Rishab Mehra is a distinguished figure in the world of artificial intelligence and computer vision. As the CTO and co-founder of Pinnacle, Rishab brings a wealth of experience from his time at Stanford University, where he conducted groundbreaking research under the guidance of Fei-Fei Li, and his tenure at Apple, where he led significant projects in on-device machine learning.
With a track record of publications in top-tier journals like Nature and NeurIPS, as well as an impressive portfolio of 20+ patents filed during his time at Apple, Rishab has consistently pushed the boundaries of what’s possible in AI and computer vision. Today, we’ll explore his experiences, insights into the AI industry, and his vision for Pinnacle.
Your research at Stanford under Fei-Fei Li led to publications in Nature and NeurIPS. How has this academic background influenced your approach to building AI solutions at Pinnacle?
Answer Here: My research at Stanford was focussed on Computer Vision in the healthcare field. We pushed boundaries of privacy preserving Computer Vision using thermal and depth sensors. This gave me experience of how to create state of the art Computer Vision models. Computer Vision is one of the core pillars of Pinnacle, where we are using Computer Vision to create a neuro-feedback loop – truly understanding what’s going on the user’s mind, simply using the smartphone camera.
At Apple, you led fast-paced prototyping efforts for Apple Intelligence. How do you apply this experience to the development process at Pinnacle?
Answer Here: Fast-paced prototyping lends itself well to the startup world, where we are continually iterating on ideas and pitching to investors / users. While the models and architecture we are using at Pinnacle are completely different, getting experience prototyping on, on-device ML systems and Apple intelligence helped me create the initial Pinnacle system quickly since I had the core understanding of how to get models working on iPhones and how to make sure they don’t break at scale.
You’ve filed 21 patents related to ML systems at Apple. Can you discuss how this experience in innovation translates to your role as CTO at a startup?
Answer Here: Knowing the process and requirements for patents is a huge plus for a Machine Learning startup. Within the first few months of starting Pinnacle, we published a patent to protect our on-device Computer Vision based neuro-feedback system.
Pinnacle aims to be a “personal trainer for your mind.” How are you leveraging computer vision and language models to achieve this goal?
Answer Here: While we are in stealth and I can’t give a super detailed answer, the LLM system helps understand the user’s goals, just like a personal trainer at the gym would, while the computer vision system creates a neuro-feedcack loop, helping the user train, just like the equipment in the gym would.
Your experience spans from iOS security research to computer vision in healthcare. How do these diverse experiences inform your approach to mental performance technology?
Answer Here: Both these experiences help with the data management at Pinnacle. The data needed in the mental performance space is extremely private. Knowing how to keep this data anonymous and secure is a key part of Pinnacle, as it establishes trust amongst users, and both my experience with iOS security and computer vision in the Healthcare space, have taught me the best practices to keep this data secure.
At Google, you worked on automating dataset quality improvement. How does this experience influence your data strategy at Pinnacle?
Answer Here: At google, I worked on how to manage noise in datasets best. This is core to every machine learning problem, and I am applying learnings to the datasets we create at Pinnacle to train neurofeedback computer vision models.
You’ve worked in both large tech companies and startups. What are the key differences in approaching AI development in these contrasting environments?
Answer Here: These are two completely different worlds. At large companies, even though I was on a very user-facing team, small features could take months of planning and a full year to execute. This made sense, since you have a billion active users and you want to handle every edge case. At startups, we follow the 80-20 rule, we get 80% of the results in 20% of the time to get maximal value. This strategy works well at an early stage startup, since we are testing with a small group of users and iterating quickly to find product market fit.
What qualities do you look for in potential team members for a cutting-edge AI startup?
Answer Here: The key thing we look for is drive – does this person care about the problem space and are they willing to commit to bring a step function change in the world. Besides this, the skills required for each role vary, but drive is by far the most important factor.
As someone who has published in top academic journals and also built commercial products, how do you bridge the gap between research and practical applications in AI?
Answer Here: This is a hard one. Academic research can often be far removed from commercial applications, but often you can take a bunch of learnings and apply it to the real world. Large Large Models, such as ChatGPT, are a good example of this, where the paper “Attention Is All You Need” began the movement and now we live in a world, where learnings from these papers are becoming central to a bunch of AI products.
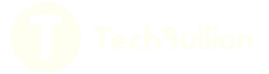