In 2024, data analysis continues to evolve as one of the most critical processes for businesses seeking to harness the power of data analytics and data science. With advancements in predictive models and artificial intelligence (AI), companies are transforming and modeling data, turning raw data sets into actionable business insights. Modeling data allows organizations to discover patterns and make more informed decisions. Data analytics is the process of systematically examining raw data to extract value, enabling businesses to make data-driven decisions that optimize operations, enhance customer experiences, and improve overall performance.
The data analysis process has expanded beyond historical data to include predictive analysis, which anticipates future trends and outcomes. Through analyzing and visualizing data, companies use data to discover insights, draw conclusions, and identify key factors that influence success. Data analysis involves collecting and inspecting information through structured data analysis plans, helping businesses shape better strategies. The role of analysis services is essential in supporting these processes by providing the tools needed to conduct in-depth examinations, ensuring that decisions data analysis drives growth and efficiency.
Exploratory data analysis : How Predictive Analytics and AI Are Reshaping Business Decision-Making in 2024
Predictive analytics and AI play pivotal roles in data science today, enhancing how businesses analyze data to forecast trends and make informed decisions. The integration of machine learning (ML) algorithms and data mining techniques enables companies to detect patterns in large data sets and predict future behaviors. This capability is crucial in industries such as retail, finance, and healthcare, where accurate forecasting can significantly impact operational efficiency and customer satisfaction.
In this rapidly evolving field, several data analysis techniques are becoming essential. These include time series analysis, which evaluates data points over time to identify trends, and regression analysis, a method used to predict relationships between variables. Additionally, factor analysis and cluster analysis help businesses categorize data into meaningful groups, facilitating more effective decision-making. Staying updated with the latest news in data analytics ensures that businesses are leveraging cutting-edge techniques and staying competitive in an ever-changing landscape.
Examples of AI in Predictive Analytics:
- Sales forecasting: Using historical sales data, AI can predict future sales patterns, enabling businesses to adjust inventory levels and marketing strategies.
- Customer behavior analysis: AI-driven models analyze customer purchase histories to recommend personalized products and services.
- Risk management: By examining big data, AI can identify potential risks and opportunities, allowing businesses to allocate resources more effectively.
introduction to data science for Enhanced Business Insights
The data analysis process is central to understanding complex business environments. This process typically involves data collection, data cleaning, and exploratory data analysis (EDA), which helps uncover underlying patterns within a data set. Data scientists use these insights to build predictive models that aid in decision-making. As data visualization tools become more sophisticated, decision-makers can quickly interpret data points and trends without needing to sift through raw data.
For instance, in retail, data visualization techniques allow managers to see customer behavior patterns, helping them adjust store layouts or marketing campaigns. These concise visual representations make it easier to communicate complex insights to stakeholders, facilitating quicker and more informed decisions with regular updates to reflect real-time data.
The Importance of Data Analysis in 2024
The importance of data analysis cannot be overstated in today’s data-driven world. Businesses rely on data analytics to understand market trends, optimize internal processes, and improve customer engagement. By using advanced data analysis methods such as prescriptive analysis, companies not only predict future trends but also receive actionable recommendations for achieving optimal outcomes. This is particularly valuable in industries like healthcare, where AI can suggest treatments based on predictive models built from patient data.
Additionally, spatial analysis has gained traction in 2024, particularly in sectors like logistics and real estate. By analyzing geographical data, businesses can optimize routes, predict real estate trends, and improve supply chain efficiency. Data engineers play a critical role in this ecosystem by ensuring that the infrastructure for data collection and analysis is robust and scalable.
tools for data analysis and Techniques for use data Analytics
The growing demand for advanced data analytics has led to the development of various tools that streamline the data analysis process. Popular tools such as Python, R, and Google Data Analytics enable data professionals to manage and analyze large volumes of data efficiently. These tools are essential for conducting quantitative data analysis, regression analysis, and diagnostic analysis, all of which help businesses derive deeper insights from their data.
