CNC machining is an important part of modern manufacturing. It is used a lot in industries like oil and gas, medicine, aerospace, and the automobile industry.
With the rise of machine learning (ML) and artificial intelligence (AI), the future of CNC machining processes is uncertain.
Based on real-time data, analytics, and deep learning, these technologies can change how a machine learns, adapts, and optimizes its output.
Implementing AI and ML in CNC Machines
Machine learning systems can look at data in detail and find places where changes need to be made.
With the development of cheap, effective sensors and data collection systems, machine learning methods can be used to keep track of the state of tools.
AI can also improve the accuracy of CNC machining by creating and analysing production data and giving the results to human workers in real time. This makes it easy to make quick changes to how the machine works, which increases its output.
AI can also figure out when CNC machine tools need to be serviced by looking at output data like how well the machine works and how long its tools last.
This foresight can lead to fewer tool failures, longer tool life, less downtime, and faster cutting, which saves money.
Getting the most out of CNC machining tasks
Optimisation of CNC machining processes has become a big focus of research in recent years, especially as data volumes grow and models get more complicated.
From the supply chain to the finished goods, incremental optimisation will be a key part of manufacturing in the future.
Optimisation of CNC machine tool processes is important for saving money and making more money per production run, which leads to higher productivity and fewer parts that aren’t working right.
Using live data from the production process, AI and machine learning can be used to automate optimisation even more. By optimising the machining settings, this makes machined parts more accurate and increases the speed at which parts can be made.
Using machine learning and genetic algorithms, a generalised method for optimising multi-response machining processes has been created. This method is used to improve machining conditions and performance.
Using a Multi-Objective evolutionary algorithm during CNC machining processes can improve how fast parts are made and how well they work.
Machine learning is also used to improve parallel metaheuristics in CNC cutting on the shop floor, which makes the process of making parts more efficient.
By using machine learning in the process of optimising CNC machine tools, component production can be more stable and the risk of unexpected failure can be reduced.
When turning Ti-6Al-4 V, the best choices for cutting are found by using the response surface method and machine learning. The Nelder–Mead simplex method is an example of a machine learning method that is used to improve machining variables in end milling tasks.
ML and AI can be used in CNC machining operations to get optimised machining parameters based on the changeable conditions and parameters of the workpiece and machining parameters. This makes the process of making parts more productive.
AI and machine learning to predict tool wear in CNCs
Because they can handle non-linearity, machine learning technologies like Artificial Neural Networks (ANNs) are being used more and more to predict tool wear in complex processes.
Modern sensors and AI are used to predict and stop bad things from happening to cutting tools and machinery. This helps with tool condition tracking and machine tool diagnostics.
Tool condition monitoring, also called Tool health monitoring, is becoming more popular because it helps cutting tools last longer during machining. Strategies for checking the state of tools are usually grouped into “Offline/Direct methods” and “Online/Indirect methods.” Direct methods are the best way to look at and learn from complex mistakes, but they can’t be used for machine learning.
In the turning process, adaptive neuro-fuzzy inference systems and other online tool wear prediction systems are used to provide advanced tool wear tracking systems. To get an accurate estimate of tool wear when milling complex components, approaches based on deep learning are used to track tool wear.
Advanced neural network systems are being made to correctly predict how cutting tools will wear based on the specific amount of cutting energy used during CNC machining.
Conclusions about CNCs, Machine Learning, and AI
In conclusion, machine learning and artificial intelligence are becoming more and more important in predicting tool wear in CNC machining operations. This is because they can now analyse and improve the life of cutting tools during the chip formation process of different workpiece materials and machining parameters.
Sources
https://www.sciencedirect.com/science/article/pii/S2667344423000014
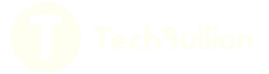