of my first priorities was to establish clear ETL and architectural standards across the organization. These standards helped everyone stay on the same page. After formalizing these standards, we launched a project to measure the quality of our software continuously. The idea was simple—”You can’t manage what you don’t measure.” We developed a system that evaluated our software’s adherence to these standards, giving each piece a quality score. This allowed teams to track their progress and identify areas for improvement.
We also set up two committees made up of the most experienced developers and analysts from each team. These committees met regularly to discuss standards, address challenges, explore emerging technologies, and share solutions. This approach fostered a culture of continuous learning and ensured that our teams were always aware of the latest developments.
Can you elaborate on the Argus Project you led at Akbank? What were its main objectives and outcomes?
The Argus Project aimed to create a modern analytics platform that could keep up with Akbank’s evolving needs in a highly competitive financial landscape. We wanted to move beyond static reports and isolated data silos and create a platform that delivered real-time, high-quality data accessible across the entire organization—from branch managers to top executives.
As a result of the Argus Project, we’re now processing about 5 billion rows of data daily, generating over 5000 metrics, and performing trend calculations across more than 40 billion records each day. The platform supports multiple timeframes—from intra-day to periodic analysis—giving us a real-time edge in decision-making. We also built an intuitive interface that’s used by 7000 people across PCs and mobile devices, making data more accessible than ever before. With the new architecture, we can develop metrics faster, handle data processing in parallel, and roll back metrics if needed.
Having worked in both Telco (Avea) and Finance (Akbank) sectors, how do the data challenges and opportunities differ between these industries?
Having experience in both the Telco and Finance sectors has shown me just how differently data can be used. In Telco, the challenge lies in managing the sheer volume and speed of data—network interactions like calls, data usage, and device connections generate a huge amount of information. The opportunities are in leveraging real-time insights for network optimization, personalized offers, and fraud detection. But it’s tough to bring together and analyze this diverse, often unstructured data in a way that’s useful.
In Finance, the challenge isn’t so much about the volume but more about ensuring data quality and governance. The stakes are higher—regulatory compliance is a big factor, and the data is used for critical activities like financial reporting and risk management. Opportunities in this sector revolve around using data to improve customer experience, proactively manage risk, and develop personalized products through analytics and AI.
As Head of Data Warehouse, Business Intelligence and Big Data, how do you balance the need for data governance and security with the demand for quick, accessible insights?
Balancing governance and security with the need for fast insights is one of the biggest challenges I face. We’ve approached this by understanding that data governance and quick access can actually support each other. Trust in data starts with solid governance—if our data isn’t accurate, compliant, or secure, it’s useless for decision-making. So, we’ve implemented strong governance frameworks, including access controls, encryption, and regular audits to maintain data integrity.
At the same time, we want to empower our business teams to make data-driven decisions quickly. We’ve invested in self-service BI tools and created a Common Data Layer (CDM) that ensures all data is centrally governed but easily accessible. This approach helps us meet both goals—governance and quick, reliable insights.
In your experience, what are the key factors in successfully implementing advanced analytics in a large financial institution?
Successfully implementing advanced analytics in a large financial institution depends on a mix of strategic focus, quality data, the right technology, cultural readiness, and executive backing.
First, advanced analytics needs to be aligned with business objectives. It’s about using analytics to directly support goals like improving customer experience or managing risks better. Data governance is crucial—reliable analytics depend on accurate, high-quality data. The technology infrastructure also matters; cloud platforms and AI tools help run complex analyses at scale.
Fostering a data-driven culture is also key. We need people to see data as an asset, not just a byproduct of their work. Promoting data literacy and using self-service analytics tools helps embed this thinking into everyday decision-making. Finally, having strong support from leadership is critical. This ensures that analytics initiatives have the resources they need and are aligned with the broader strategy of the institution.
Looking ahead, what emerging trends or technologies in data and BI do you believe will have the most significant impact on the finance sector in the next 5 years?
I think several trends will significantly impact the finance sector over the next five years.
First, AI and machine learning will keep changing the game, especially in areas like fraud detection, risk management, and creating personalized customer experiences. As these technologies and our ability to process data improve, banks will be able to get deeper insights in real time.
Real-Time Analytics will also become even more crucial. Financial institutions that can analyze and act on data instantly will have a big advantage, whether it’s reacting to market shifts or improving customer engagement.
Data democratization through self-service BI tools is another trend that will continue to grow. The easier it becomes for business users to access and use data, the less they’ll need to rely on IT departments, speeding up decision-making across the board.
Finally, the Data Lakehouse architecture will be a game-changer for financial data management. By combining the best of data lakes and warehouses, lakehouses allow organizations to manage structured and unstructured data on a unified platform—making advanced analytics more seamless and cost-effective. This flexibility will be crucial as banks continue to deal with diverse and growing datasets.
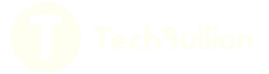