In a world where automation once threatened to replace human labor, a new frontier is taking shape—one where artificial intelligence (AI) doesn’t substitute human expertise but strengthens it. Gaurav Bansal, a forward-thinking researcher, has articulated a visionary framework for human-AI collaboration, delving deep into architectural models and monitoring systems that promise to reshape enterprise operations. A scholar with a background in engineering and innovation, he brings to light the transformative potential of collaborative intelligence.
The Blueprint: Designing for Seamless Integration
Successful collaboration between humans and AI begins with intelligent system design. Unlike rudimentary automation tools, modern frameworks focus on preserving context throughout workflows. This means systems must remember not just the data, but the nuanced reasoning behind decisions. Context preservation mechanisms, as highlighted, utilize semantic networks and data layering to ensure continuity and accuracy. These networks allow for the retention of critical information across complex tasks, adapting dynamically to shifts in objectives or personnel without losing thread.
Smooth Transitions: The Role of Handoff Protocols
At the heart of collaborative systems lies the challenge of seamless interaction. When an AI hands off a task to a human—or vice versa—there must be more than just a notification. Structured handoff protocols ensure that transitions are smooth, with all contextual data intact. These include confidence levels, task urgency, and detailed state information, enabling the human operator to quickly orient and act. Adaptive triggers also help decide when a handoff should occur, ensuring tasks are directed to the most capable handler based on real-time insights.
Adapting on the Fly: Intelligent Workflow Engines
Flexibility is essential in dynamic business environments. Adaptive workflow engines enable real-time changes in task routing based on resource availability and contextual urgency. These engines combine rule-based logic with statistical models to continuously optimize performance. They not only monitor current workflows but also anticipate potential disruptions and adjust accordingly. As a result, organizations benefit from both consistency and agility, especially in environments where demand can shift rapidly.
Talking the Talk: Natural Language Interfaces
Bridging the gap between machine logic and human cognition is another key innovation. Natural language interfaces have evolved to provide tailored experiences, adjusting output based on user role and task complexity. Instead of overloading users with data, these interfaces reveal information progressively, offering more detail as needed. They help human workers engage with AI systems confidently, especially in high-stakes or technical environments, where precision and clarity are paramount.
Keeping Watch: Monitoring Systems for Collaborative Performance
Integration is only the first step; sustained success depends on robust monitoring. Real-time dashboards and distributed sensor networks track both technical and human performance. This data feeds into analytics engines capable of identifying performance trends, potential bottlenecks, and areas for improvement. Importantly, these systems are designed to avoid cognitive overload—only surfacing insights that are relevant, timely, and actionable.
Analytics That Learn and Adapt
Analytical tools in these collaborative frameworks do more than just report—they learn. By combining structured data with unstructured interactions, analytics engines can detect subtle shifts in performance or collaboration dynamics. These insights are not just retrospective but predictive, enabling proactive optimization. Over time, these systems become smarter, adapting to new usage patterns and user behaviors.
Smart Routing: The Rise of Adaptive Algorithms
In these frameworks, not all tasks are created equal. Adaptive routing algorithms evaluate each task in real-time, assigning it based on complexity, workload, and context. Unlike traditional logic trees, these algorithms incorporate machine learning models that evolve with historical data. This ensures the most effective use of human and machine resources, improving both speed and reliability of decision-making.
Seeing the Big Picture: Real-Time Dashboards
Visibility into complex workflows is critical for strategic oversight. Performance dashboards have advanced from simple reports to intelligent, role-based tools that highlight trends and suggest interventions. These visual systems offer tailored insights depending on whether the user is a technician, analyst, or executive, enhancing coordination and response strategies across departments.
Cross-Industry Impact: A Framework for All
While the paper outlines a wide variety of potential use cases, the underlying architectural principles are applicable across industries. Whether in service delivery, diagnostics, or advisory roles, collaborative systems are proving that blending AI with human judgment yields stronger, more adaptive outcomes. The key lies in building systems that recognize and respect the distinct strengths each brings to the table.
In conclusion, Gaurav Bansal’s work makes one thing clear—true progress in artificial intelligence won’t come from replacing humans, but from working alongside them. His proposed models offer a scalable, ethical, and efficient blueprint for organizations looking to unlock the next level of performance. By embracing systems that are not just smart but also collaborative, enterprises can craft a future where human creativity and machine precision thrive together.
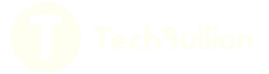