One significant advancement in 2024 is the rise of augmented analytics, which combines AI with traditional data analytics to automate data preparation and insight generation. This not only accelerates the data analysis process but also makes data analytics more accessible to non-technical users. For example, a marketing team can use augmented analytics to identify customer segments and tailor campaigns without needing extensive programming knowledge.
Key steps in data analysis Process
The data analysis process typically follows a structured approach to ensure accurate and meaningful insights are derived. The key steps include:
- Data collection: Gathering relevant data from various sources such as databases, surveys, or sensors.
- Data cleaning: Removing inconsistencies, missing data, and errors from the data set.
- Exploratory data analysis (EDA): Identifying patterns, correlations, and anomalies in the data.
- Model building: Using statistical and machine learning models to predict future outcomes.
- Data visualization: Presenting findings through charts, graphs, and dashboards to facilitate decision-making.
These steps are integral to transforming raw data into insights that drive business strategies. Data scientists and data analysts play crucial roles in this process, applying their expertise to interpret data and communicate findings effectively.
skills for data analysis and visualization
As businesses increasingly rely on data analytics for decision-making, the demand for skilled professionals in this field has skyrocketed. Careers in data analysis, such as becoming a data analyst, data scientist, or data engineer, offer lucrative opportunities for those with the right skill set. In 2024, professionals skilled in data science in Python, statistical analysis, and data mining are particularly sought after due to the increasing complexity of data.
To meet this demand, many universities and online platforms offer data analysis courses that cover essential skills such as data collection, data cleaning, exploratory data analysis, and predictive analysis. Additionally, specialized programs such as Google Data Analytics certifications help aspiring professionals gain hands-on experience with industry-standard tools.
Examples of career in data analysis :
- Data Scientist: Focuses on creating predictive models and deriving insights from complex data.
- Data Engineer: Manages and optimizes the infrastructure needed for data analysis.
- Data Analyst: Interprets data to help businesses make informed decisions.
These roles are crucial in industries ranging from finance to healthcare, where data plays an essential role in operational efficiency and customer satisfaction.
Data Science in Python
Data science in Python has become a vital part of the data analysis process in 2024. Python is widely used due to its extensive libraries like Pandas, NumPy, and SciPy, which allow data scientists to manage structured and unstructured data efficiently. Python’s capabilities in data visualization also play a critical role in helping businesses explore and represent data effectively, allowing decision-makers to understand the data at a glance.
For example, Python’s exploratory data analysis (EDA) tools help data professionals analyze the data by identifying patterns and anomalies within the data. This process often involves analyzing historical data to uncover trends that can influence business strategies. Moreover, Python’s integration with machine learning frameworks such as TensorFlow enables the development of predictive models that leverage predictive analysis uses, such as sales forecasting based on historical sales data. This makes data science in Python a go-to choice for any organization engaging in data analytics projects, and just as Backlink Checkers help track SEO performance, Python tools provide deep insights that drive data-driven strategies.
Data Analysis Methods
When approaching data analysis methods, businesses today utilize a variety of techniques to derive insights from their data collected. The data analysis process typically begins with descriptive analysis, which helps summarize historical data by identifying trends, patterns, and relationships within the dataset. This method is often the first step in understanding representations of the data and is critical for organizations that need a clear view of their current performance.
Predictive analysis, another common type of analysis, goes beyond description to forecast future trends. By leveraging analyzing sales data, businesses can use predictive models to anticipate market demands and adjust their strategies accordingly. Diagnostic analysis, which determines the causes of certain outcomes, is also frequently used. This method helps businesses pinpoint issues within their processes and optimize operations by suggesting specific interventions. For instance, diagnostic analysis might reveal that certain marketing efforts lead to higher conversion rates, thereby informing future campaigns.
Cohort analysis is also an important technique, particularly in customer segmentation. This method groups customers by shared characteristics and tracks their behavior over time, offering deep insights into how specific actions, like promotions, affect customer retention and loyalty. The ability to analyze the data across different cohorts allows companies to refine their strategies and improve overall performance.
