Artificial intelligence (AI) is only as good as the data that powers it. Whether it’s a machine learning model for image recognition, language processing, or business analytics, one thing that remains constant is that the quality of your dataset will directly impact your outcomes.
That’s where data curation steps in- A very important practice that ensures your data sets are accurate, have the right structure in them. Let’s explore why data curation is essential and how it’s shaping the future of AI development
What is Data Curation?
Data curation comprises the processes of selecting, and organizing data, making datasets usable across the AI life-cycle and ensuring their usability. It’s more than just collecting data. It is about elaborating raw information into useful processes that can be used to build machine learning models.
Data curation, in practice, comprises:
- Cleaning data sets in order to get rid of irrelevant or wrong appertaining information.
- Shaping the information in a targeted structure so that it is easy and quick to retrieve.
- Labeling and annotating information in a standardized manner to provide uniformity.
- Incorporating the new data with the existing datasets and enhancing them.
Without a robust curation process, datasets can become noisy, biased, or incomplete—leading to underperforming AI models.
Why Data Curation Matters in Machine Learning
AI now dominates the space and the significance of datasets prepared in a suitable manner is something that is universally agreed upon and here is why data curation should be the prime focus in any machine learning activity:
1. Enhanced Model Accuracy
Accurate data leads to accurate models. By prioritizing curation, you can minimize errors and inconsistencies that might otherwise compromise your AI’s performance.
2. Streamlined Development
Curated datasets reduce the time spent debugging or retraining models, speeding up development cycles and improving efficiency.
3. Reduced Bias and Noise
Bias in datasets is a common challenge in machine learning. Effective curation helps mitigate this issue by ensuring diverse and balanced data inputs.
The Most Important Reason for Data Curation in Machine Learning
Problems Associated with Data Curation
Despite its importance, data curation comes with its own set of challenges:
- Scale: Handing large sets of data requires a good proportion of human control with automation at scale.
- Annotation Complexity: As data sets become more diverse there is increased difficulty in consistency when it comes to labelling.
- Evolving Requirements: AI projects often require iterative updates to datasets, making long-term maintenance a priority.
With the help of the right tools and right strategies, these problems can be converted into possibilities.
The Future of Data Curation
As the market for AI tools and solutions increases, the requirement for high-quality and well-structured datasets is only going to go up. New tools are using AI to aid the process of the curation itself, allowing for the better and quicker preparation of datasets for analysis.
Superb AI has started to take part in and is also leading this transformation by offering AI-enabled features that enable teams to optimize how they work with data. Such platforms, whether it’s
Conclusion
Data curation is an important engine for AI progress. Spending resources on the curation process allows companies to achieve better model results, reduced time invested in deploying a project as well as finding an ongoing benefit from the AI model.
If you are dealing with the complexities of data curation, maybe it is high time, you look for tools and approaches that will make the task easier for you. High-quality datasets aren’t just a nice-to-have—they’re a necessity in today’s AI-driven world.
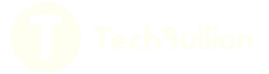