In today’s era of digital transformation, advancements in artificial intelligence are playing a crucial role in overcoming long-standing communication barriers. One such advancement is presented in the research by Kranti Kumar Appari, co-authored by M. Amru and S. Pothalaiah, which explores a deep learning-based system for real-time sign language detection. Their study integrates computer vision and machine learning to create a more accessible and inclusive communication platform for individuals with hearing impairments.
Smart Vision: From Gesture to Meaning
At the core of this system lies the use of a Convolutional Neural Network (CNN), a powerful algorithm adept at analyzing visual inputs. The method begins with a webcam capturing hand gestures in real time. These visuals are then refined using computer vision techniques like hand tracking and background elimination. By isolating key landmarks such as fingertips and knuckles the system gains an accurate outline of the hand’s posture and movement, essential for understanding sign language. The result is a smooth transition from physical gesture to digital interpretation.
Learning the Language of Hands
To effectively train the CNN model, the research team utilized a comprehensive dataset combining a publicly available sign language repository with a custom-built collection of hand gesture images. This hybrid approach enhanced the model’s adaptability to diverse real-world scenarios. By incorporating both British and American Sign Language signs, the system achieved greater linguistic versatility, enabling broader application. To further strengthen performance, dynamic preprocessing techniques were implemented to normalize variations in lighting and background conditions. These strategies ensured that the CNN model could accurately interpret gestures in various environments, making it more reliable for real-time sign language recognition tasks.
Why Deep Learning Wins
While traditional models like K-Nearest Neighbors (k-NN) offer simplicity, CNNs outperform them when complexity and scale are introduced. The system’s training was enhanced through backpropagation, which fine-tunes the model by minimizing prediction errors. This approach allows the network to learn intricate gesture patterns without manual feature selection, ultimately leading to a highly accurate recognition engine. This capability underscores the effectiveness of deep learning in understanding non-verbal communication.
Real-Time Translation: More Than Just Code
A distinguishing feature of this system is its ability to convert recognized gestures into readable text or audible speech. The immediate visual or vocal feedback assists in communication and creates a more natural user experience. This transformation is made possible by integrating the CNN with a user-friendly interface, emphasizing accessibility. The system architecture supports real-time operation, which is critical in day-to-day interactions where instant feedback is essential.
Expanding the Communication Horizon
Future prospects for this innovation are expansive. Enhancing the model to interpret dynamic gestures and full sentences can make conversations more fluid. Further integration with natural language processing would allow context-aware translations, helping bridge subtle nuances in communication. Additionally, incorporating facial expressions and lip-reading features would move the system closer to true linguistic comprehension, making it even more inclusive.
Tech That Learns and Adapts
Adaptability is key in assistive technologies. By training the model on a range of hand shapes, skin tones, and environmental settings, the system becomes more inclusive and accurate. Using tools like MediaPipe for landmark detection and frameworks like TensorFlow ensures that the model remains scalable, portable, and efficient. Moreover, by focusing on cost-effective implementation, this solution holds the potential for broader use—even in resource-constrained environments.
Vision for the Future
Beyond gesture recognition, the research hints at future integration with immersive technologies like augmented reality (AR) and virtual reality (VR). These tools could facilitate interactive learning for individuals new to sign language or offer advanced communication channels for the hearing-impaired. Mobile deployment and web-based platforms could make the tool accessible anytime, anywhere. Ensuring privacy and data security will be pivotal as the system scales, reinforcing user trust.
In conclusion, Kranti Kumar Appari’s work, along with contributions from co-authors, lays a strong foundation for next-generation assistive communication tools. By combining real-time gesture recognition with deep learning, this system empowers the hearing-impaired community and champions digital inclusivity. The innovation stands not only as a technical achievement but also as a step forward in building a more connected and understanding world.
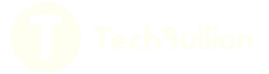