Integrating artificial intelligence (AI) into healthcare promises immense benefits, such as enhanced diagnostics, treatment optimization, and streamlined processes. At the same time, it raises critical ethical concerns.
Robust practices are required to protect sensitive patient data from breaches or misuse. Bias and a lack of fairness in AI algorithms can perpetuate healthcare disparities if not mitigated. Maintaining transparency in AI decision-making processes is essential for patient autonomy and informed consent. It’s imperative for societal implications such as accessibility barriers and erosion of public trust to be proactively addressed. Solutions to these issues include encryption and anonymization of data, diverse training data, ongoing monitoring, and strategies promoting equitable access and ethical marketing. Overall, ethical frameworks that balance innovation and patient welfare are crucial as AI transforms healthcare delivery. This demands a collaborative approach to ensure accuracy, safety, ethical integrity, user-centric design, regulatory compliance, and patient trust.
AI in healthcare
AI is not a new concept. It was first described in 1950, but its use was limited due to the constraints of early models. Improvements in technology, including the advent of deep learning, resulted in more widespread use. Now, AI systems can analyze complex algorithms and allow healthcare users to apply AI to clinical practice.
Today, some of the most common applications of AI within the healthcare industry include using AI algorithms to help interpret X-rays, MRIs, and CT scans. Providers use AI-powered tools to make evidence-based decisions by analyzing patient data and medical literature and enlist AI models to predict patient outcomes, disease progression, and risk of readmission. AI also accelerates the identification of potential drug candidates and enhances clinical trial processes. Geisinger, a Pennsylvania healthcare organization, deploys AI technology for numerous tasks, including enabling patients and members to manage their health better. On a larger scale, the Mayo Clinic uses AI to help predict and diagnose serious or complex heart problems.
Ethical concerns about AI and adverse outcomes
While AI’s contributions to healthcare and its promise are evident, there are also ethical concerns, including:
- Privacy and data security. Collecting and analyzing vast amounts of sensitive health data raises concerns about data breaches and patient privacy. According to the World Economic Forum, mistrust among doctors and the public is among the most significant barriers to adopting AI in healthcare. Data breaches and unethical use of patient information can erode trust in healthcare institutions and providers.
- Algorithmic bias. The AI system may cause or worsen existing healthcare disparities if the algorithm is based on biased datasets. For instance, a Health and Human Rights Journal article cites an example where an algorithm used to distinguish malignant and benign moles was trained on fair-skinned patients. This algorithm might fail to diagnose moles in people of color properly. Another cited example comes from an algorithm that was deployed to detect cardiovascular diseases that “might under-perform on women because most of the medical training data concerns men.”
- Lack of transparency. When processes and decision-making criteria used in AI algorithms are not clearly explained or accessible, it can be difficult for users to understand how the algorithm arrives at its conclusions, what data it was trained on, and whether any biases or errors might impact its outputs. This can lead to mistrust, lack of accountability, and ethical challenges.
- Patient-informed consent. Patients may not completely understand how AI is used in their care, which may call informed consent into question. It’s important that healthcare organizations pay attention to existing and emerging regulations to ensure compliance.
- Accountability and responsibility for AI-related processes and outcomes. It is imperative for organizations to establish well-defined roles and responsibilities and communicate those standards throughout the enterprise. It is also essential to ensure AI-driven processes are correctly implemented, regularly reviewed, and revised to optimize outcomes and mitigate potential challenges.
- Job displacement. There is concern that the automation of certain healthcare tasks may lead to job losses or role changes for some healthcare workers. Yet, human oversight and critical thinking are essential to maximizing the benefits of AI.
