“In an industry where data is king, embracing AI and advanced analytics is no longer optional for private equity firms that want to stay ahead.”
Private equity (PE) firms face unprecedented pressure to make faster and more accurate investment decisions in today’s hyper-competitive landscape. The traditional reliance on intuition, financial ratios, and qualitative analysis is no longer sufficient in a world where speed, precision, and data-driven insights define success. As data continues to grow exponentially in both volume and complexity, the integration of artificial intelligence (AI) and data analytics has emerged as a transformative force in the PE industry.
AI and data analytics are redefining how PE firms operate, providing tools that not only enhance decision-making but also create competitive advantages. These technologies enable firms to sift through massive datasets quickly, identify patterns, and make informed predictions about future outcomes. This shift is not just about efficiency; it’s about fundamentally changing the way private equity firms approach investments, risk assessment, and portfolio management.
As we explore the role of AI and data analytics in shaping smarter private equity investments, it becomes clear that these technologies are no longer a luxury but a necessity. This article will look into the traditional methods of private equity, the current impact of AI, the enhancement of due diligence processes through data analytics, practical steps for implementation, and the future of private equity in an AI-driven world.
The Traditional Approach to Private Equity
Private equity has traditionally relied on a combination of intuition, experience, and financial metrics like EBITDA multiples and debt-to-equity ratios to make investment decisions. This conventional approach, though effective for many years, primarily depends on historical data and subjective judgment, which limits its ability to capture the full picture. The industry’s decision-making processes often involved labor-intensive due diligence, focusing on reviewing financial statements, conducting market research, and interviewing company executives. However, these methods are increasingly being challenged by the demands of a fast-paced, data-driven world.
The major drawback of the traditional private equity approach lies in its dependence on backward-looking data, which may fail to account for future market disruptions, emerging trends, or shifts in consumer behavior. For instance, when evaluating a potential acquisition in the retail sector, traditional methods might miss critical factors such as the growing consumer shift towards e-commerce. This can result in overvaluing a company based on past performance while underestimating future risks that could impact its profitability and market position.
Given these limitations, there is a growing recognition of the value that AI and data analytics can bring to private equity. These tools can offer more accurate, forward-looking insights that traditional methods may overlook, thereby improving the ability of firms to predict future performance and avoid potential pitfalls. By incorporating advanced data analytics into their decision-making processes, private equity firms can better navigate the complexities of today’s rapidly changing market landscape, making more informed and strategic investments.
The Role of AI in Private Equity Today
AI is revolutionizing private equity by automating repetitive tasks, analyzing vast datasets, and delivering faster and more accurate insights. The ability of AI to process and interpret large volumes of data in real time has the potential to transform every aspect of the PE investment lifecycle, from deal sourcing and due diligence to portfolio management and exit strategies.
One of the most significant contributions of AI to private equity is in the realm of predictive analytics. By leveraging machine learning algorithms, AI can analyze historical data and identify patterns that are not immediately apparent to human analysts. This allows PE firms to make more informed predictions about the future performance of potential acquisition targets.
For example, AI-driven tools can analyze financial statements, market data, and even unstructured data such as news articles and social media posts to assess the growth potential of a company. These insights can help PE firms identify opportunities that may have been overlooked using traditional methods. Additionally, AI can flag potential risks, such as market volatility or changes in regulatory environments, that could impact the success of an investment.
Real-world examples of AI implementation in private equity are becoming increasingly common. For instance, Bloomberg has introduced AI-powered tools that provide contextualized financial insights and summaries of earnings calls. These tools allow investors to quickly grasp the key takeaways from complex financial reports, improving the speed and accuracy of investment decisions.
Similarly, S&P Global has developed AI-driven platforms that analyze vast amounts of financial data to deliver insights into market trends and company performance. These platforms enable PE firms to identify investment opportunities more quickly and with greater precision. Private market intelligence companies are also leveraging AI to provide real-time data and analytics, helping firms stay ahead of the competition.
AI is also playing a crucial role in automating routine tasks such as data collection, report generation, and compliance monitoring. By reducing the time and effort required for these tasks, AI allows PE professionals to focus on higher-value activities, such as strategic decision-making and relationship-building.
Enhancing Due Diligence with Data Analytics
Due diligence is a critical component of the private equity investment process, and data analytics is elevating this process to new heights. By leveraging advanced analytics, PE firms can gain deeper insights into market conditions, competitors, and potential synergies, ultimately leading to more informed investment decisions.
A prime example of how data analytics can enhance due diligence is the evaluation of growth potential in the cybersecurity industry. In this case, a PE firm used predictive modeling and competitive benchmarking to assess the future performance of a cybersecurity company. By analyzing market trends, customer retention rates, and the competitive landscape, the firm was able to uncover hidden value and potential risks that were not immediately apparent through traditional analysis.
