Introduction
The global banking industry is undergoing a profound transformation, driven by technological advancements, changing customer expectations, and regulatory developments. In this article, we will explore the current state of the banking industry worldwide, delve into the types of banking systems with regional specifics, examine the pivotal role of AI and machine learning in banking, highlight the diverse applications of AI in this sector, present five prime examples of AI in banking, and discuss the essential role of a machine learning development company in shaping the future of banking.
Modern Situation in the Banking Industry Worldwide
The modern banking landscape is characterized by several key trends:
- Digitalization: Banks worldwide are embracing digital channels to offer customers more accessible and convenient banking services.
- Fintech Integration: Collaboration with fintech startups and technology companies is becoming commonplace, driving innovation in banking.
- Regulatory Changes: Evolving regulatory frameworks, such as PSD2 in Europe, are reshaping the industry, emphasizing data security and open banking.
- Customer-Centric Approach: Banks are focusing on enhancing customer experiences, offering personalized services, and improving user interfaces.
Types of Banking Systems with Regional Specifics
Banking systems vary globally, with some key regional specifics:
- Commercial Banking: Predominant in the United States, commercial banks offer a wide range of services, from savings and loans to checking accounts.
- Universal Banking: Common in Europe, universal banks provide a comprehensive suite of financial services, including investment banking and insurance.
- Islamic Banking: Prevalent in the Middle East, Islamic banks adhere to Sharia principles, avoiding interest-based transactions and emphasizing risk-sharing.
- Centralized Banking: Found in some Asian countries, centralized banks play a significant role in the financial system, controlling credit and monetary policy.
AI in Banking
AI is revolutionizing the banking industry by automating processes, enhancing customer experiences, and improving risk management.
Machine Learning in Banking
Machine learning, a subset of AI, is instrumental in predictive analytics, fraud detection, and personalization in banking.
Types of Using AI in Banking
- Chatbots and Virtual Assistants: AI-powered chatbots provide 24/7 customer support, answering queries, and helping with routine tasks.
- Fraud Detection: Machine learning models analyze transaction data to identify and prevent fraudulent activities in real-time.
- Credit Scoring: AI algorithms assess creditworthiness more accurately, leading to fairer lending decisions.
- Personalized Financial Services: AI-driven systems offer tailored financial advice, investment recommendations, and product offerings based on individual profiles.
- Risk Management: Machine learning models analyze vast datasets to assess and manage risks more effectively, including credit risk and market risk.
Prime Examples of Using AI in Banking
- JPMorgan Chase’s COIN: JPMorgan employs COIN (Contract Intelligence), an AI system that reviews legal documents, saving thousands of hours in manual work.
- Wells Fargo’s Chatbots: Wells Fargo’s chatbots assist customers in finding information, conducting transactions, and resolving issues efficiently.
- Capital One’s Eno: Eno is an AI-driven virtual assistant that helps customers manage their accounts, track spending, and make payments.
- HSBC’s AI-Powered Anti-Money Laundering (AML): HSBC employs AI for AML compliance, automating the detection and reporting of suspicious transactions.
- Ally Bank’s Personalized Insights: Ally Bank uses AI to provide customers with personalized financial insights, offering tailored advice and recommendations.
Role of Machine Learning Development Companies in Banking
Machine learning development companies play a critical role in the banking industry by:
- Custom Model Development: Creating tailored machine learning models to address specific banking challenges.
- Data Analysis and Insights: Extracting valuable insights from vast financial datasets to inform decision-making.
- Model Optimization: Fine-tuning machine learning algorithms to improve performance, accuracy, and efficiency.
- Security and Compliance: Ensuring robust security measures and regulatory compliance in AI solutions.
- Scalability and Integration: Building scalable machine learning solutions that seamlessly integrate with existing banking systems.
Conclusion
The banking industry is at the forefront of technological innovation, with AI and machine learning playing a pivotal role in reshaping its future. As banks across the globe embrace digitalization and customer-centric approaches, the contributions of machine learning development companies in developing and implementing AI solutions will become increasingly essential. The future of banking promises a seamless fusion of human expertise and machine intelligence, offering customers more personalized, efficient, and secure financial services.
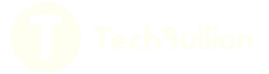