Predictive analytics is a powerful tool that helps businesses make data-driven decisions and improve their overall performance. By leveraging statistical algorithms and machine learning techniques, predictive analytics enables companies to analyze current and historical data to forecast future outcomes. With the increasing amount of data available from diverse sources, companies can now use predictive analytics to gain valuable insights into customer behavior, market trends and operational efficiency. This data-centric approach allows businesses to anticipate customer needs, optimize marketing strategies and streamline supply chain operations. Using tools like Kyvos Viz can help organizations use AI-powered predictive analytics and harness the value of their data.
In this article, we will explore the concept of predictive analytics in greater detail and examine various real-world applications across different industries.
Understanding Predictive Analytics
Predictive analytics is the use of statistical techniques, machine learning algorithms and data mining to analyze historical and current data to make predictions about future events or trends. It involves analyzing past patterns and trends in data to identify potential outcomes and make informed decisions based on those insights.
The process of predictive analytics involves collecting data from various sources, cleaning and organizing it, analyzing the data using statistical models and algorithms and interpreting the results to make predictions. These predictions are used to guide decision-making processes in business operations.
Key Components of Predictive Analytics
Explore the essential elements that drive the effectiveness of predictive analytics in today’s data-driven landscape. By combining historical data with advanced statistical techniques and machine learning, organizations can forecast future outcomes, uncover hidden trends and make more informed decisions. Below are the critical components that form the foundation of predictive analytics:
Data Collection and Preparation
The first step in predictive analytics is collecting data from various sources, including structured and unstructured data. This can include customer demographics, purchase history, social media interactions, website visits and more. Once the data is collected, it needs to be cleaned and organized to ensure accuracy in the analysis process.
Statistical Modeling
Statistical modeling involves using mathematical algorithms to analyze historical data and identify patterns or relationships between variables. These models are then used to make predictions about future events or trends.
Machine Learning
Machine learning is a subset of artificial intelligence that uses algorithms to learn from data without explicit programming. It allows computer systems to automatically improve their performance based on experience or new data, making it a crucial component of predictive analytics.
Data Visualization
Data visualization is the process of presenting data in a visual format such as charts, graphs and maps. This allows for easier interpretation of complex data sets and can help businesses identify patterns and trends that may not be apparent in raw data.
Predictive Analytics in Action: Use Cases Across Industries
Predictive analytics is a powerful tool that leverages data to forecast future outcomes and trends. By analyzing historical data and applying advanced algorithms, it provides valuable insights that can drive strategic decision-making. Its numerous applications span across different industries, including:
- Marketing and sales – Predictive analytics can help businesses optimize their marketing strategies by identifying the most profitable customer segments, predicting customer behavior and recommending personalized offers.
- Finance – In the finance industry, predictive analytics is used for credit scoring, fraud detection and risk management. By analyzing customer data, financial institutions can make more accurate decisions and reduce potential losses.
- Healthcare – Predictive analytics is used in healthcare to improve patient outcomes, optimize hospital operations and identify at-risk patients for preventive care. It enables healthcare professionals to make data-driven decisions that lead to better patient care.
- Manufacturing – In the manufacturing industry, predictive analytics can help businesses optimize supply chain operations by predicting demand and identifying potential issues in production processes.
Overcoming Challenges in Implementing Predictive Analytics
Implementing predictive analytics in a business environment presents several challenges that organizations must overcome to achieve successful outcomes. One of the primary challenges is data quality and integration. To make accurate predictions, it is crucial for businesses to have access to clean, relevant and high-quality data from various sources.
Another challenge is the need for skilled personnel who can manage and interpret complex data sets. Organizations often require data scientists and analysts who possess the expertise to build and maintain predictive models. Additionally, there is the challenge of technological infrastructure. Developing and deploying predictive analytics solutions often requires significant investments in technology to support data processing, storage and analysis. Finally, the complexity of change management cannot be overlooked.
Implementing predictive analytics often requires a cultural shift within an organization as it involves changing traditional decision-making processes and encouraging data-driven decision-making. Addressing these challenges effectively can position businesses to reap the full benefits of predictive analytics.
How Predictive Analytics Boosts Business Outcomes
By leveraging predictive analytics, businesses can gain a competitive advantage and drive better outcomes across various areas. Some of the key benefits include:
- Improved forecasting – Predictive analytics enables organizations to make accurate predictions about future events, including customer demand, market trends and sales performance.
- Enhanced customer experience – By gaining insights into customer behavior and preferences, businesses can personalize their offerings, improve customer satisfaction and build long-term relationships.
- Increased operational efficiency – With predictive analytics, organizations can optimize their operations by predicting potential issues and taking proactive measures to prevent them. This leads to cost savings and improved productivity.
- Better risk management – Predictive analytics helps businesses identify potential risks and fraud in advance, allowing them to take preventive measures and mitigate potential losses.
- Increased revenue – By utilizing predictive analytics in areas such as marketing, sales and supply chain operations, businesses can increase their revenue by targeting the right customers, optimizing pricing strategies and reducing costs.
Conclusion
Predictive analytics has become an essential tool for businesses across industries to gain valuable insights into customer behavior, market trends and operational efficiency. By leveraging historical data and advanced algorithms, organizations can make accurate predictions that guide decision-making processes and drive better outcomes. Despite its challenges, implementing predictive analytics offers numerous benefits and is a crucial element of successful business operations in today’s data-driven world.
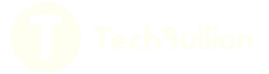