Predictive modeling is one of the most powerful tools in data analytics, allowing firms to forecast future performance based on a wide range of variables. In the context of private equity, predictive modeling can be used to evaluate the growth potential of a target company, assess the impact of market conditions, and identify potential exit strategies. For example, a PE firm considering an investment in a technology startup could use predictive modeling to forecast the company’s revenue growth based on factors such as market demand, competition, and technological advancements.
Competitive benchmarking is another valuable technique that data analytics brings to the table. By comparing a target company’s performance against industry peers, PE firms can identify areas of strength and weakness, as well as potential opportunities for growth. This information is critical for making informed investment decisions and developing strategies for post-acquisition value creation.
Customer retention analysis is also becoming increasingly important in due diligence. By analyzing customer data, PE firms can gain insights into the loyalty and satisfaction of a company’s customer base. High customer retention rates are often indicative of a strong business model and can be a key driver of future growth. Conversely, low retention rates may signal underlying issues that need to be addressed before making an investment.
The use of data analytics in due diligence extends beyond the acquisition phase. Once a deal is completed, data analytics can continue to play a crucial role in portfolio management. By monitoring key performance indicators (KPIs) and analyzing trends, PE firms can make data-driven decisions to optimize the performance of their portfolio companies. This ongoing analysis helps to identify areas for improvement and ensures that the investment is on track to achieve its desired outcomes.
Practical Steps for PE Firms to Implement AI and Analytics
For private equity firms looking to harness the power of AI and data analytics, a strategic and systematic approach is essential. Implementing these technologies requires careful planning and execution to ensure that they deliver the desired results.
Define Goals
The first step for any PE firm looking to adopt AI and data analytics is to define clear and measurable goals. These goals will vary depending on the firm’s specific needs and objectives. For example, a firm may want to use AI to improve deal sourcing by identifying high-potential investment opportunities more quickly. Alternatively, the goal may be to enhance portfolio management by using predictive analytics to monitor the performance of portfolio companies.
It’s important for firms to prioritize their goals and focus on the areas where AI and data analytics can have the most significant impact. This focus will help to guide the selection of tools and methodologies and ensure that resources are allocated effectively.
Data Collection
Once goals are defined, the next step is to collect and organize the data needed for analysis. Data is the foundation of AI and analytics, and the quality of the data will directly impact the accuracy and reliability of the insights generated.
PE firms should focus on collecting both internal and external data. Internal data may include financial statements, operational metrics, and customer data from portfolio companies. External data sources can include market research, industry reports, and macroeconomic indicators. The key is to build robust datasets that provide a comprehensive view of the investment landscape.
Data quality is also a critical consideration. Poor-quality data can lead to inaccurate analysis and flawed decision-making. Firms should implement processes to clean and validate data, ensuring that it is accurate, complete, and up-to-date.
Tech Adoption
With data in hand, the next step is to adopt the right technology tools and methodologies. There are a wide range of AI and analytics platforms available, each with its own strengths and capabilities. Firms should carefully evaluate these tools to determine which ones are best suited to their needs.
Predictive analytics platforms are particularly valuable for private equity firms, as they can help to forecast future performance and identify potential risks and opportunities. Machine learning models are also useful for analyzing complex datasets and identifying patterns that are not immediately apparent.
In addition to selecting the right tools, firms should also consider the infrastructure needed to support AI and analytics initiatives. This may include cloud computing resources, data storage solutions, and software platforms for data visualization and reporting.
Team Building
Successful implementation of AI and data analytics requires a cross-functional team that combines financial expertise with data science capabilities. PE firms should invest in building teams that include both seasoned investment professionals and skilled data scientists.
Financial experts bring a deep understanding of the investment process and the specific challenges faced by private equity firms. Data scientists, on the other hand, bring the technical skills needed to analyze data, develop predictive models, and interpret the results.
Collaboration between these two groups is essential for translating data-driven insights into actionable investment strategies. Firms should foster a culture of collaboration and continuous learning, ensuring that team members from different disciplines work together effectively.
Pilot Projects
Before rolling out AI and analytics initiatives across the organization, it’s advisable to start with pilot projects. These projects allow firms to test new technologies and methodologies on a smaller scale, minimizing risk and providing valuable learning opportunities.
Pilot projects should be carefully selected to align with the firm’s strategic goals. For example, a firm might start by using predictive analytics to evaluate a single acquisition target or by implementing a machine learning model to improve the accuracy of financial forecasting.
By starting small and learning from pilot projects, firms can refine their approach and build the confidence needed to scale AI and analytics initiatives across the organization.
