Research is one of the most important functions across fields in an age wherein data rules. From market analysis and scientific studies to financial modeling and content generation, organizations and individuals alike are always on the lookout for modes of speeding up the research.
In the traditional sense, research has demanded a lot of time, in hours of sorting and funneling any data, which may in turn act as possible bottlenecks laden with potential for error. The AI agents can be powerful tools to change the entire research with automatic execution of these very tasks with little human effort.
The AI agents use machine learning, natural language processing, and various forms of data integration to carry out complicated analyses of research tasks. But are they going to be efficient and capable of automating research? You shall look into AI agents in research workflows: their pros, cons, and the future of information discovery.
AI Agents in Automated Research Activities
Perhaps the most monotonous phase of any research is the collection of relevant data from different sources. AI agents can scrape the web, dig through databases, and pull together information from structured and unstructured sources in seconds. These agents can use integrations into numerous APIs and cloud-based infrastructure to gather an immense amount of data passively.
For instance, companies using machine-learning-enabled research assistants such as Datagrid AI can automatically pull data on market trends, competitor pricing, and customer feedback from numerous sources, making the process less tedious and hastening the decision-making process.
-
Filtering and Organizing Data
AI agents can also clean and structure the data collected making analysis easy. This is to say, removing duplicates, organizing information, and filtering out noise. The data is then used by machine learning models to improve search results so users can find the best, most accurate insights they need.
AI-powered tools such as Google’s Gemini or OpenAI’s ChatGPT can scan and summarize many research papers to present the most valuable information without a researcher needing to read every paper.
-
Text Analysis
AI agents with natural language processing capabilities can comprehend and analyze text-based information, enabling them to read research papers, legal documents, news articles, and reports. They can process large volumes of data much faster than a human researcher can identify patterns, trends, and findings that would take human researchers days or weeks to discern.
For example, AI-driven research platforms can summarize complex reports, extract key metrics, and conduct sentiment analysis on large datasets to assist researchers in deriving meaningful conclusions.
-
Generating Research Insights
AI-enabled agents can analyze those data points and transform them into insights. These agents track correlations, recognize trends, and propose potential new avenues of inquiry. It allows businesses to make data-backed actions when powered by AI-enabled analytics.
Financial analysts can also use artificial intelligence tools to study stock market patterns, forecast investment risks, or generate reports without any, or very little, human involvement.
-
Automating Literature Reviews
Many academic and scientific research begins with literature reviews. AI agents can facilitate the process, scouring thousands of articles, summarizing applicable findings, and suggesting references that relate to a researcher’s topic of interest.
Platforms like Semantic Scholar or Elicit use AI to pull relevant insight out of research papers and save academics precious time and effort.
-
Seamless Workflow Integration
AI research agents are working in the background for businesses or academics to get real-time updates and alerts that can be built into existing workflows. These AI agents are effectively plugging the gap between research and real-time application.
For example, companies working with Datagrid AI can integrate their AI agents with Microsoft Teams or Slack, allowing them to receive research updates and recommendations in their collaboration hubs.
-
Dealing with Repetitive Research Tasks
Academia’s AI agents excel at handling foundational research tasks such as data entry, citation formatting, and stats. This is to free human researchers from day-to-day routine operations to make them think at a higher level of critical thinking and intuition.
The Advantages of Using AI Agents for Research
-
Speed and efficiency
AI agents can look through vast quantities of information within a matter of mere minutes, thereby greatly reducing the time of the research process.
-
Accuracy and reliability
AI assists agents in machine learning by decreasing human errors and producing more accurate data that leads to more reliable research.
-
Cost-Effectiveness
Certain duties in research could be automated, therefore bringing the smaller size of research teams and lessening overheads in industries and faculties.
-
Enhanced Productivity
AI takes care of repetitive, data-heavy tasks, thus leaving researchers with time for critical analysis and high-level strategic decision-making.
-
Scalability
Organizations dealing with such vast datasets benefit from the fact these AI-powered research tools can tackle growing volumes of data without being resource-intensive.
Challenges and Limitations
Even with their advantages, AI agents have challenges:
-
Data Bias and Inaccuracy
AI models train on data until October 2023. Training data should be diverse and data quality should be ensured to mitigate biases.
-
Lack of Human Judgment
Even though it can analyze data, it does not have human intuition or context, both of which are necessary for understanding complex research topics.
-
Ethical Concerns
Ethics Concerns: Working with AI on research raises ethical questions, such as data privacy, plagiarism, and misinformation. Researchers need to keep in mind ethical compliance in AI-generated content.
-
Dependence on AI Models
Data-only effective research is based on AI models. Due to the ever-evolving nature of AI, companies, and researchers must regularly update and improve their AI agents to stay relevant and provide accurate information.
The Future of AI Agents in Research
Some of these are the future of AI-powered research, such as the developments in multi-modal AI models, enhanced NLP capabilities, and improved data-processing techniques. Machine learning will evolve to offer almost intuitive AI agents that assist researchers with everything from raw data to forecasting and real-time decision support.
AI agents will become increasingly integrated into industries such as healthcare, finance, marketing, and academia, which will lead to more automated, fair, and broad research.
Conclusion
Inference and study are the objectives of articles, and AI specialists are revolutionizing how study is accomplished, automating time-feeding work and increasing the precision of data-driven insights.
Researchers and businesses can work smarter and faster with AI-supported data collection, filtering, text analysis, and insights. Although AI does not substitute human intuition and critical thinking, it can act as a powerful assistant, leading to faster, more intelligent, and more comprehensive research.
As AI technologies progress further, their potential in automating research will amplify, establishing it as an essential asset for experts from diverse backgrounds. AI agents not only present a new approach to improving research in general, but they also transform the way this information is obtained, studied, and applied to the real world.
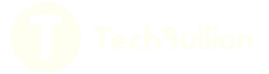