Type of Data Analysis Technique
There are several types of data analysis techniques used in businesses today, each offering unique advantages based on the nature of the data being analyzed. Descriptive analysis focuses on summarizing past data, helping businesses get a snapshot of their performance. Diagnostic analysis seeks to identify the reasons behind specific outcomes, allowing companies to optimize their operations.
More advanced techniques like predictive analysis and prescriptive analysis are now at the forefront. Predictive analysis uses historical data to forecast future trends, while prescriptive analysis goes a step further by recommending actions to optimize outcomes based on the data insights. For example, predictive models might suggest increased production of a particular product during peak seasons based on sales data, while prescriptive models could recommend specific resource allocations to maximize efficiency during that period.
Advanced Data Analytics: Data Mining
One of the most powerful tools in advanced data analytics is data mining. This technique involves extracting meaningful patterns from vast datasets, which often include both structured and unstructured data. Data mining allows businesses to discover hidden relationships within the data that might not be immediately obvious through traditional analysis methods.
In practice, data mining is used to identify customer purchase patterns, predict trends in consumer behavior, and optimize marketing efforts. For example, a retailer might use data mining techniques to analyze sales based on historical data and identify key products that drive customer loyalty. From there, predictive models can be developed to forecast future sales trends, allowing businesses to adjust their inventory and marketing strategies accordingly.
By combining data mining with other data analysis methods, organizations can optimize their processes and enhance decision-making capabilities. Data analysis can help companies make better use of their resources, whether by improving customer targeting, optimizing supply chains, or reducing operational inefficiencies.
Google Data Analytics
For many businesses, especially small to mid-sized companies, Google Data Analytics offers a powerful yet accessible solution for managing and analyzing data. Google’s suite of tools, such as Google Analytics, Data Studio, and BigQuery, allows companies to track website performance, analyze customer behavior, and build dashboards that visualize key performance indicators (KPIs).
Google Data Analytics tools also support cohort analysis, enabling businesses to track the behavior of customer segments over time and optimize their marketing strategies. With data visualization features built into these platforms, decision-makers can quickly grasp insights from complex datasets, making Google Data Analytics a crucial part of any modern data strategy.
Furthermore, these tools facilitate exploring your data by offering interactive dashboards that update in real-time. This empowers businesses to make data-driven decisions on the fly, ensuring they remain agile in a rapidly changing market environment. Additionally, Google Data Analytics courses are widely available, helping individuals build their skills in data analysis and pursue lucrative careers in the field of data analytics.
Embracing Data Analytics for Future Success
data analytics in 2024 is more than just a business tool—it’s a fundamental driver of strategic decision-making. By embracing AI, machine learning, and advanced data analysis techniques, businesses can transform raw data into meaningful insights that foster innovation, efficiency, and growth. Whether it’s through predictive analysis, data visualization, or spatial analysis, the ability to analyze data effectively will continue to be a competitive differentiator in the years to come.
As organizations continue to invest in data science and data analytics, the future of business will be shaped by those who can harness the power of data to make smarter, faster, and more informed decisions.
In conclusion, data analysis is the process that transforms raw data into meaningful insights, allowing businesses to make informed decisions. The process of data analysis involves various stages where data is collected, cleaned, and analyzed to derive actionable insights. Effective analysis requires the right analysis tools and methodologies to ensure that the data is in order and ready for interpretation.
Data analysis is the practice of utilizing various data analysis techniques to uncover patterns and trends. Whether through data science in R or other programming languages, this practice helps professionals analyze your data to optimize performance. Visualization and data representation also play a crucial role in helping stakeholders grasp the insights quickly and clearly.
As the future of data continues to evolve, the demand for skilled data professionals remains high, with competitive salaries for data analysts. Diagnostic analysis determines the root causes of issues, and analysis to optimize operations often leads to better decision-making. Ultimately, analysis is often the key to understanding business challenges, and insights from data drive innovation and growth in all sectors.
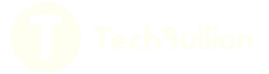