Challenges and Considerations
While the benefits of AI and data analytics are clear, implementing these technologies in private equity is not without its challenges. Firms must be prepared to navigate potential obstacles and develop strategies to overcome them.
Data Quality Issues
One of the biggest challenges in implementing AI and analytics is ensuring the quality of the data being used. Inaccurate or incomplete data can lead to flawed analysis and poor decision-making. PE firms must invest in processes and technologies to clean, validate, and manage data effectively.
Data quality issues can arise from a variety of sources, including inconsistent data entry, outdated information, and data silos within the organization. To address these issues, firms should establish data governance policies and invest in tools for data integration and management.
Internal Resistance to Change
Another common challenge is internal resistance to change. AI and data analytics represent a significant shift from traditional methods, and some team members may be hesitant to embrace these new technologies. This resistance can be driven by a fear of job displacement, a lack of understanding of the technology, or a preference for traditional approaches.
To overcome resistance, firms should focus on education and communication. Leadership should clearly articulate the benefits of AI and analytics and provide training to help team members understand how these technologies can enhance their work. It’s also important to demonstrate the ROI of AI initiatives, showing how they can lead to better investment outcomes and drive business growth.
Leadership Hesitation
Leadership hesitation can also be a barrier to adopting AI and analytics. Senior executives may be reluctant to invest in new technologies without a clear understanding of the potential benefits and risks. This hesitation can slow down the implementation process and limit the firm’s ability to capitalize on AI-driven insights.
To address this challenge, it’s important to build a strong business case for AI and analytics. This should include a clear explanation of the expected ROI, supported by data and case studies. Leadership should also be encouraged to take a long-term view, recognizing that AI and analytics are strategic investments that will deliver value over time.
Fostering a Data-Driven Culture
Finally, firms must work to foster a data-driven culture. This involves not only adopting new technologies but also changing the way the organization thinks about data and decision-making. A data-driven culture encourages team members to rely on data and analytics to inform their decisions, rather than relying solely on intuition or experience.
To build a data-driven culture, firms should invest in training and development, ensuring that all team members have the skills and knowledge needed to use AI and analytics effectively. Leadership should also set the tone by modeling data-driven decision-making and rewarding teams for using data to drive business outcomes.
The Future of Private Equity
As AI and data analytics continue to evolve, their impact on private equity is expected to grow even more profound. In the near future, we can expect to see several key trends shaping the industry.
Deal Flow Automation
One of the most significant changes will be the automation of deal flow. AI-powered platforms will enable PE firms to identify and evaluate potential investment opportunities more quickly and with greater accuracy. These platforms will use machine learning algorithms to analyze vast amounts of data, flagging high-potential targets and providing insights into their future performance.
AI-Driven Portfolio Management
AI will also play a critical role in portfolio management. By continuously analyzing data from portfolio companies, AI tools will provide real-time insights into performance, helping firms to make proactive decisions to optimize value. This will include everything from identifying opportunities for cost reduction to predicting the impact of market changes on portfolio companies.
Enhanced Post-Merger Integrations
Post-merger integration is another area where AI is expected to have a significant impact. AI-driven tools will help firms to identify synergies between merged companies, optimize integration processes, and monitor progress in real time. This will lead to smoother and more successful integrations, ultimately driving higher returns.
More Precise and Actionable Insights
As AI technology continues to advance, the insights generated will become even more precise and actionable. This will enable PE firms to develop more effective investment strategies, mitigate risks more effectively, and achieve higher returns. AI will also facilitate more personalized and tailored investment approaches, allowing firms to better meet the unique needs of their investors.
The New Normal
Ultimately, the adoption of AI and data analytics will become the new normal in private equity. Firms that fail to embrace these technologies risk falling behind their competitors and missing out on valuable opportunities. Those that do embrace AI will be well-positioned to thrive in an increasingly complex and data-driven world.
Conclusion
AI and data analytics are transforming the private equity industry, offering powerful tools to enhance decision-making, optimize portfolio management, and drive higher returns. For PE firms, adopting these technologies is not just an option—it’s a necessity in today’s fast-paced and competitive market.
As we move into the future, the firms that successfully integrate AI and analytics into their investment strategies will be the ones that lead the industry. By defining clear goals, collecting and managing high-quality data, adopting the right technologies, and building cross-functional teams, PE firms can harness the power of AI to make smarter, more informed investment decisions.
The time to act is now. Private equity firms that begin exploring and implementing AI and data analytics today will be the ones best positioned to capitalize on the opportunities of tomorrow. Embrace the future of private equity, and let AI and data analytics guide you to smarter, more successful investments.
Author: Kirill Iaroshenko (Management Consultant, Expert in Financial Services and Private Equity Industries and AI implementation)
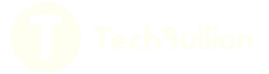