Some praise artificial intelligence (AI) as a solution to some of humankind’s toughest problems and those who demonize technology as an existential threat.
As you can see, AI has both exciting opportunities and challenges to address, and they present both opportunities and challenges. In recent years, the prospect of bias in AI has attracted much media attention.
Algorithms may be biased in several ways. Soon, AI will become an essential part of our lives, transforming human analytical abilities to the level of super-intelligent computers.
Over- or underestimation of the actual risk posed by an applicant. This means the problem isn’t in the algorithm itself but in the data that informs it.
The Problems of AI Bias
Despite AI being helpful to businesses, biased AI models often cause problems. AI cannot make an informed decision without the full picture of historical data and inaccuracies in training.
Reliability
It’s hard to overstate the accuracy of AI, but sometimes it isn’t. The model’s training is likely to be to blame for this discrepancy.
Using more light-skinned people photos and more men from the start may be necessary to increase the system’s ability to recognize light-skinned men. It will be easier for AI to make accurate judgments with more training with minority groups.
When looking at any type of AI solution, it’s always important to read the reviews online, while getting an outside perspective from other users.
Rules of humanity
When machines consider attributes that are often attributed to bias, they follow some complex rules.
Having different insurance rates for male and female drivers is perfectly acceptable and legal and results from statistically different accident rates.
Therefore, would calculating auto insurance rates partly by inferring facts about an attribute such as a driver’s religion be acceptable? Of course not.
The prohibition may not be obvious to an AI algorithm that slices enormous amounts of data in every way possible.
Evaluation of cases
The problem of bias in AI is undeniable. However, it does not follow that algorithmic bias will appear in all cases due to the underlying algorithms and data.
At least initially, this can make distinguishing apparent bias from actual bias more difficult. Allegations of AI bias ought to be taken seriously, but the accusations alone should not be enough.
Studies of AI bias must be structured to maximize the ability to perform objective analysis free of pressure to reach predetermined conclusions.
The Solutions to AI Bias
Using AI successfully requires implementing it in objective processes where bias cannot contribute to results or hiding factors that can lead to bias.
Annotate with diversity
Diverse human annotations will result in more diverse viewpoints. By doing that, you can reduce bias at the time of start-up as well as during retraining.
A global crowd of contributors can provide different perspectives and support various languages, dialects, and geographically specific content.
Uprooting discriminatory language
The language in job descriptions in many companies is discriminatory, whether accidentally or by design.
Artificial intelligence is used to analyze job descriptions for gender bias and choppy or weak language.
In addition to ensuring organizations reach a diverse audience, it will also help them strengthen their job descriptions to find the right candidates.
Recruiting blindly
Managers are often biased when making hiring decisions, even when they do not intend to do so.
Before interviewing a candidate, factors such as race, gender, and even college affiliation can affect their opinion of them.
AI can be used to automate screening and scheduling so factors such as a candidate’s name, accent, or origin will not disqualify them early in the hiring process. AI scores all candidates the same way based on the same questions.
Conclusion
Although AI has tremendous potential, the above challenges, such as knowing when and how bias can be introduced into data and algorithms, will require attention.
Despite these challenges, AI creators should not be burdened with new regulations that are shouldered hastily and stifle innovation.
Nevertheless, they advocate that it is important to work hard to minimize the risk of bias introducing itself into AI algorithms, either externally or internally. In cases where AI bias is suspected, you need frameworks for identifying whether it is present.
With tech changing all the time, it’s always important to stay up to date on the latest IoT trends and changes. Be sure to visit the main page of the blog often, as new content is being added all the time.
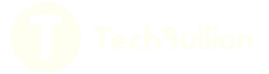