Best practices for improving healthcare using AI
Addressing data security is paramount to improving healthcare using AI. Some of the best practices that will improve healthcare using AI are implementing robust data encryption by using strong encryption methods for data at rest and in transit. Adopting a zero-trust security model that verifies every user and device attempting to access the network, regardless of location, enhances data safety and reduces the possibility of a breach. Implementing multifactor authentication that requires several verification forms to access sensitive data and systems is vital to security. Employing secure backup and recovery systems to ensure data can be quickly restored if a breach or system failure occurs may reduce the breach’s impact. Conducting regular security audits by performing frequent assessments to identify and address the vulnerabilities in systems and processes may prevent mishaps.
It’s important to address the algorithmic bias that can exacerbate inequities in race, ethnic background, religion, disability, gender, sexual orientation, and other factors. To ensure fair representation, it’s essential to include diverse populations in training data. Frequently assessing AI models to identify and address potential biases is also critical. Interdisciplinary development teams that include ethicists, social scientists, and healthcare professionals from various backgrounds can guarantee robust assessments. Once designed, ongoing monitoring of system outputs is necessary to detect and address emerging biases. Documenting and disclosing the factors considered in the AI decision-making process will increase trust in the algorithm design.
With cyberthreats and their implications continuing to plague organizations of every size and sector, data privacy is another concern, especially in healthcare. A best practice to improve privacy is anonymizing data when possible. It is vital to remove personally identifiable information when it is unnecessary for analysis or care. In addition, evaluate the privacy implications of new technologies or processes before implementation.
When developing, implementing, and evaluating AI initiatives in healthcare, it is important to involve all existing and potential stakeholders. For example, hospital and healthcare administrators are responsible for approving, funding, and overseeing the strategic implementation of AI, managing resources, and aligning initiatives with organizational goals. Providers, on the other hand, share insights into clinical needs, validate AI models, and guarantee the technology aligns with patient care practices. Further, the data scientists and AI developers design, develop, and refine AI algorithms, confirming their accuracy, reliability, and suitability for various use cases. IT and technical support teams integrate AI systems into existing infrastructure, ensuring seamless operation and data security. Regulatory and legal experts are vital to address all regulatory, ethical, and legal standards. Patients and patient advocacy groups can offer valuable feedback on AI tools, helping to ensure they are patient-centric and transparent. Additional stakeholders may include healthcare payers and insurers as well as government and policymakers who shape the responsible adoption of AI in healthcare. This collaborative and holistic approach creates AI initiatives that are well-rounded, effective, and aligned with the needs and values of the healthcare ecosystem.
The future of AI and the healthcare industry
Integrating AI and other emerging technologies can further enhance the capabilities of healthcare organizations. For instance, AI-driven analyses of genetic, lifestyle, and environmental factors can help tailor treatments to individual patients. In addition, advanced AI models may forecast disease outbreaks, and advanced AI-powered surgical robots can be employed to perform complex surgical procedures. AI can also accelerate the development of new medications and therapies.
The use of AI in healthcare is seemingly limitless, but it must be secure and unbiased as well as engender patient and provider trust. The most effective teams are interdisciplinary and include diverse perspectives in AI development, including ethicists, social scientists, and healthcare professionals from various backgrounds. The development and improvement of AI technology aren’t simply a technical issue. They also encompass ethical issues that impact healthcare recipients—patients. Earning their confidence is critical, and by addressing AI’s issues and training patients on the use of technology, healthcare providers can alleviate concerns.
About the Author:
Cornell Anthony is a senior cloud infrastructure architect with over 11 years of professional experience. Among other accomplishments, he designed the infrastructure strategy for a LATAM e-commerce giant, optimized a Fortune 500 financial organization’s containerized infrastructure, and helped another client migrate applications with more than 100,000 users monthly to container services to facilitate global expansion. Cornell is passionate about modernization, Kubernetes, and GenAI and excels in collaborating with stakeholders to deliver value and innovation to customers. He graduated with a Master of Science degree in computer engineering from New York University and a bachelor of engineering degree in electronics and telecommunications engineering from the University of Mumbai. Connect with Cornell on LinkedIn.
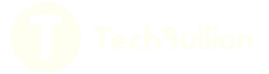